Regularising Inverse Problems with Generative Machine Learning Models
Journal of Mathematical Imaging and Vision(2024)
摘要
Deep neural network approaches to inverse imaging problems have produced impressive results in the last few years. In this survey paper, we consider the use of generative models in a variational regularisation approach to inverse problems. The considered regularisers penalise images that are far from the range of a generative model that has learned to produce images similar to a training dataset. We name this family generative regularisers . The success of generative regularisers depends on the quality of the generative model and so we propose a set of desired criteria to assess generative models and guide future research. In our numerical experiments, we evaluate three common generative models, autoencoders, variational autoencoders and generative adversarial networks, against our desired criteria. We also test three different generative regularisers on the inverse problems of deblurring, deconvolution, and tomography. We show that restricting solutions of the inverse problem to lie exactly in the range of a generative model can give good results but that allowing small deviations from the range of the generator produces more consistent results. Finally, we discuss future directions and open problems in the field.
更多查看译文
关键词
Inverse problems,Generative models,Machine learning,Imaging
AI 理解论文
溯源树
样例
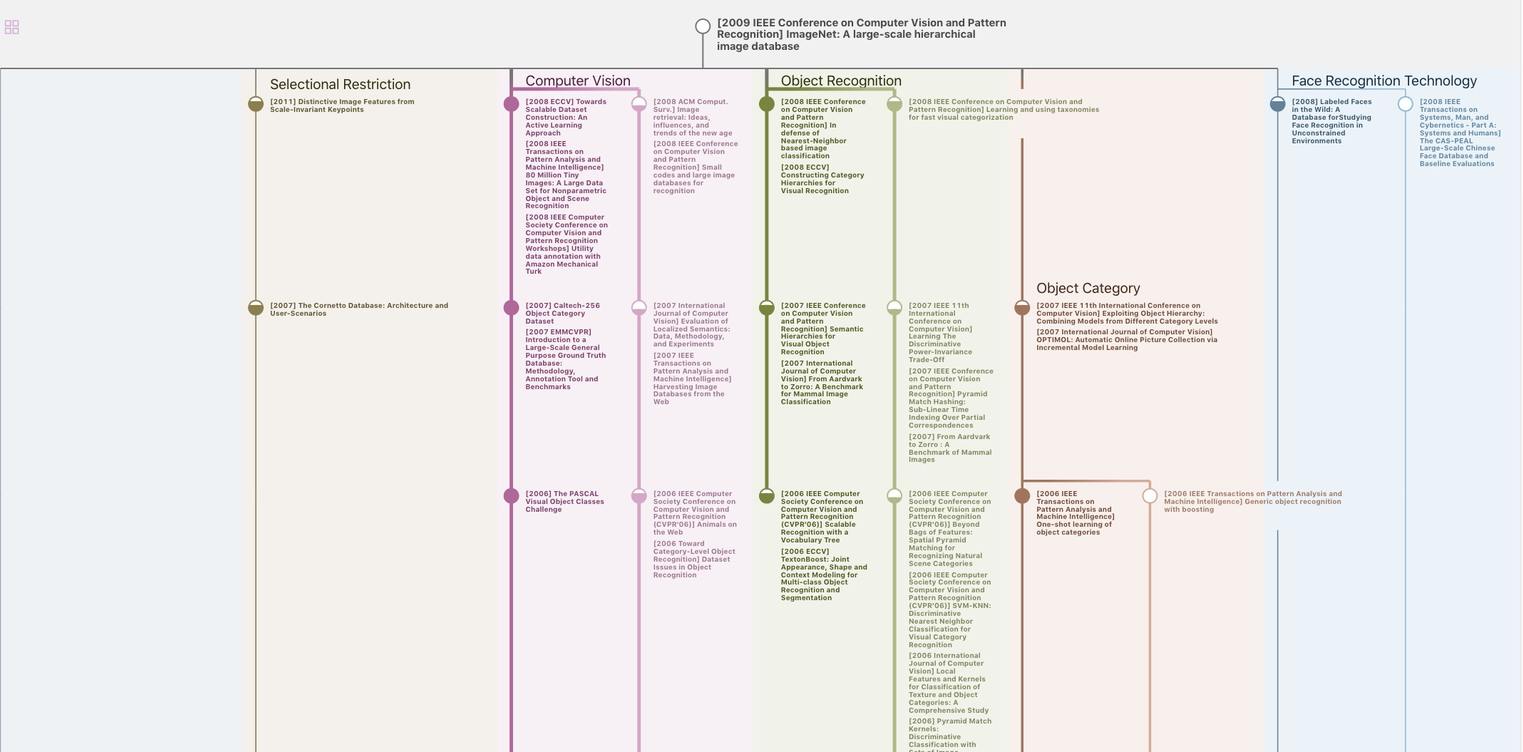
生成溯源树,研究论文发展脉络
Chat Paper
正在生成论文摘要