Kernel Path for ν-Support Vector Classification
IEEE Transactions on Neural Networks and Learning Systems(2023)
摘要
It is well known that the performance of a kernel method is highly dependent on the choice of kernel parameter. However, existing kernel path algorithms are limited to plain support vector machines (SVMs), which has one equality constraint. It is still an open question to provide a kernel path algorithm to
$\nu $
-support vector classification (
$\nu $
-SVC) with more than one equality constraint. Compared with plain SVM,
$\nu $
-SVC has the advantage of using a regularization parameter
$\nu $
for controlling the number of support vectors and margin errors. To address this problem, in this article, we propose a kernel path algorithm (KP
$\nu $
SVC) to trace the solutions of
$\nu $
-SVC exactly with respect to the kernel parameter. Specifically, we first provide an equivalent formulation of
$\nu $
-SVC with two equality constraints, which can avoid possible conflicts during tracing the solutions of
$\nu $
-SVC. Based on this equivalent formulation of
$\nu $
-SVC, we propose the KP
$\nu $
SVC algorithm to trace the solutions with respect to the kernel parameter. However, KP
$\nu $
SVC traces nonlinear solutions of kernel method rather than the errors of loss function, and it is still a challenge to provide the algorithm that guarantees to find the global optimal model. To address this challenging problem, we extend the classical error path algorithm to the nonlinear kernel solution paths and propose a new kernel error path (KEP) algorithm that ensures to find the global optimal kernel parameter by minimizing the cross validation error. We also provide the finite convergence analysis and computational complexity analysis to KP
$\nu $
SVC and KEP. Extensive experimental results on a variety of benchmark datasets not only verify the effectiveness of KP
$\nu $
SVC but also show the advantage of applying KEP to select the optimal kernel parameter.
更多查看译文
关键词
Kernel path,model selection,piecewise non-linear,support vector machine (SVM)
AI 理解论文
溯源树
样例
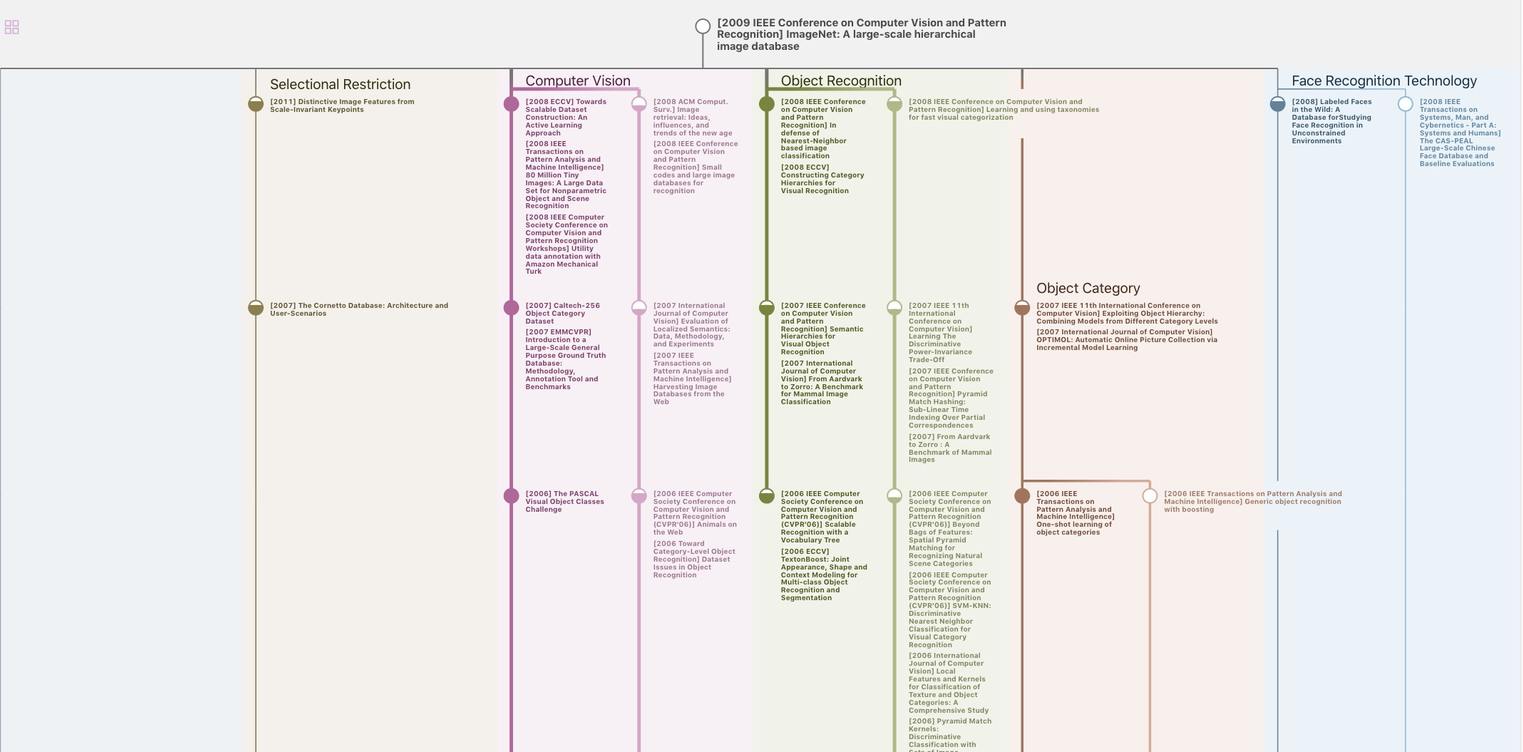
生成溯源树,研究论文发展脉络
Chat Paper
正在生成论文摘要