Direct Speech-to-Speech Translation With Discrete Units
PROCEEDINGS OF THE 60TH ANNUAL MEETING OF THE ASSOCIATION FOR COMPUTATIONAL LINGUISTICS (ACL 2022), VOL 1: (LONG PAPERS)(2022)
摘要
We present a direct speech-to-speech translation (S2ST) model that translates speech from one language to speech in another language without relying on intermediate text generation. We tackle the problem by first applying a self-supervised discrete speech encoder on the target speech and then training a sequence-to-sequence speech-to-unit translation (S2UT) model to predict the discrete representations of the target speech. When target text transcripts are available, we design a joint speech and text training framework that enables the model to generate dual modality output (speech and text) simultaneously in the same inference pass. Experiments on the Fisher Spanish-English dataset show that the proposed framework yields improvement of 6.7 BLEU compared with a baseline direct S2ST model that predicts spectrogram features. When trained without any text transcripts, our model performance is comparable to models that predict spectrograms and are trained with text supervision, showing the potential of our system for translation between unwritten languages(1).
更多查看译文
关键词
translation,direct,speech-to-speech
AI 理解论文
溯源树
样例
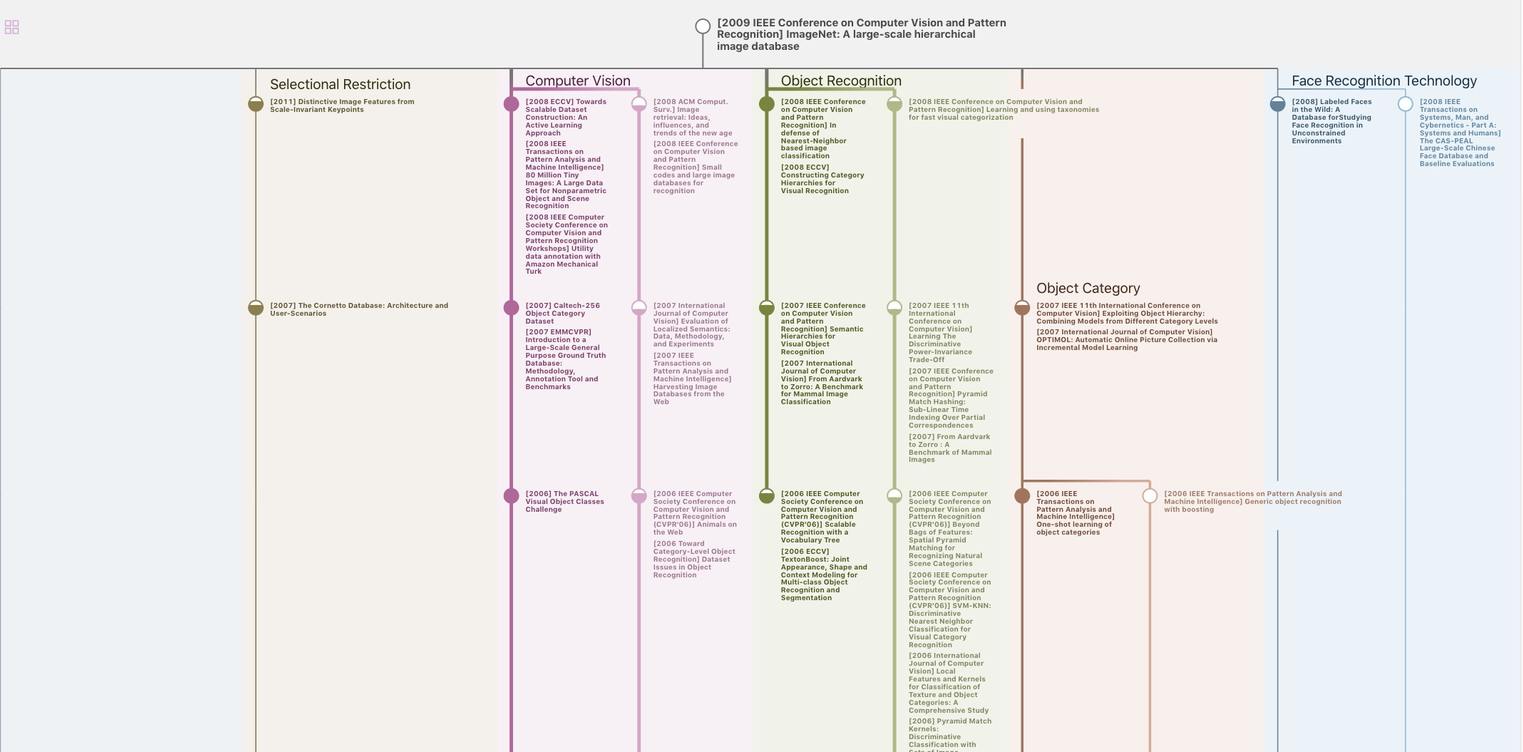
生成溯源树,研究论文发展脉络
Chat Paper
正在生成论文摘要