Nomen est Omen - The Role of Signatures in Ascribing Email Author Identity with Transformer Neural Networks
2021 IEEE Security and Privacy Workshops (SPW)(2021)
摘要
Authorship attribution, an NLP problem where anonymous text is matched to its author, has important, cross-disciplinary applications, particularly those concerning cyber-defense. Our research examines the degree of sensitivity that attention-based models have to adversarial perturbations. We ask, what is the minimal amount of change necessary to maximally confuse a transformer model? In our investigation we examine a balanced subset of emails from the Enron email dataset, calculating the performance of our model before and after email signatures have been perturbed. Results show that the model’s performance changed significantly in the absence of a signature, indicating the importance of email signatures in email authorship detection. Furthermore, we show that these models rely on signatures for shorter emails much more than for longer emails. We also indicate that additional research is necessary to investigate stylometric features and adversarial training to further improve classification model robustness.
更多查看译文
关键词
natural language processing,authorship attribution,transformer-based networks,attention-based models,adversarial perturbation,digital forensics
AI 理解论文
溯源树
样例
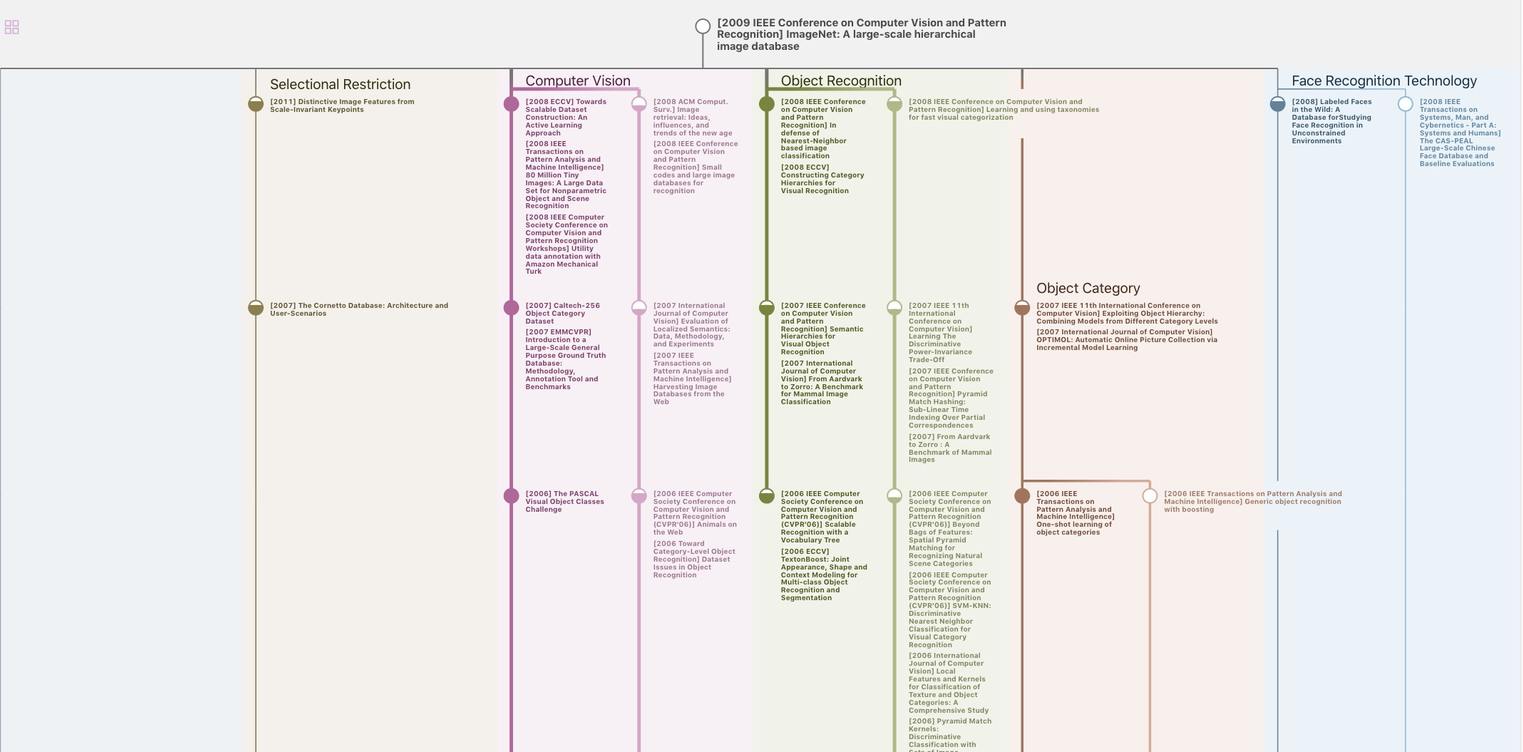
生成溯源树,研究论文发展脉络
Chat Paper
正在生成论文摘要