An Improved Live Anomaly Detection System (I-LADS) based on Deep Learning Algorithms
SECRYPT 2021: PROCEEDINGS OF THE 18TH INTERNATIONAL CONFERENCE ON SECURITY AND CRYPTOGRAPHY(2021)
摘要
Network Anomaly detection is an open issue that considers the problem of finding patterns in data that do not conform to expected behavior. Anomalies exhibit themselves in network statistics differently; therefore developing general models of normal network behavior and anomalies is a challenging task. This paper presents an Improved Live Anomaly Detection System (I-LADS) based on AutoEncoder (AE), a well known deep learning algorithm, to detect network traffic anomalies. I-LADS comes in two versions: (i) I-LADS-v1, that uses filters to independently model IP addresses from the NetFlow dataset, making it possible to train one model for each filtered IP address; and (ii) I-LADS-v2, that uses no filter and therefore a single algorithm is trained for all IP addresses. Experiments have been conducted using a valid dataset containing over two million connections to build a model with multiple features in order to identify the approach that most accurately detects traffic anomalies in the target network. Preliminary results show a promising solution with 99% and 94% of accuracy for the supervised and unsupervised learning approaches respectively.
更多查看译文
关键词
Deep Learning, Neural Network, Anomaly Detection, Network Traffic Behavior, AutoEncoder
AI 理解论文
溯源树
样例
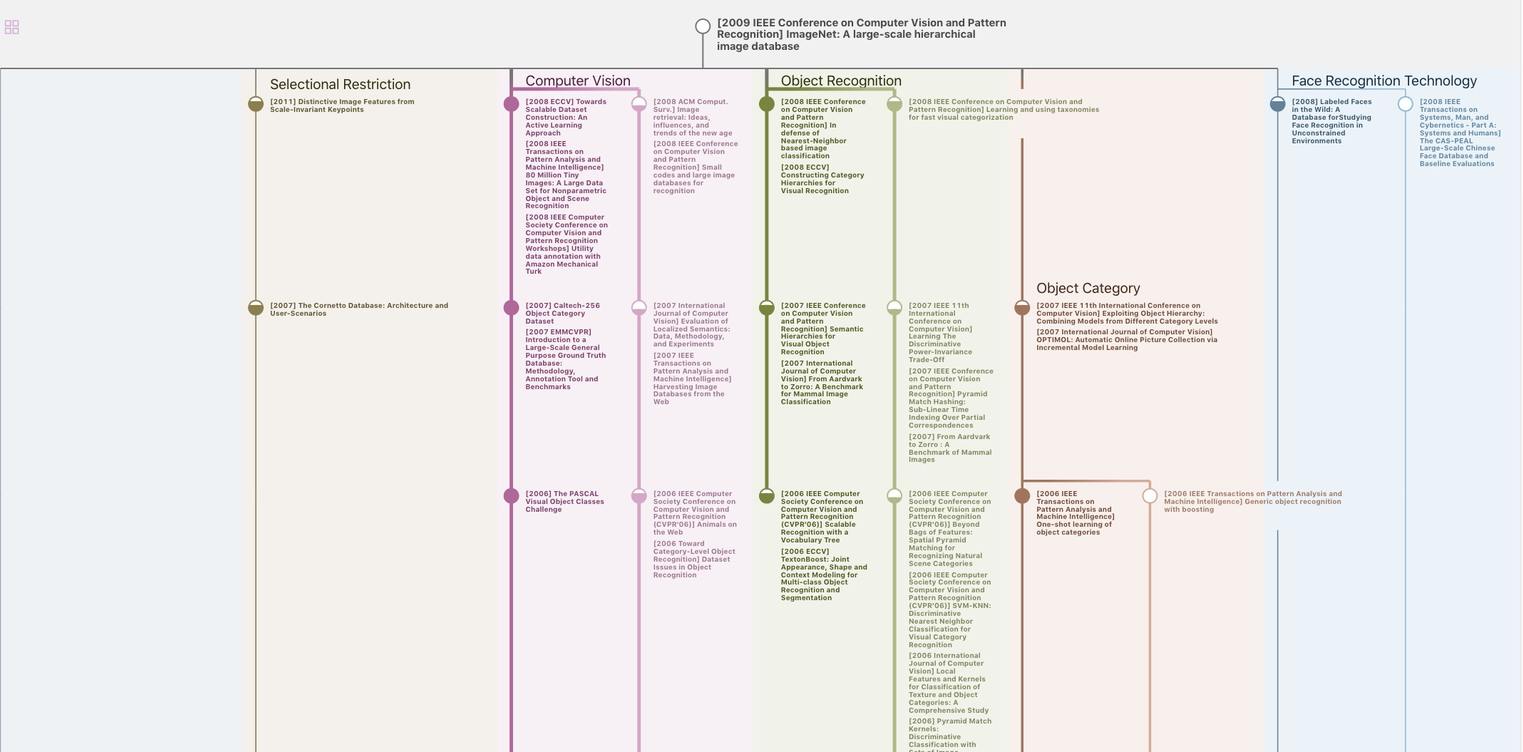
生成溯源树,研究论文发展脉络
Chat Paper
正在生成论文摘要