Interactive Monte Carlo denoising using affinity of neural features
ACM Transactions on Graphics(2021)
摘要
AbstractHigh-quality denoising of Monte Carlo low-sample renderings remains a critical challenge for practical interactive ray tracing. We present a new learning-based denoiser that achieves state-of-the-art quality and runs at interactive rates. Our model processes individual path-traced samples with a lightweight neural network to extract per-pixel feature vectors. The rest of our pipeline operates in pixel space. We define a novel pairwise affinity over the features in a pixel neighborhood, from which we assemble dilated spatial kernels to filter the noisy radiance. Our denoiser is temporally stable thanks to two mechanisms. First, we keep a running average of the noisy radiance and intermediate features, using a per-pixel recursive filter with learned weights. Second, we use a small temporal kernel based on the pairwise affinity between features of consecutive frames. Our experiments show our new affinities lead to higher quality outputs than techniques with comparable computational costs, and better high-frequency details than kernel-predicting approaches. Our model matches or outperfoms state-of-the-art offline denoisers in the low-sample count regime (2--8 samples per pixel), and runs at interactive frame rates at 1080p resolution.
更多查看译文
关键词
Monte Carlo denoising, deep learning
AI 理解论文
溯源树
样例
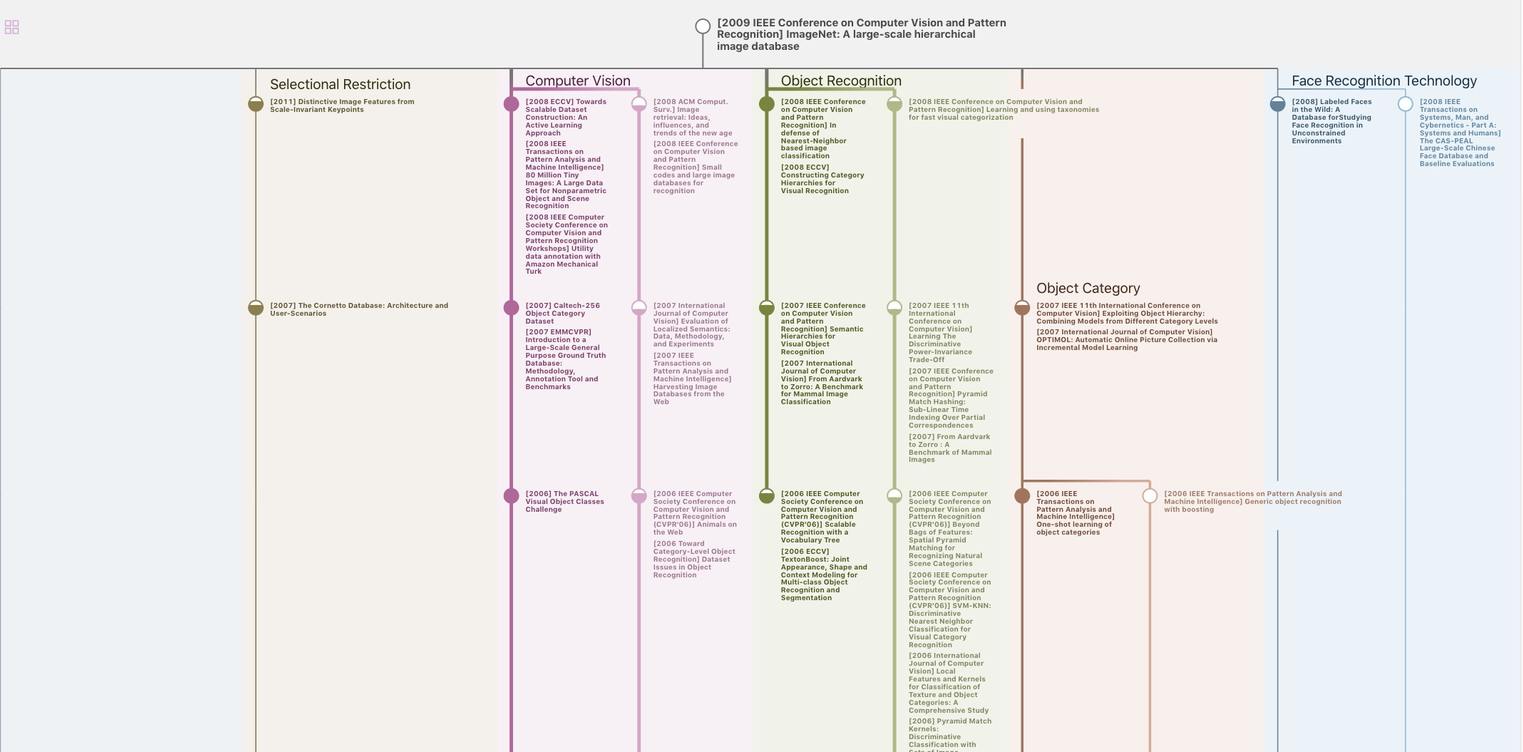
生成溯源树,研究论文发展脉络
Chat Paper
正在生成论文摘要