RigNet: Repetitive Image Guided Network for Depth Completion.
European Conference on Computer Vision(2022)
摘要
Depth completion deals with the problem of recovering dense depth maps from sparse ones, where color images are often used to facilitate this task. Recent approaches mainly focus on image guided learning frameworks to predict dense depth. However, blurry guidance in the image and unclear structure in the depth still impede the performance of the image guided frameworks. To tackle these problems, we explore a repetitive design in our image guided network to gradually and sufficiently recover depth values. Specifically, the repetition is embodied in both the image guidance branch and depth generation branch. In the former branch, we design a repetitive hourglass network to extract discriminative image features of complex environments, which can provide powerful contextual instruction for depth prediction. In the latter branch, we introduce a repetitive guidance module based on dynamic convolution, in which an efficient convolution factorization is proposed to simultaneously reduce its complexity and progressively model high-frequency structures. Extensive experiments show that our method achieves superior or competitive results on KITTI benchmark and NYUv2 dataset.
更多查看译文
关键词
Depth completion,Image guidance,Repetitive design
AI 理解论文
溯源树
样例
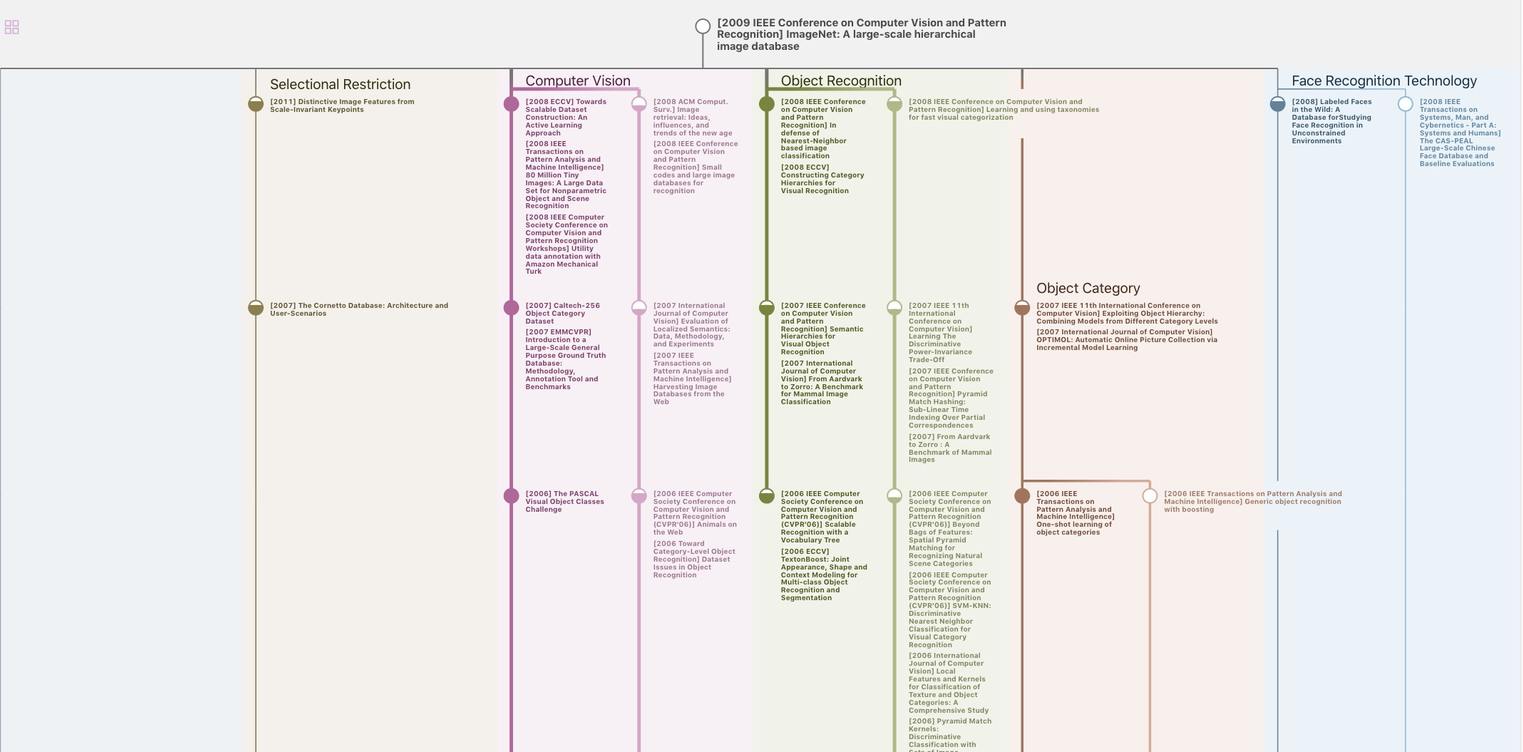
生成溯源树,研究论文发展脉络
Chat Paper
正在生成论文摘要