Vibra: neural adaptive streaming of VBR-encoded videos
ACM Conferences(2021)
摘要
ABSTRACTVariable Bitrate (VBR) video encoding can provide much high quality-to-bits ratio compared to the widely adopted Constant Bitrate (CBR) encoding, and thus receives significant attentions by content providers in recent years. However, it is challenging to design efficient adaptive bitrate algorithms for VBR-encoded videos due to the sharply fluctuating chunk size and the resulting bitrate burstiness. In this paper, we propose a neural adaptive streaming framework called Vibra for VBR-encoded videos, which can well accommodate the high fluctuation of video chunk sizes and improve the quality-of-experience (QoE) of end users significantly. Our framework takes the characteristics of VBR-encoded videos into account, and adopts the technique of deep reinforcement learning to train a model for bitrate adaptation. We also conduct extensive trace-driven experiments, and the results show that Vibra outperforms the state-of-the-art ABR algorithms with an improvement of 8.17% -- 29.21% in terms of the average QoE.
更多查看译文
关键词
VBR-encoded Video, Video Streaming, Adaptive Bitrate, Reinforcement Learning
AI 理解论文
溯源树
样例
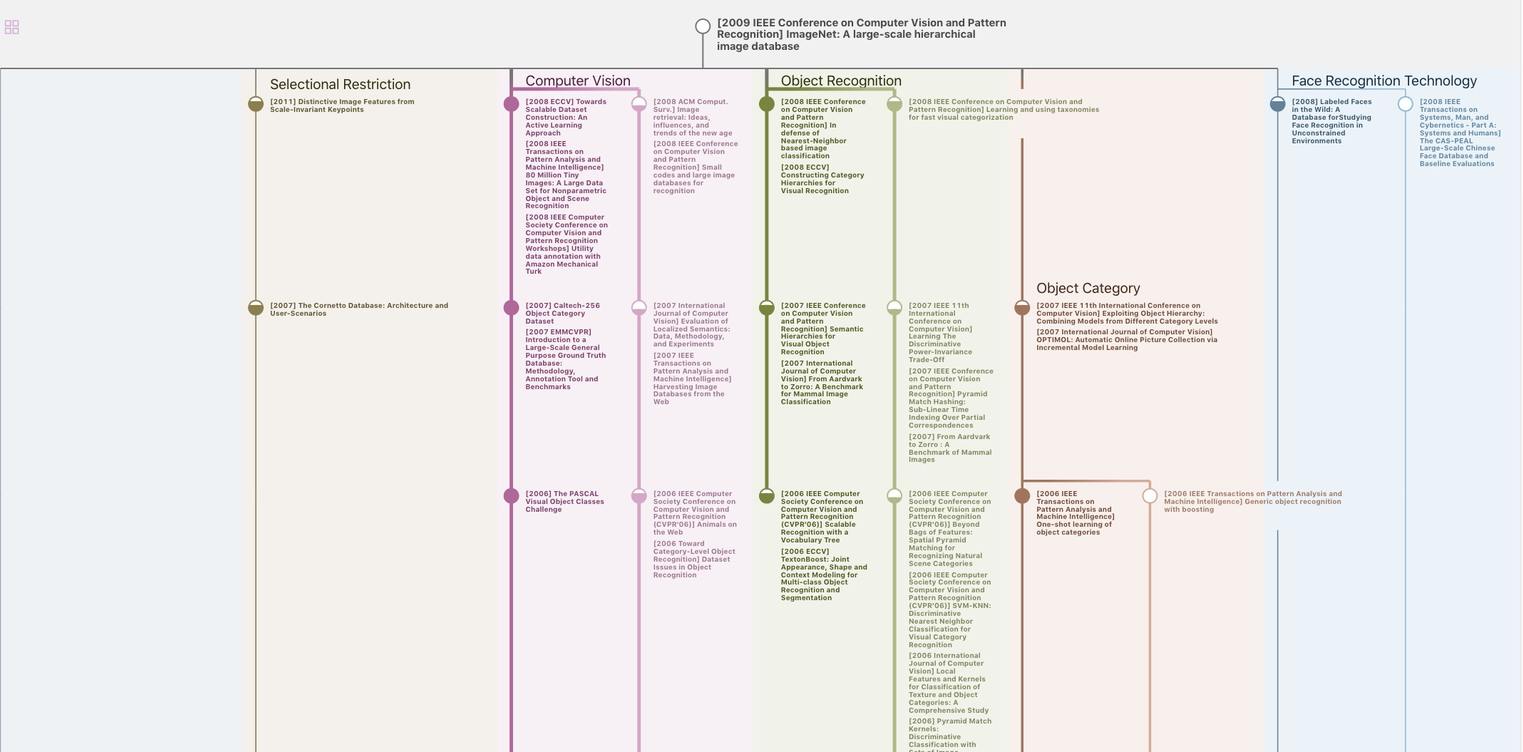
生成溯源树,研究论文发展脉络
Chat Paper
正在生成论文摘要