AI-Assisted Human Labeling: Batching for Efficiency without Overreliance.
Proc. ACM Hum. Comput. Interact.(2021)
摘要
Human labeling of training data is often a time-consuming, expensive part of machine learning. In this paper, we study "batch labeling", an AI-assisted UX paradigm, that aids data labelers by allowing a single labeling action to apply to multiple records. We ran a large scale study on Mechanical Turk with 156 participants to investigate labeler-AI-batching system interaction. We investigate the efficacy of the system when compared to a single-item labeling interface (i.e., labeling one record at-a-time), and evaluate the impact of batch labeling on accuracy and time. We further investigate the impact of AI algorithm quality and its effects on the labelers' overreliance, as well as potential mechanisms for mitigating it. Our work offers implications for the design of batch labeling systems and for work practices focusing on labeler-AI-batching system interaction.
更多查看译文
关键词
agents,ai,collaboration,data labeling
AI 理解论文
溯源树
样例
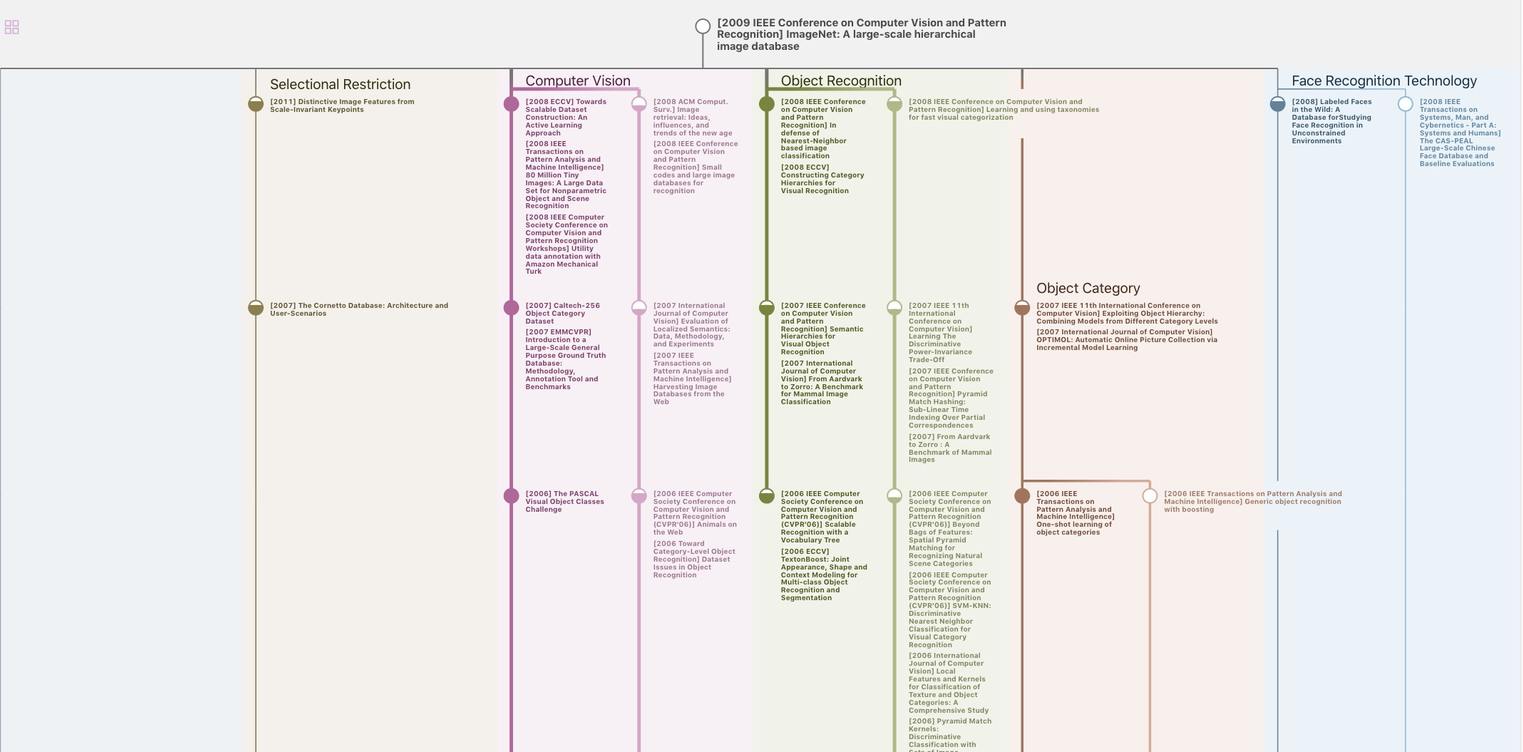
生成溯源树,研究论文发展脉络
Chat Paper
正在生成论文摘要