Stoichiometric Modeling of Artificial String Chemistries Reveals Constraints on Metabolic Network Structure
JOURNAL OF MOLECULAR EVOLUTION(2021)
摘要
Uncovering the general principles that govern the structure of metabolic networks is key to understanding the emergence and evolution of living systems. Artificial chemistries can help illuminate this problem by enabling the exploration of chemical reaction universes that are constrained by general mathematical rules. Here, we focus on artificial chemistries in which strings of characters represent simplified molecules, and string concatenation and splitting represent possible chemical reactions. We developed a novel Python package, ARtificial CHemistry NEtwork Toolbox (ARCHNET), to study string chemistries using tools from the field of stoichiometric constraint-based modeling. In addition to exploring the topological characteristics of different string chemistry networks, we developed a network-pruning algorithm that can generate minimal metabolic networks capable of producing a specified set of biomass precursors from a given assortment of environmental nutrients. We found that the composition of these minimal metabolic networks was influenced more strongly by the metabolites in the biomass reaction than the identities of the environmental nutrients. This finding has important implications for the reconstruction of organismal metabolic networks and could help us better understand the rise and evolution of biochemical organization. More generally, our work provides a bridge between artificial chemistries and stoichiometric modeling, which can help address a broad range of open questions, from the spontaneous emergence of an organized metabolism to the structure of microbial communities.
更多查看译文
关键词
Artificial chemistry, Flux balance analysis, Metabolism, Genome-scale metabolic models
AI 理解论文
溯源树
样例
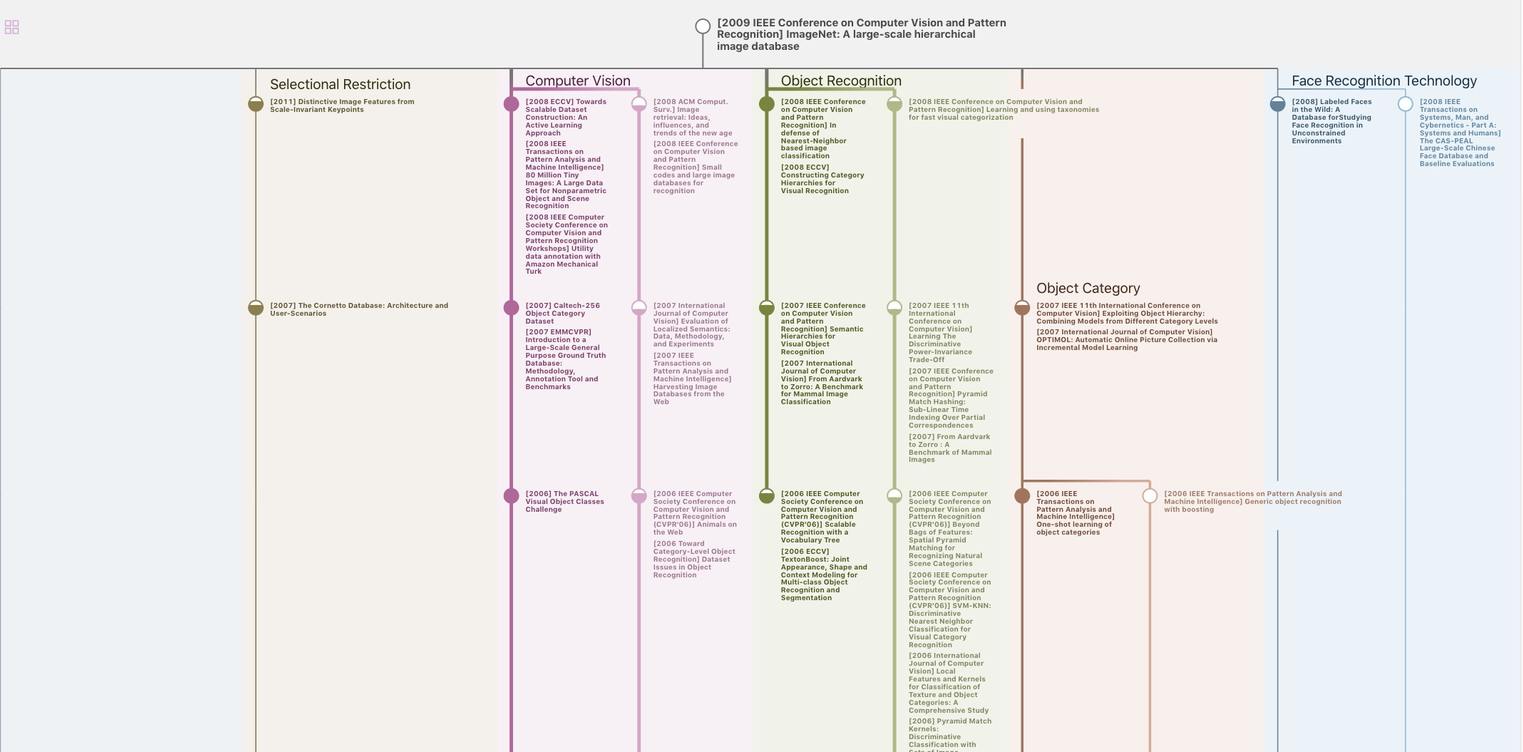
生成溯源树,研究论文发展脉络
Chat Paper
正在生成论文摘要