Automated Paraphrase Quality Assessment Using Recurrent Neural Networks and Language Models
INTELLIGENT TUTORING SYSTEMS (ITS 2021)(2021)
摘要
The ability to automatically assess the quality of paraphrases can be very useful for facilitating literacy skills and providing timely feedback to learners. Our aim is twofold: a) to automatically evaluate the quality of paraphrases across four dimensions: lexical similarity, syntactic similarity, semantic similarity and paraphrase quality, and b) to assess how well models trained for this task generalize. The task is modeled as a classification problem and three different methods are explored: a) manual feature extraction combined with an Extra Trees model, b) GloVe embeddings and a Siamese neural network, and c) using a pretrained BERT model fine-tuned on our task. Starting from a dataset of 1998 paraphrases from the User Language Paraphrase Corpus (ULPC), we explore how the three models trained on the ULPC dataset generalize when applied on a separate, small paraphrase corpus based on children inputs. The best out-of-the-box generalization performance is obtained by the Extra Trees model with at least 75% average F1-scores for the three similarity dimensions. We also show that the Siamese neural network and BERT models can obtain an improvement of at least 5% after fine-tuning across all dimensions.
更多查看译文
关键词
Paraphrase quality assessment, Natural language processing, Recurrent neural networks, Language models
AI 理解论文
溯源树
样例
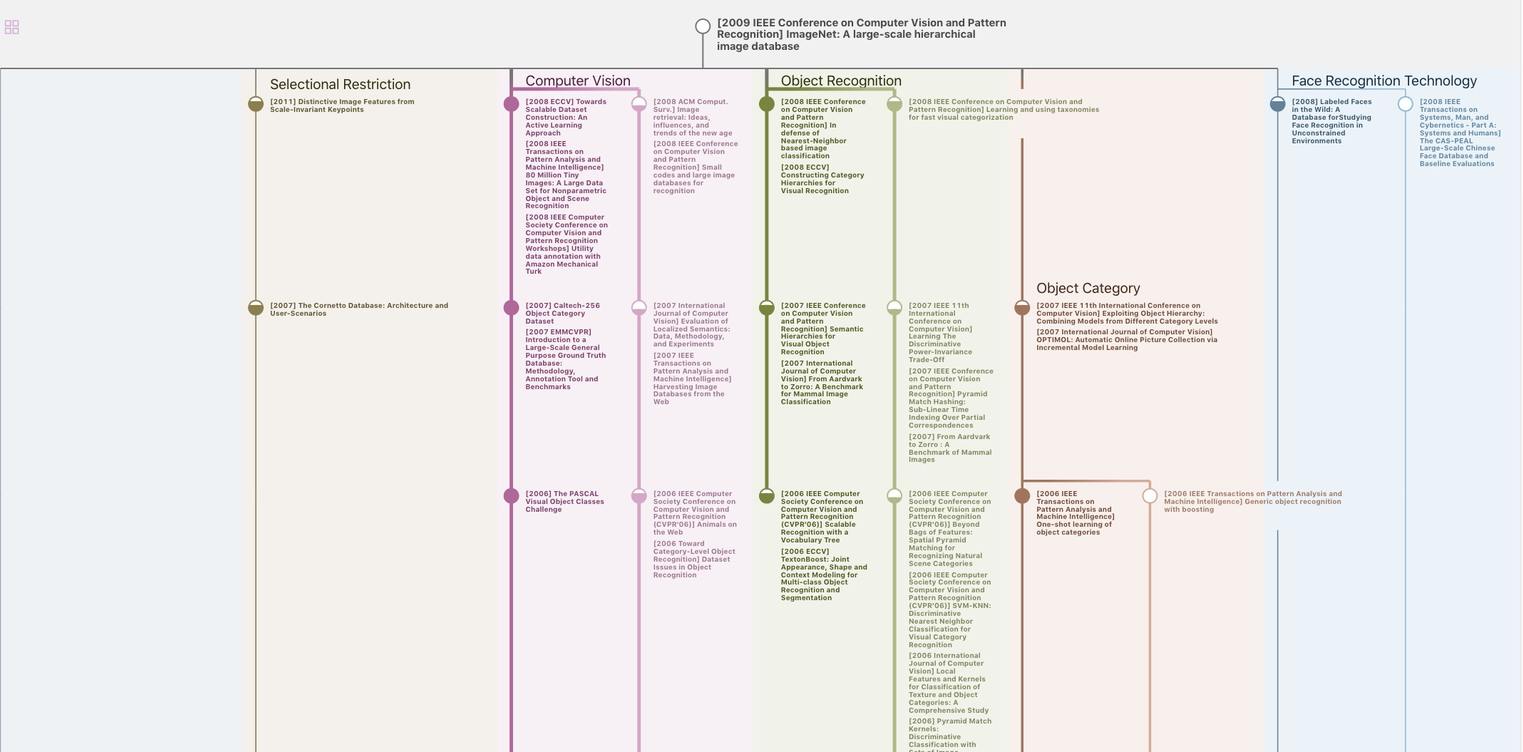
生成溯源树,研究论文发展脉络
Chat Paper
正在生成论文摘要