Byzantine-resilient distributed learning under constraints
2021 AMERICAN CONTROL CONFERENCE (ACC)(2021)
摘要
We consider a class of convex distributed statistical learning problems with inequality constraints in an adversarial scenario. At each iteration, an α-fraction of m machines, which are supposed to compute stochastic gradients of the loss function and send them to a master machine, may act adversarially and send faulty gradients. To guard against defective information sharing, we develop a Byzantine primal-dual algorithm. For α ∈ [0,0.5), we prove that after T iterations the algorithm achieves ~O(1/T+1/√{mT}-+α/√T) statistical error bounds on both the optimality gap and the constraint violation. Our result holds for a class of normed vector spaces and, when specialized to the Euclidean space, it attains the optimal error bound for Byzantine stochastic gradient descent.
更多查看译文
关键词
inequality constraints,convex distributed statistical learning problems,Byzantine-resilient,Byzantine stochastic gradient descent,optimal error,constraint violation,Byzantine primal-dual algorithm,defective information sharing,faulty gradients,master machine,loss function,stochastic gradients,α-fraction,iteration,adversarial scenario
AI 理解论文
溯源树
样例
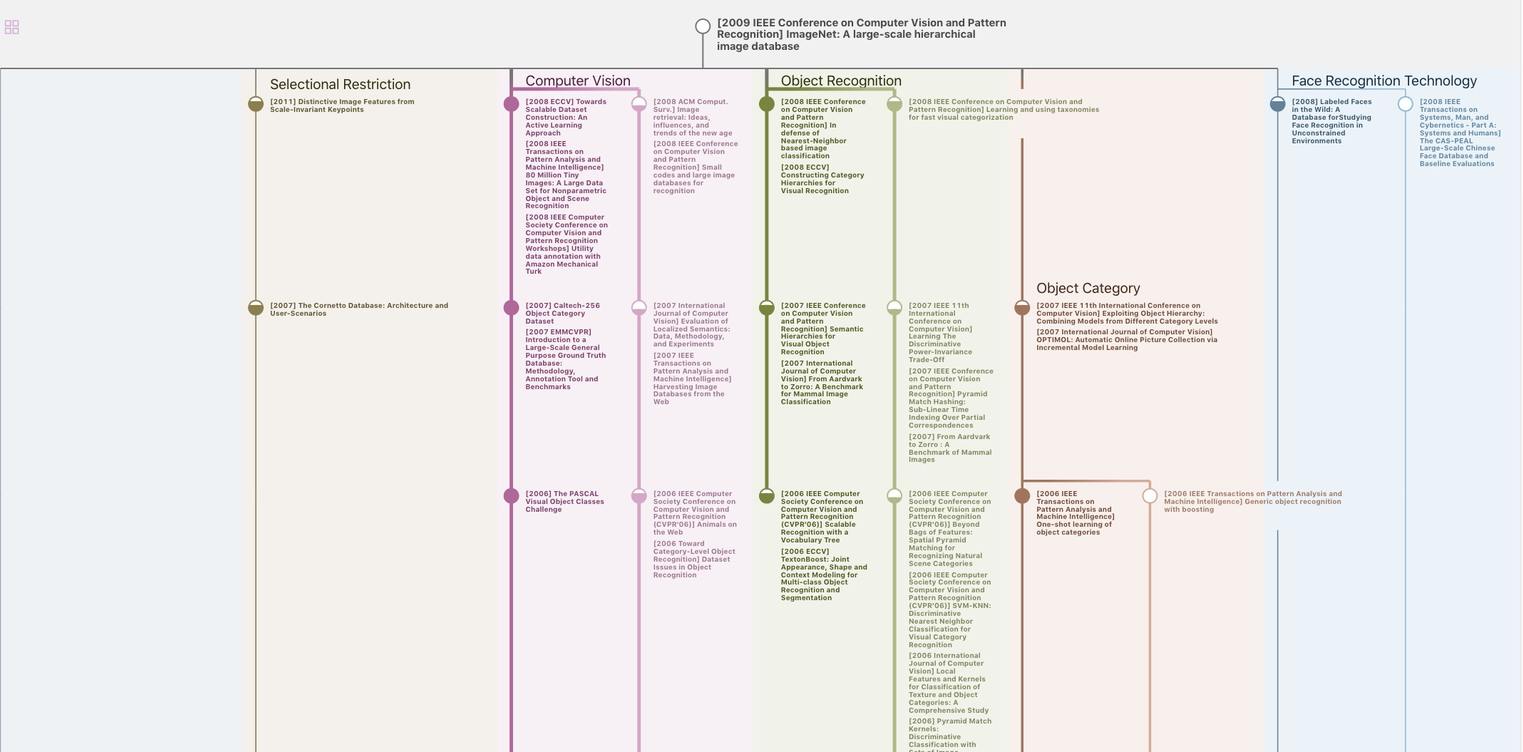
生成溯源树,研究论文发展脉络
Chat Paper
正在生成论文摘要