Learned Upper Bounds for the Time-Dependent Travelling Salesman Problem
IEEE Access(2023)
摘要
Fleet management plays a central role in several application contexts such as distribution planning, mail delivery, garbage collection, salt gritting, field service routing. Since road congestion has a big impact on driving times, fleet management can be enhanced by taking into account data on current traffic conditions. Today, most carriers gather high-quality historical traffic data by using global position system information. These data serve as an input for defining time-dependent travel times, i.e. travel times changing according to traffic conditions throughout the day. Given a fixed-size fleet of vehicles and a graph with arc traversal times varying over time, Time-Dependent Vehicle Routing Problems aim to select the best routes while minimizing the travelling costs. The basic version with only one route is usually referred to as the Time-Dependent Travelling Salesman Problem. The main goal of this work is to define tight upper bounds for this problem by reusing the information gained when solving instances with similar features. This is customary in distribution management, where vehicle routes have to be generated over and over again with similar input data. To this aim, the authors devise an upper bounding technique based on the solution of a classical (and simpler) time-independent Asymmetric Travelling Salesman Problem, where the constant arc costs are suitably defined by the combined use of a Linear Program and a mix of unsupervised and supervised Machine Learning techniques. The effectiveness of this approach has been assessed through a computational campaign on the real travel time functions of two European cities: Paris and London. The overall average gap between the proposed heuristic and the best-known solutions is about 0.001%. For 31 instances, new best solutions have been obtained.
更多查看译文
关键词
Routing,Heuristic algorithms,Approximation algorithms,Upper bound,Machine learning algorithms,Technological innovation,Urban areas,Traveling salesman problems,Ranking (statistics),Machine learning,path ranking invariance,time-dependent routing,travelling salesman problem
AI 理解论文
溯源树
样例
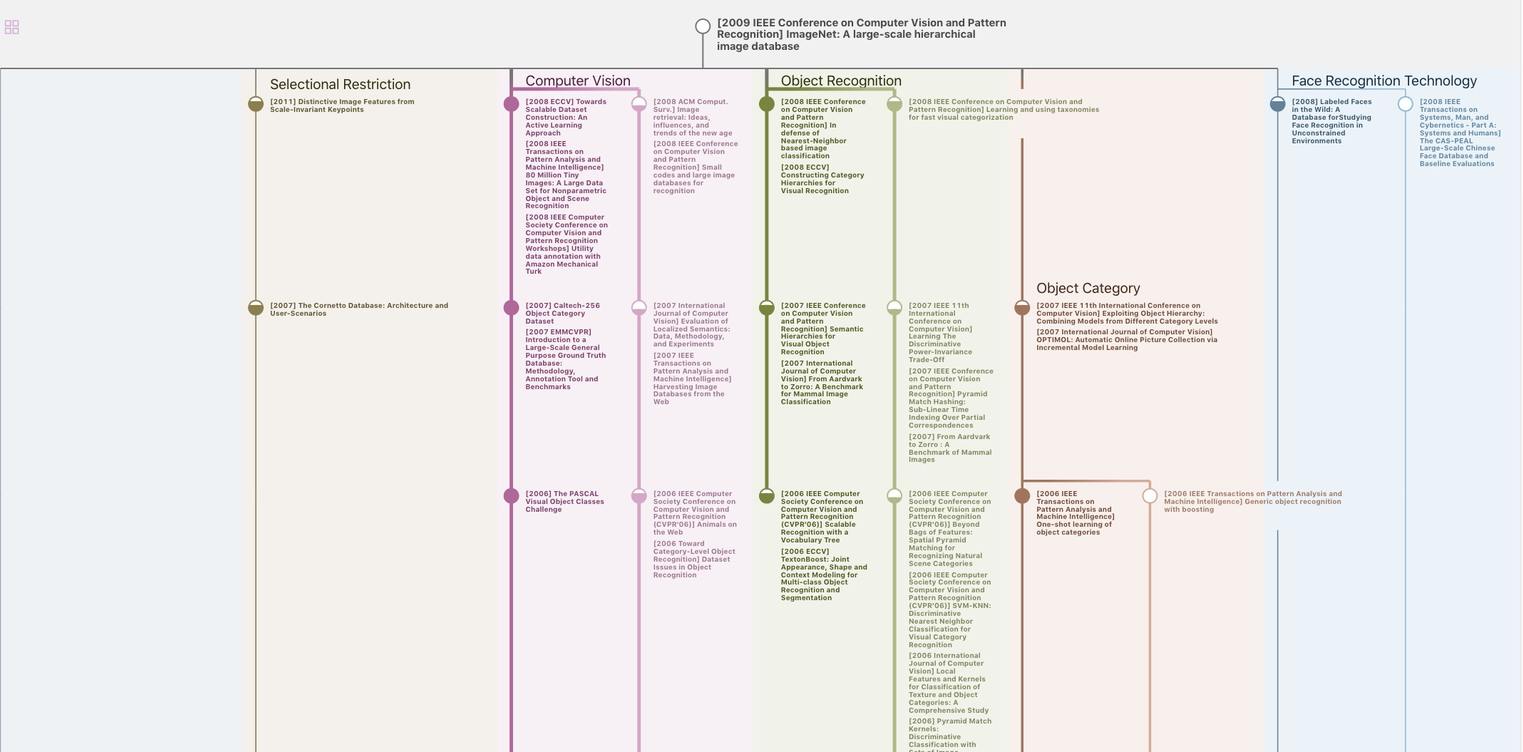
生成溯源树,研究论文发展脉络
Chat Paper
正在生成论文摘要