Improvements to Deep-Learning-based Feasibility Prediction of Switched Ethernet Network Configurations.
RTNS(2021)
摘要
Graph neural network (GNN) is an advanced machine learning model, which has been recently applied to encode Ethernet configurations as graphs and predict their feasibility in terms of meeting deadlines constraints. Ensembles of GNN models have proven to be robust to changes in the topology and traffic patterns with respect to the training set. However, the moderate prediction accuracy of the model, 79.3% at the lowest, hinders the application of GNN to real-world problems. This study proposes improvements to the base GNN model in the construction of the training set and the structure of the model itself. We first introduce new training sets that are more diverse in terms of topologies and traffic patterns and focus on configurations that are difficult to predict. We then enhance the GNN model with more powerful activation functions, multiple channels and implement a technique called global pooling. The prediction accuracy of ensemble of GNNs with a combination of the suggested improvements increases significantly, up to 11.9% on the same 13 testing sets. Importantly, these improvements increase only marginally the time it takes to predict unseen configurations, i.e., the speedup factor is still from 50 to 1125 compared to schedulability analysis, which allows a far more extensive exploration of the design space.
更多查看译文
关键词
Machine learning, Graph Neural Network, Schedulability analysis, Design Space Exploration, Time-Sensitive Networking
AI 理解论文
溯源树
样例
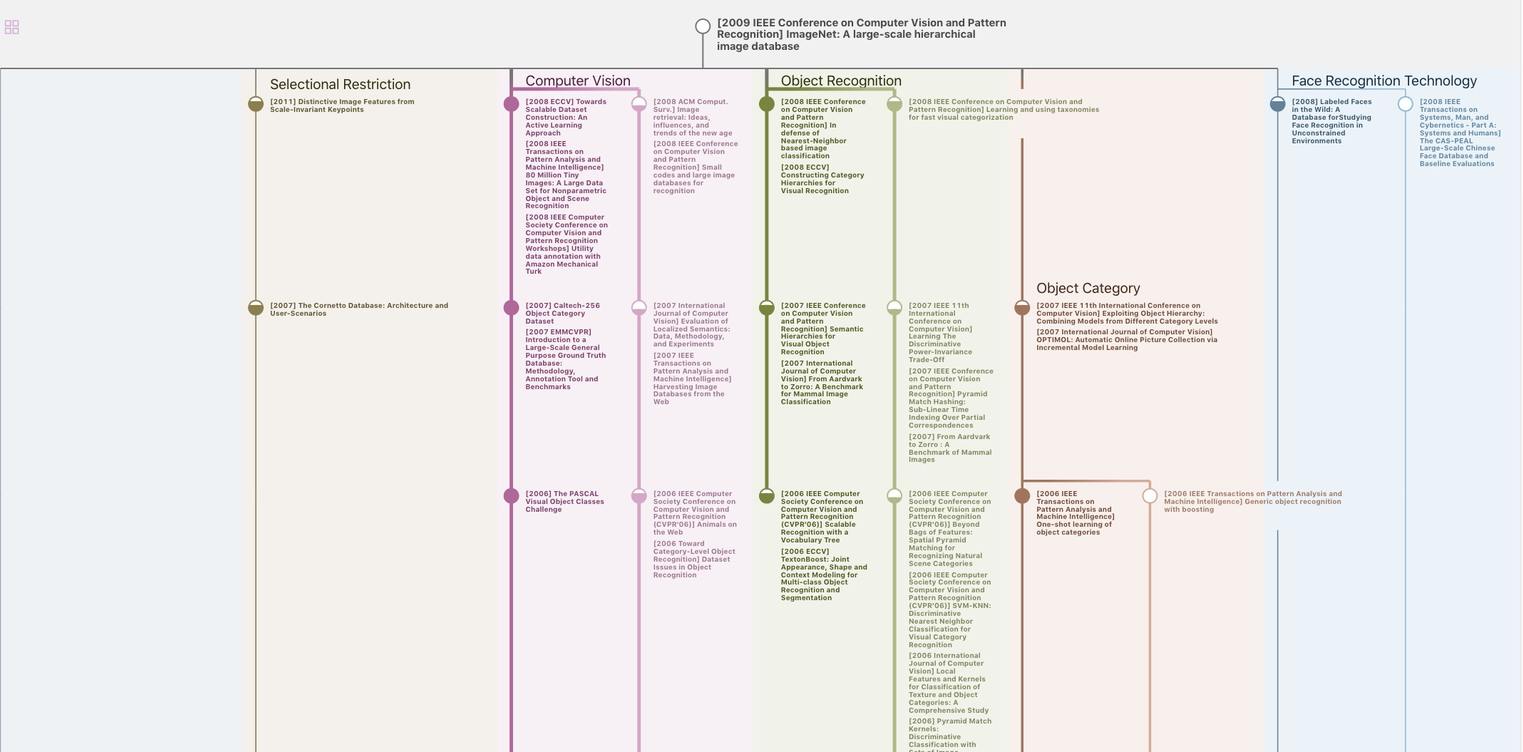
生成溯源树,研究论文发展脉络
Chat Paper
正在生成论文摘要