Self-organized criticality in neural networks
arxiv(2021)
摘要
We demonstrate, both analytically and numerically, that learning dynamics of neural networks is generically attracted towards a self-organized critical state. The effect can be modeled with quartic interactions between non-trainable variables (e.g. states of neurons) and trainable variables (e.g. weight matrix). Non-trainable variables are rapidly driven towards stochastic equilibrium and trainable variables are slowly driven towards learning equilibrium described by a scale-invariant distribution on a wide range of scales. Our results suggest that the scale invariance observed in many physical and biological systems might be due to some kind of learning dynamics and support the claim that the universe might be a neural network.
更多查看译文
关键词
criticality,neural networks,self-organized
AI 理解论文
溯源树
样例
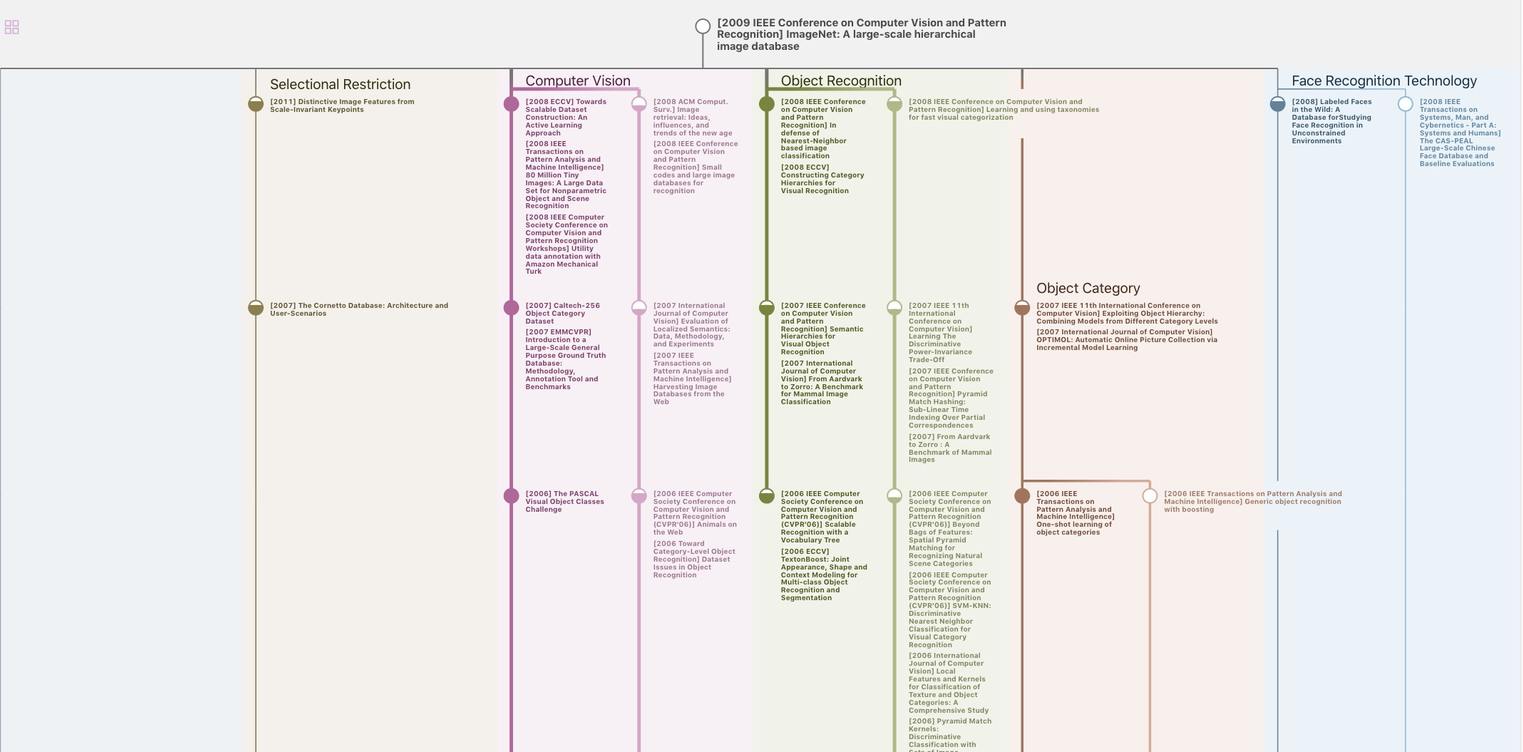
生成溯源树,研究论文发展脉络
Chat Paper
正在生成论文摘要