Low Size, Weight, and Power Neuromorphic Computing to Improve Combustion Engine Efficiency
2020 11th International Green and Sustainable Computing Workshops (IGSC)(2020)
摘要
Neuromorphic computing offers one path forward for AI at the edge. However, accessing and effectively utilizing a neuromorphic hardware platform is non-trivial. In this work, we present a complete pipeline for neuromorphic computing at the edge, including a small, inexpensive, low-power, FPGA-based neuromorphic hardware platform, a training algorithm for designing spiking neural networks for neuromorphic hardware, and a software framework for connecting those components. We demonstrate this pipeline on a real-world application, engine control for a spark-ignition internal combustion engine. We illustrate how we connect engine simulations with neuromorphic hardware simulations and training software to produce hardware-compatible spiking neural networks that perform engine control to improve fuel efficiency. We present initial results on the performance of these spiking neural networks and illustrate that they outperform open-loop engine control. We also give size, weight, and power estimates for a deployed solution of this type.
更多查看译文
关键词
neuromorphic,FPGA,engine control unit,internal combustion engine
AI 理解论文
溯源树
样例
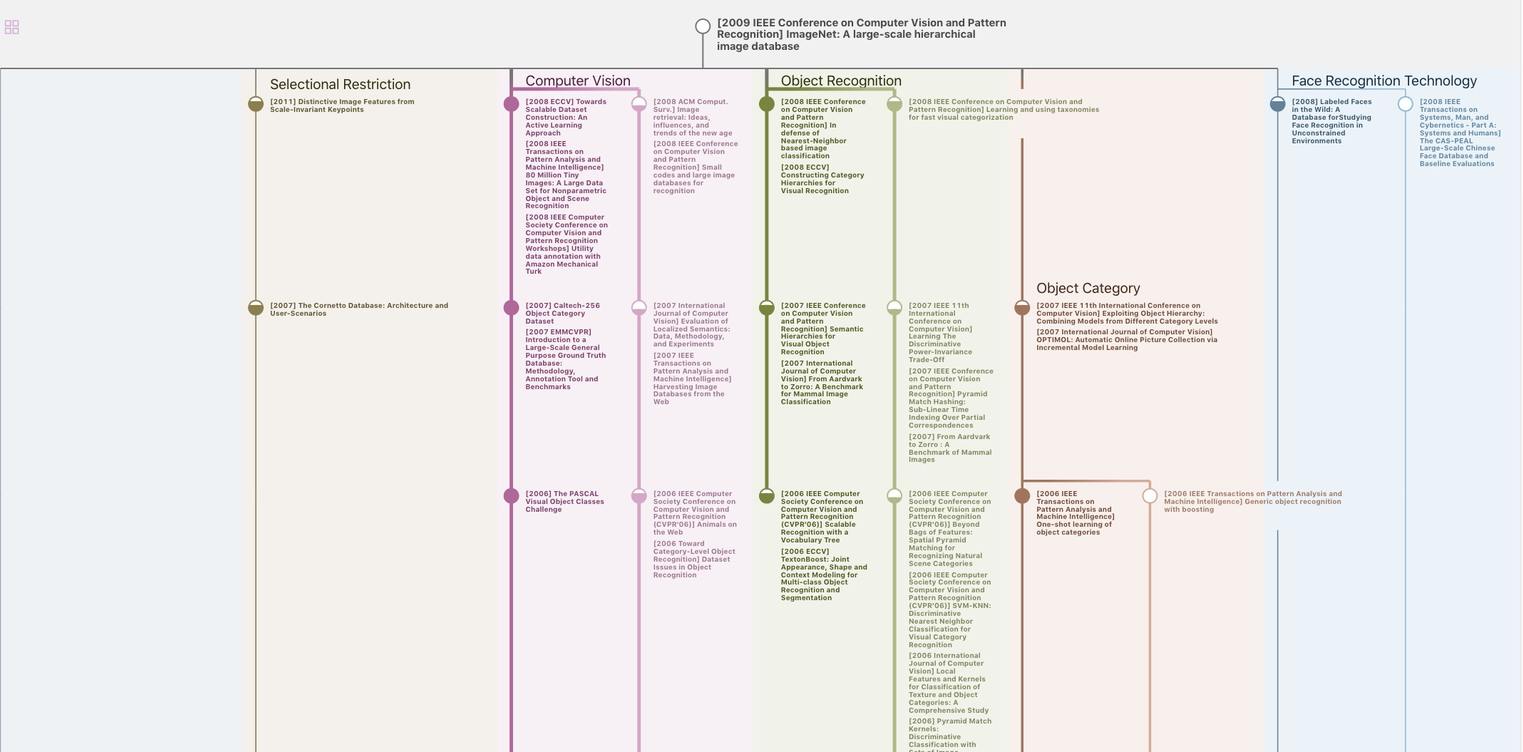
生成溯源树,研究论文发展脉络
Chat Paper
正在生成论文摘要