A Convex Optimization Framework For The Inverse Problem Of Identifying A Random Parameter In A Stochastic Partial Differential Equation
SIAM-ASA JOURNAL ON UNCERTAINTY QUANTIFICATION(2021)
摘要
The primary objective of this work is to study the inverse problem of identifying a stochastic parameter in partial differential equations with random data. In the framework of stochastic Sobolev spaces, we prove the Lipschitz continuity and the differentiability of the parameter-to-solution map and provide a new derivative characterization. We introduce a new energy-norm based modified output least-squares (OLS) objective functional and prove its smoothness and convexity. For stable inversion, we develop a regularization framework and prove an existence result for the regularized stochastic optimization problem. We also consider the OLS based stochastic optimization problem and provide an adjoint approach to compute the derivative of the OLS-functional. In the finite-dimensional noise setting, we give a parameterization of the inverse problem. We develop a computational framework by using the stochastic Galerkin discretization scheme and derive explicit discrete formulas for the considered objective functionals and their gradient. We provide detailed computational results to illustrate the feasibility and efficacy of the developed inversion framework. Encouraging numerical results demonstrate some of the advantages of the new framework over the existing approaches.
更多查看译文
关键词
stochastic parameter identification, stochastic inverse problem, partial differential equations with random data, stochastic Galerkin method, regularization, infinite-dimensional noise
AI 理解论文
溯源树
样例
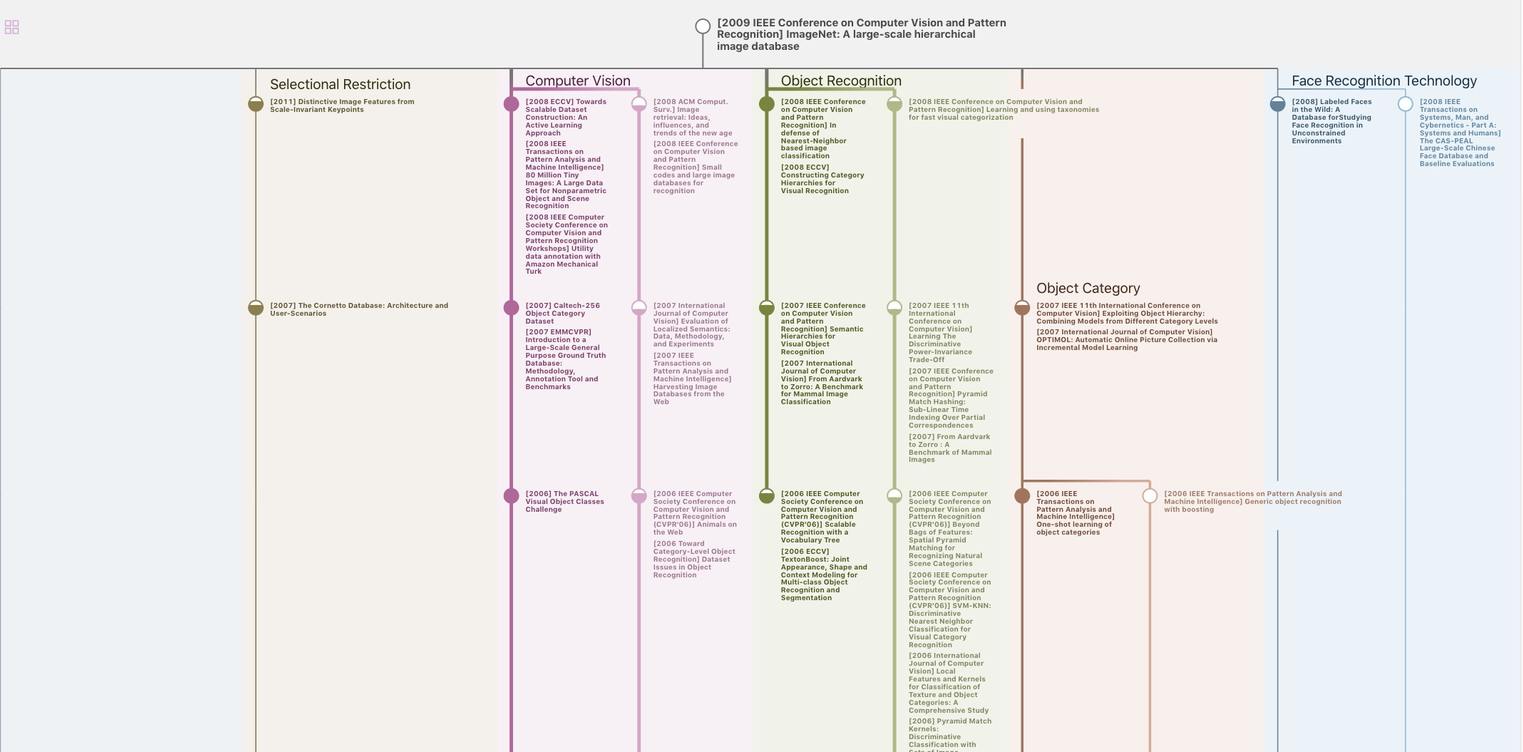
生成溯源树,研究论文发展脉络
Chat Paper
正在生成论文摘要