Controlled Text Generation as Continuous Optimization with Multiple Constraints.
Annual Conference on Neural Information Processing Systems(2021)
摘要
As large-scale language model pretraining pushes the state-of-the-art in text generation, recent work has turned to controlling attributes of the text such models generate. While modifying the pretrained models via fine-tuning remains the popular approach, it incurs a significant computational cost and can be infeasible due to a lack of appropriate data. As an alternative, we propose \textsc{MuCoCO}---a flexible and modular algorithm for controllable inference from pretrained models. We formulate the decoding process as an optimization problem that allows for multiple attributes we aim to control to be easily incorporated as differentiable constraints. By relaxing this discrete optimization to a continuous one, we make use of Lagrangian multipliers and gradient-descent-based techniques to generate the desired text. We evaluate our approach on controllable machine translation and style transfer with multiple sentence-level attributes and observe significant improvements over baselines.
更多查看译文
关键词
continuous optimization,text,constraints,generation
AI 理解论文
溯源树
样例
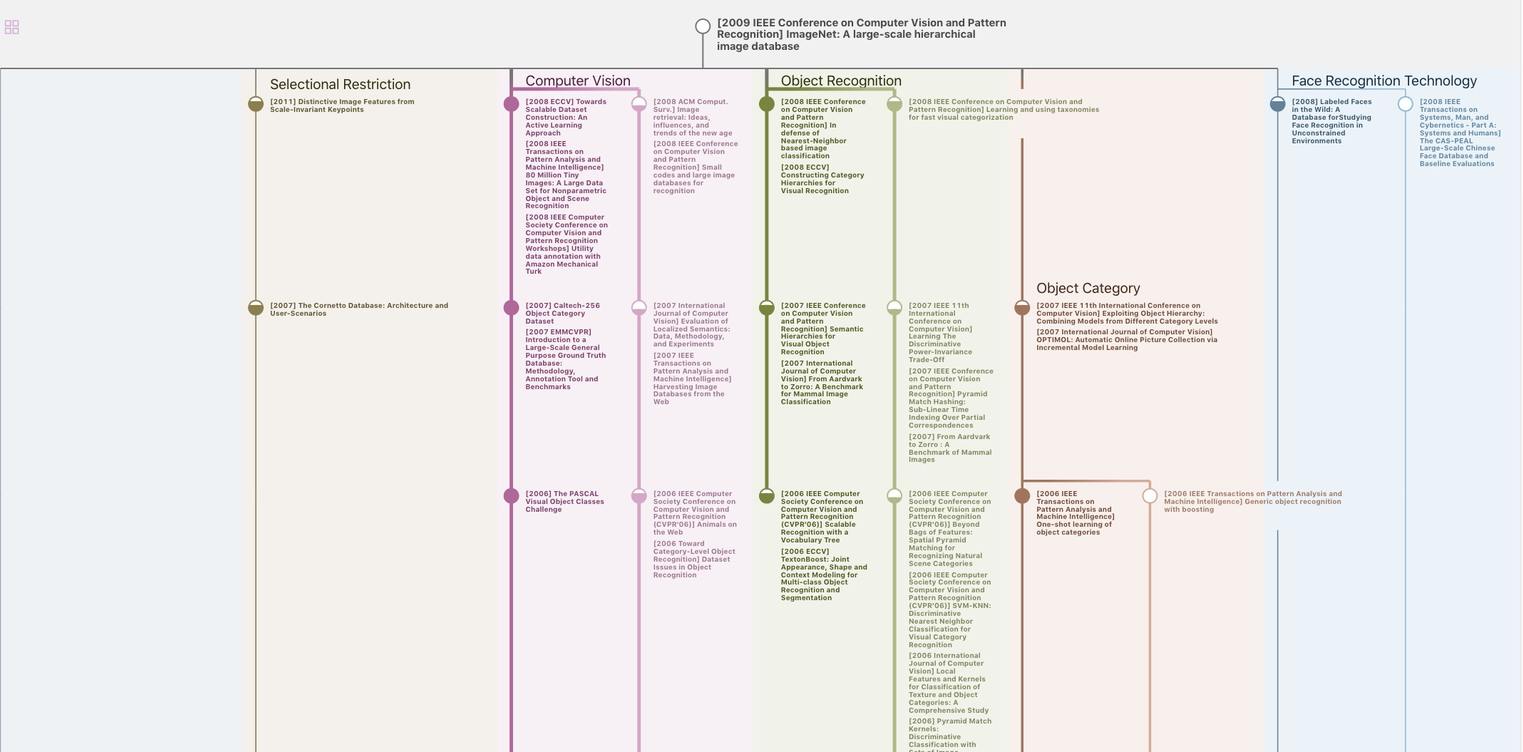
生成溯源树,研究论文发展脉络
Chat Paper
正在生成论文摘要