Distributed Multi-Agent Learning for Service Function Chain Partial Offloading at the Edge.
ICC Workshops(2021)
摘要
Multi-Access Edge Computing (MEC) along with "learning at the edge" brings unique opportunities for enhancing the utilization of resources in the next generation wireless networks. Using Network Function Virtualization (NFV), Service Function Chains (SFCs), a set of ordered virtual network functions (VNFs), can be deployed within the MEC infrastructure. The user equipment (UEs) can offload VNFs with intense computational load to the MEC servers with rich storage and computation resources. In this paper, we address the problem of partial offloading of a chain of services where each VNF of the SFC request can be either performed locally or offloaded onto a MEC server. The objective is to concurrently minimize the long-term cost of the UEs which is given in terms of both delay and energy consumption. This problem is highly complex and calls for distributed multi-agent learning techniques. We formulate the problem as a distributed multi-agent reinforcement learning problem and use double deep Q-network (DDQN) algorithm to solve it. Our simulation results show that the proposed DDQN-based solution has comparable results to an exhaustive search algorithm.
更多查看译文
关键词
Network function virtualization,mobile edge computing,multi-agent edge learning,double deep Q-network
AI 理解论文
溯源树
样例
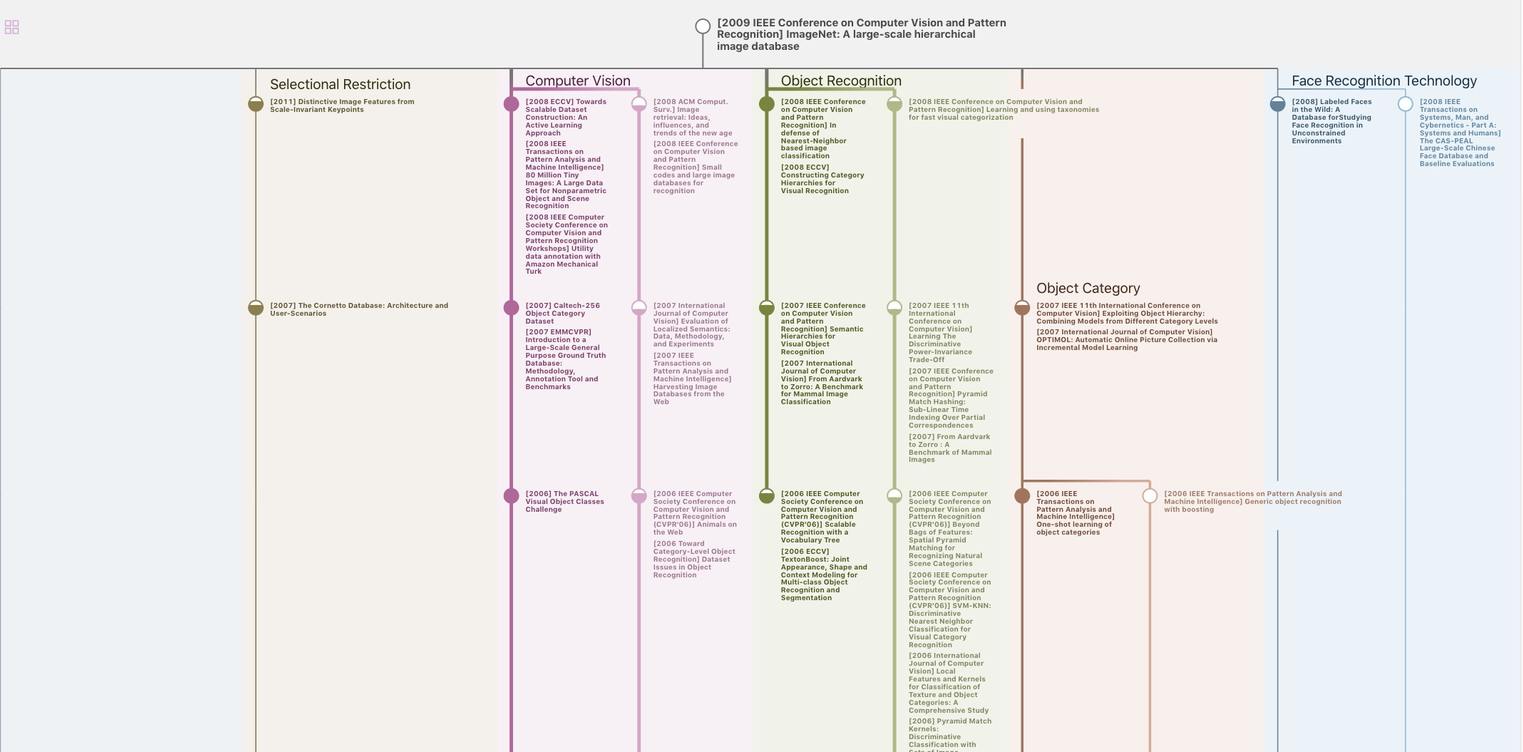
生成溯源树,研究论文发展脉络
Chat Paper
正在生成论文摘要