Sensitivity analysis in differentially private machine learning using hybrid automatic differentiation
arxiv(2021)
摘要
In recent years, formal methods of privacy protection such as differential privacy (DP), capable of deployment to data-driven tasks such as machine learning (ML), have emerged. Reconciling large-scale ML with the closed-form reasoning required for the principled analysis of individual privacy loss requires the introduction of new tools for automatic sensitivity analysis and for tracking an individual's data and their features through the flow of computation. For this purpose, we introduce a novel \textit{hybrid} automatic differentiation (AD) system which combines the efficiency of reverse-mode AD with an ability to obtain a closed-form expression for any given quantity in the computational graph. This enables modelling the sensitivity of arbitrary differentiable function compositions, such as the training of neural networks on private data. We demonstrate our approach by analysing the individual DP guarantees of statistical database queries. Moreover, we investigate the application of our technique to the training of DP neural networks. Our approach can enable the principled reasoning about privacy loss in the setting of data processing, and further the development of automatic sensitivity analysis and privacy budgeting systems.
更多查看译文
关键词
private machine,differentiation,machine learning
AI 理解论文
溯源树
样例
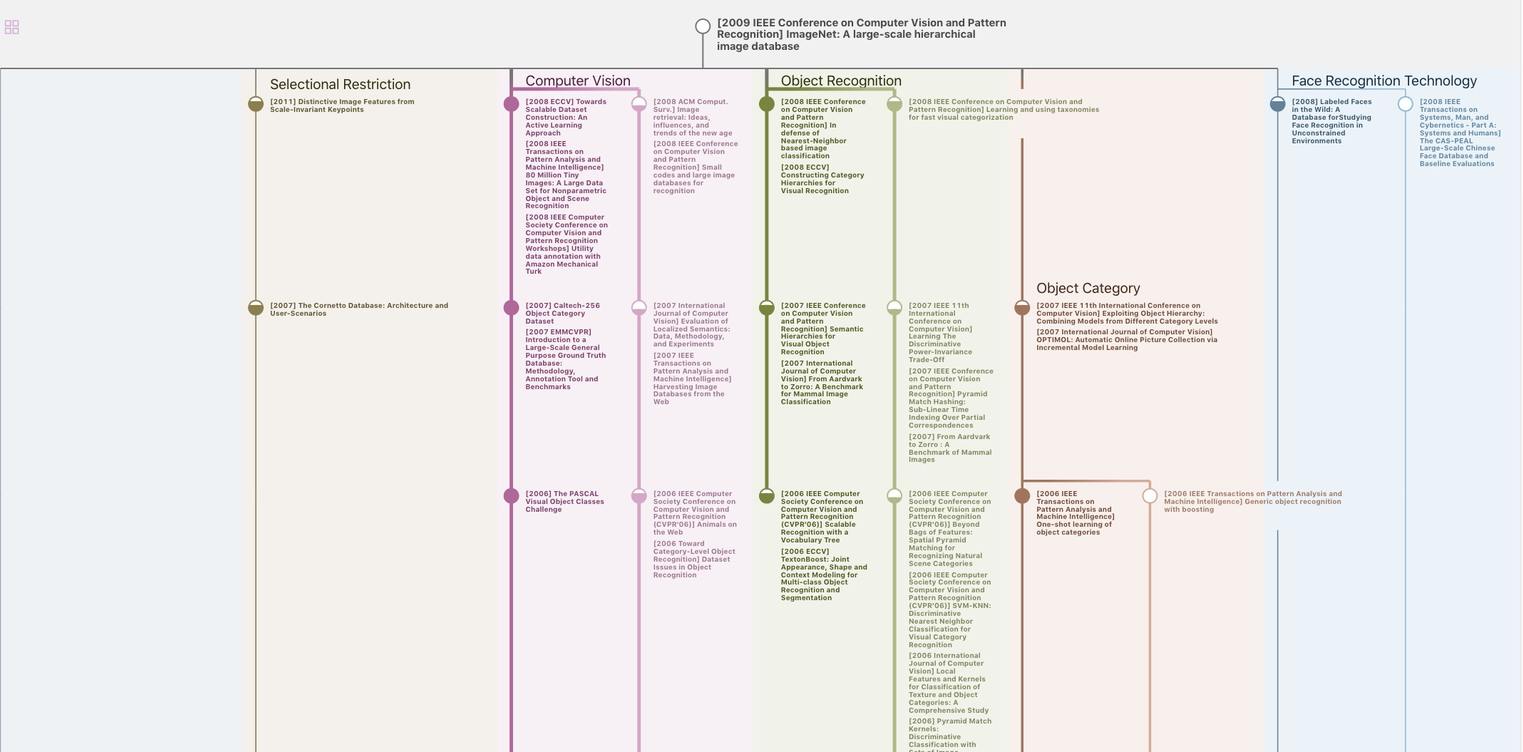
生成溯源树,研究论文发展脉络
Chat Paper
正在生成论文摘要