EGC2: Enhanced Graph Classification with Easy Graph Compression
arxiv(2023)
摘要
Graph classification is crucial in network analyses. Networks face potential security threats, such as adversarial attacks. Some defense methods may trade off the algorithm complexity for robustness, such as adversarial training, whereas others may trade off clean example performance, such as smoothingbased defense. Most suffer from high complexity or low transferability. To address this problem, we proposed EGC2, an enhanced graph classification model with easy graph compression. EGC2 captures the relationship between the features of different nodes by constructing feature graphs and improving the aggregation of the node-level representations. To achieve lower-complexity defense applied to graph classification models, EGC2 utilizes a centrality-based edge-importance index to compress the graphs, filtering out trivial structures and adversarial perturbations in the input graphs, thus improving the model's robustness. Experiments on ten benchmark datasets demonstrate that the proposed feature read-out and graph compression mechanisms enhance the robustness of multiple basic models, resulting in a state-of-the-art performance in terms of accuracy and robustness against various adversarial attacks.
更多查看译文
关键词
Graph classification,Adversarial attack,Graph compression,Feature aggregation,Robustness
AI 理解论文
溯源树
样例
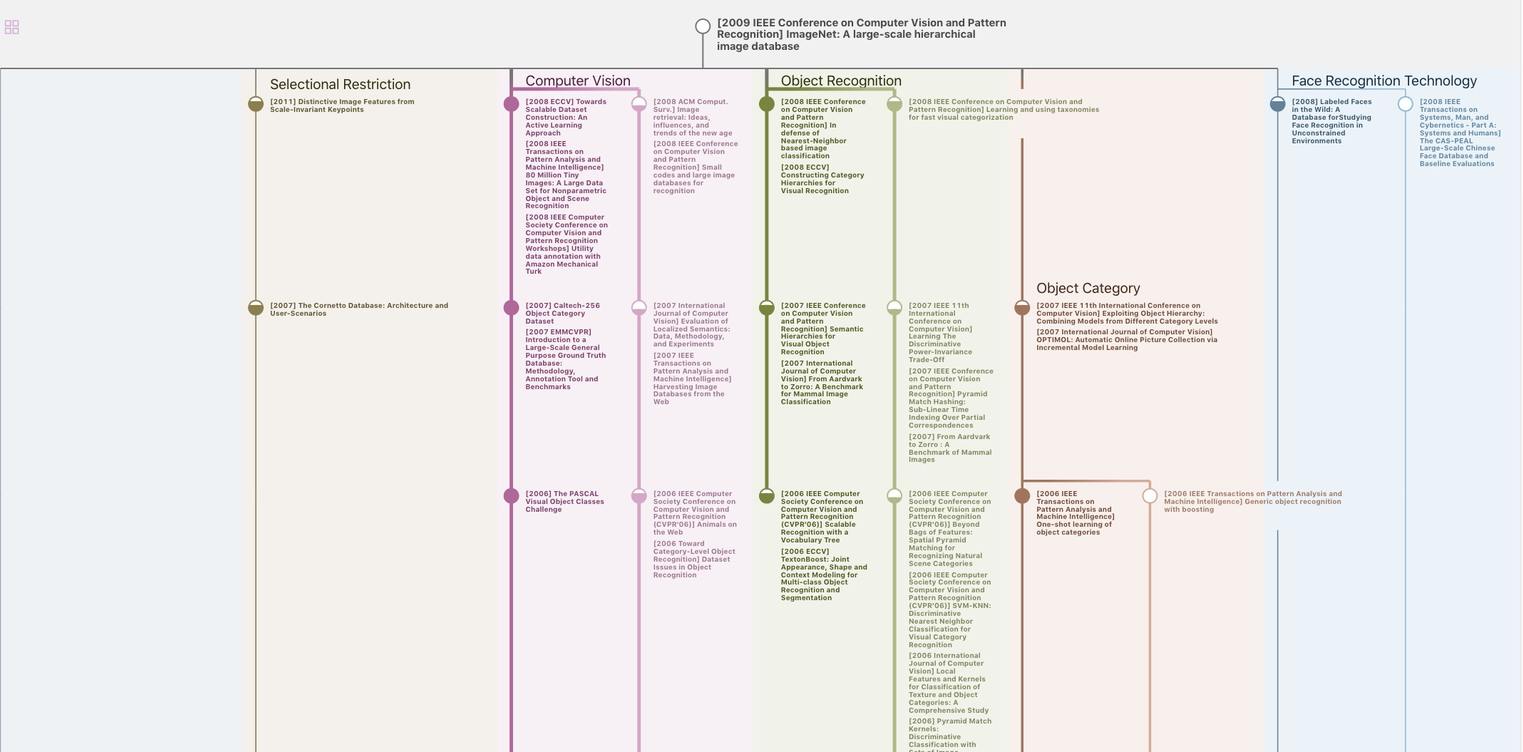
生成溯源树,研究论文发展脉络
Chat Paper
正在生成论文摘要