A 28nm 276.55TFLOPS/W Sparse Deep-Neural-Network Training Processor with Implicit Redundancy Speculation and Batch Normalization Reformulation
VLSI Circuits(2021)
摘要
A dynamic weight pruning (DWP) explored processor, named Trainer, is proposed for energy-efficient deep-neural-network (DNN) training on edge-device. It has three key features: 1) A implicit redundancy speculation unit (IRSU) improves 1.46× throughput. 2) A dataflow, allowing a reuse-adaptive dynamic compression and PE regrouping, increases 1.52× utilization. 3) A data-retrieval eliminated batch-normalization (BN) unit (REBU) saves 37.1% of energy. Trainer achieves a peak energy efficiency of 276.55TFLOPS/W. It reduces 2.23× training energy and offers a 1.76× training speedup compared with the state-of-the-art sparse DNN training processor.
更多查看译文
关键词
peak energy efficiency,batch normalization reformulation,energy-efficient deep-neural-network training,implicit redundancy speculation unit,reuse-adaptive dynamic compression,data-retrieval eliminated batch-normalization unit,sparse DNN training processor,dynamic weight pruning explored processor,PE regrouping,size 28.0 nm
AI 理解论文
溯源树
样例
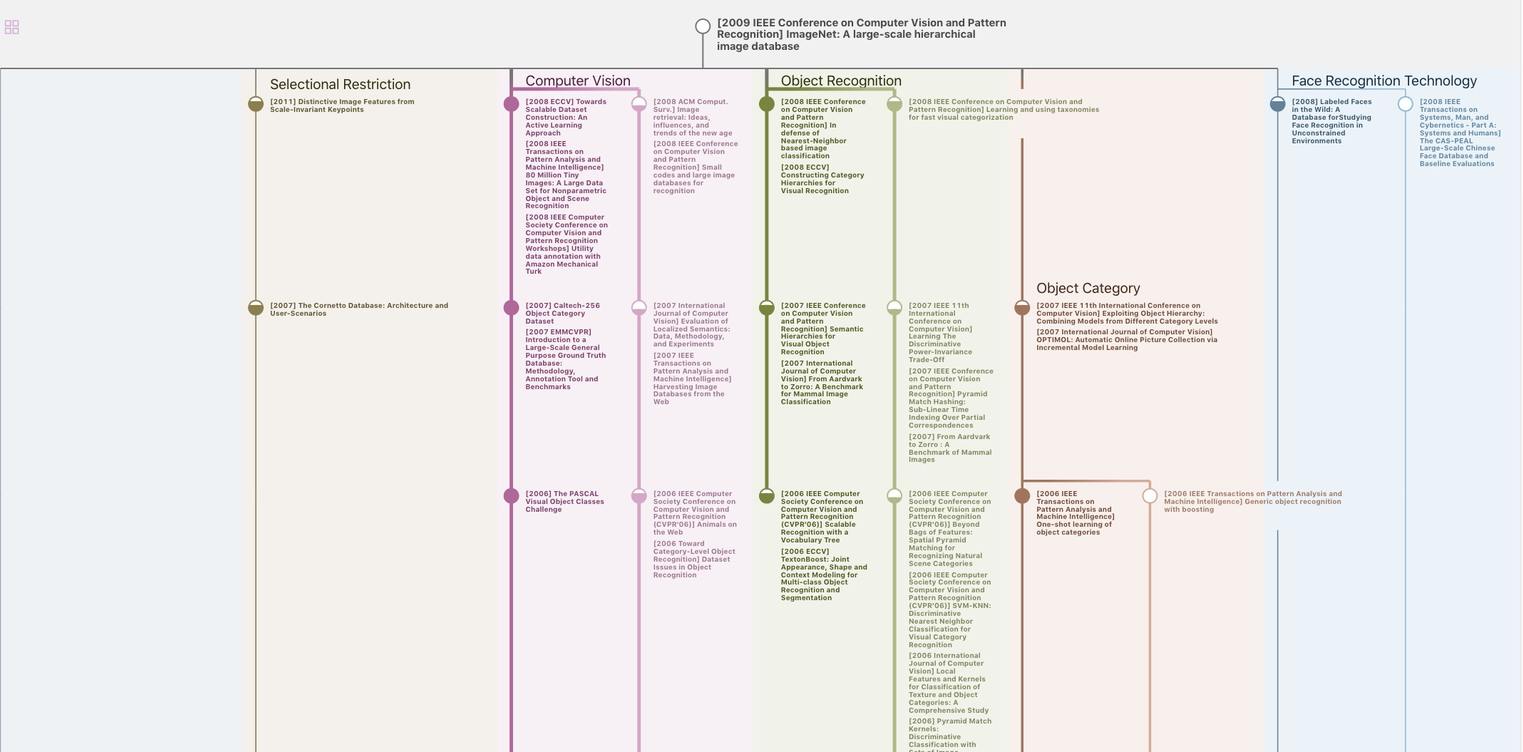
生成溯源树,研究论文发展脉络
Chat Paper
正在生成论文摘要