Towards Improved and Interpretable Action Quality Assessment with Self-Supervised Alignment.
PETRA(2021)
摘要
Action Quality Assessment (AQA) is a video understanding task aiming at the quantification of the execution quality of an action. One of the main challenges in relevant, deep learning-based approaches is the collection of training data annotated by experts. Current methods perform fine-tuning on pre-trained backbone models and aim to improve performance by modeling the subjects and the scene. In this work, we consider embeddings extracted using a self-supervised training method based on a differential cycle consistency loss between sequences of actions. These are shown to improve the state-of-the-art without the need for additional annotations or scene modeling. The same embeddings are also used to temporally align the sequences prior to quality assessment which further increases the accuracy, provides robustness to variance in execution speed and enables us to provide fine-grained interpretability of the assessment score. The experimental evaluation of the method on the MTL-AQA dataset demonstrates significant accuracy gain compared to the state-of-the-art baselines, which grows even more when the action execution sequences are not well aligned.
更多查看译文
AI 理解论文
溯源树
样例
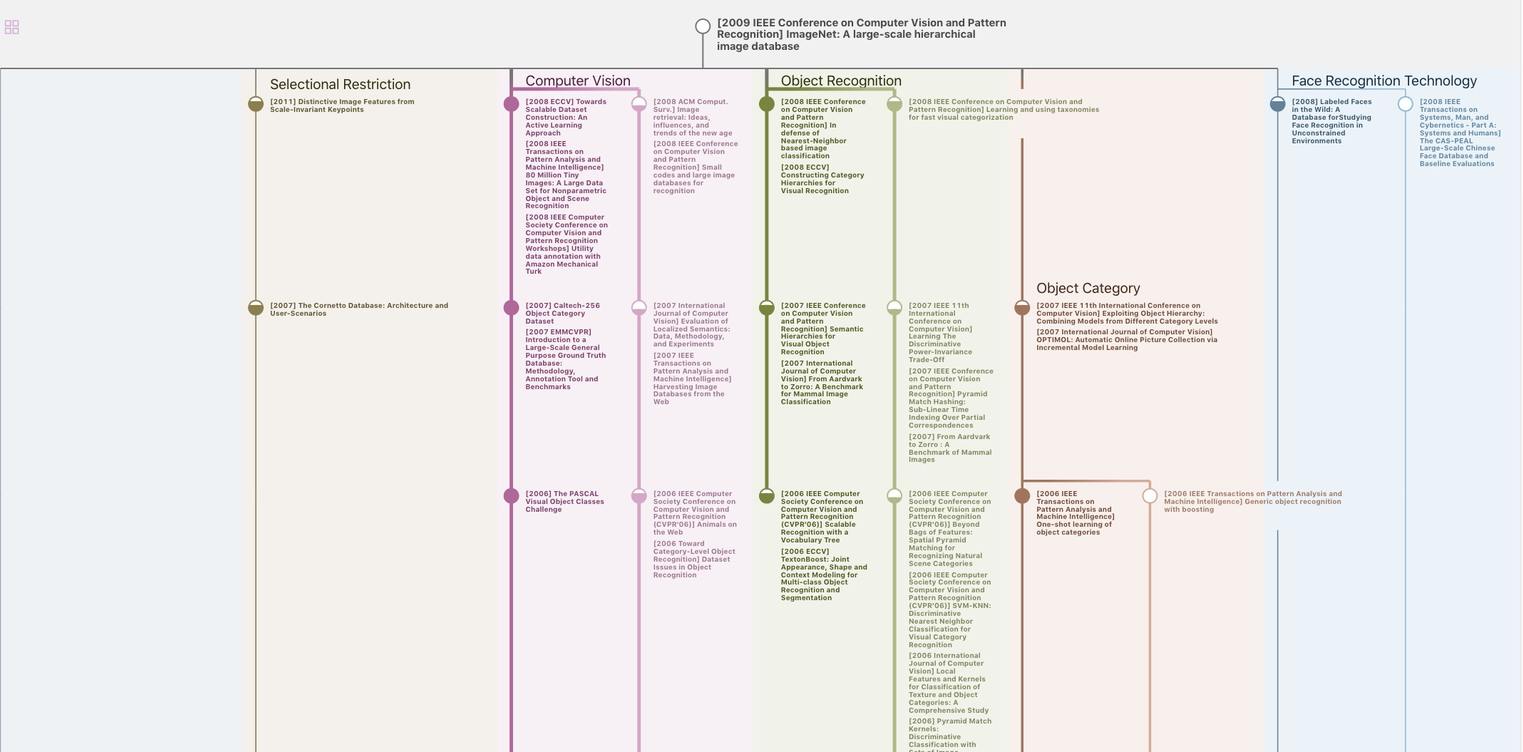
生成溯源树,研究论文发展脉络
Chat Paper
正在生成论文摘要