Positively Weighted Kernel Quadrature via Subsampling
NeurIPS 2022(2022)
摘要
We study kernel quadrature rules with convex weights. Our approach combines the spectral properties of the kernel with recombination results about point measures. This results in effective algorithms that construct convex quadrature rules using only access to i.i.d. samples from the underlying measure and evaluation of the kernel and that result in a small worst-case error. In addition to our theoretical results and the benefits resulting from convex weights, our experiments indicate that this construction can compete with the optimal bounds in well-known examples.
更多查看译文
关键词
kernel quadrature,recombination,reproducing kernel Hilbert space,Nyström approximation
AI 理解论文
溯源树
样例
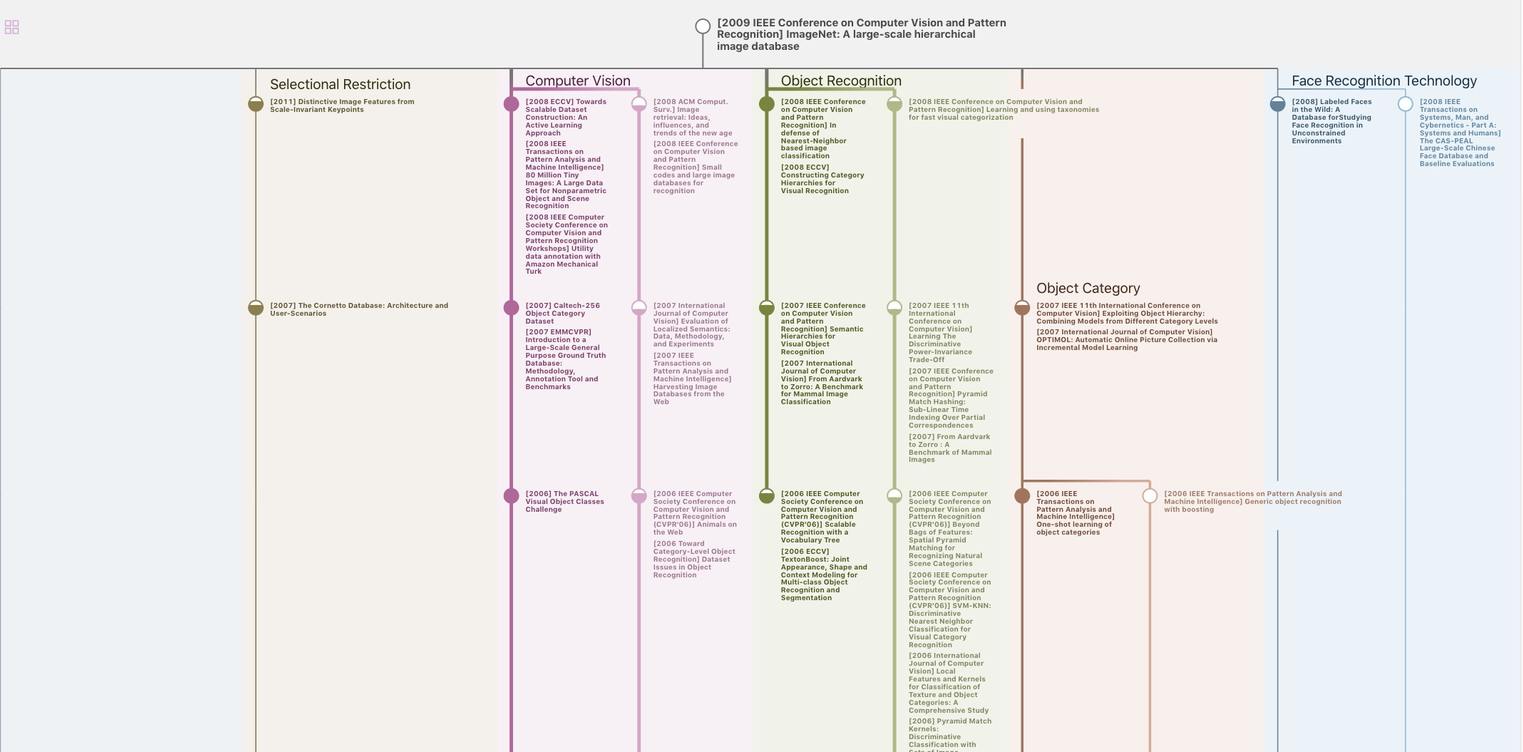
生成溯源树,研究论文发展脉络
Chat Paper
正在生成论文摘要