Distance-based Hyperspherical Classification for Multi-source Open-Set Domain Adaptation
2022 IEEE WINTER CONFERENCE ON APPLICATIONS OF COMPUTER VISION (WACV 2022)(2022)
摘要
Vision systems trained in closed-world scenarios fail when presented with new environmental conditions, new data distributions, and novel classes at deployment time. How to move towards open-world learning is a long-standing research question. The existing solutions mainly focus on specific aspects of the problem (single domain Open-Set, multi-domain Closed-Set), or propose complex strategies which combine several losses and manually tuned hyperparameters. In this work, we tackle multi-source Open-Set domain adaptation by introducing HyMOS: a straightforward model that exploits the power of contrastive learning and the properties of its hyperspherical feature space to correctly predict known labels on the target, while rejecting samples belonging to any unknown class. HyMOS includes style transfer among the instance transformations of contrastive learning to get domain invariance while avoiding the risk of negative-transfer. A self-paced threshold is defined on the basis of the observed data distribution and updates online during training, allowing to handle the known-unknown separation. We validate our method over three challenging datasets. The obtained results show that HyMOS outperforms several competitors, defining the new state-of-the-art. Our code is available at https://github.com/silvia1993/HyMOS.
更多查看译文
关键词
domain adaptation,hyperspherical classification,distance-based,multi-source,open-set
AI 理解论文
溯源树
样例
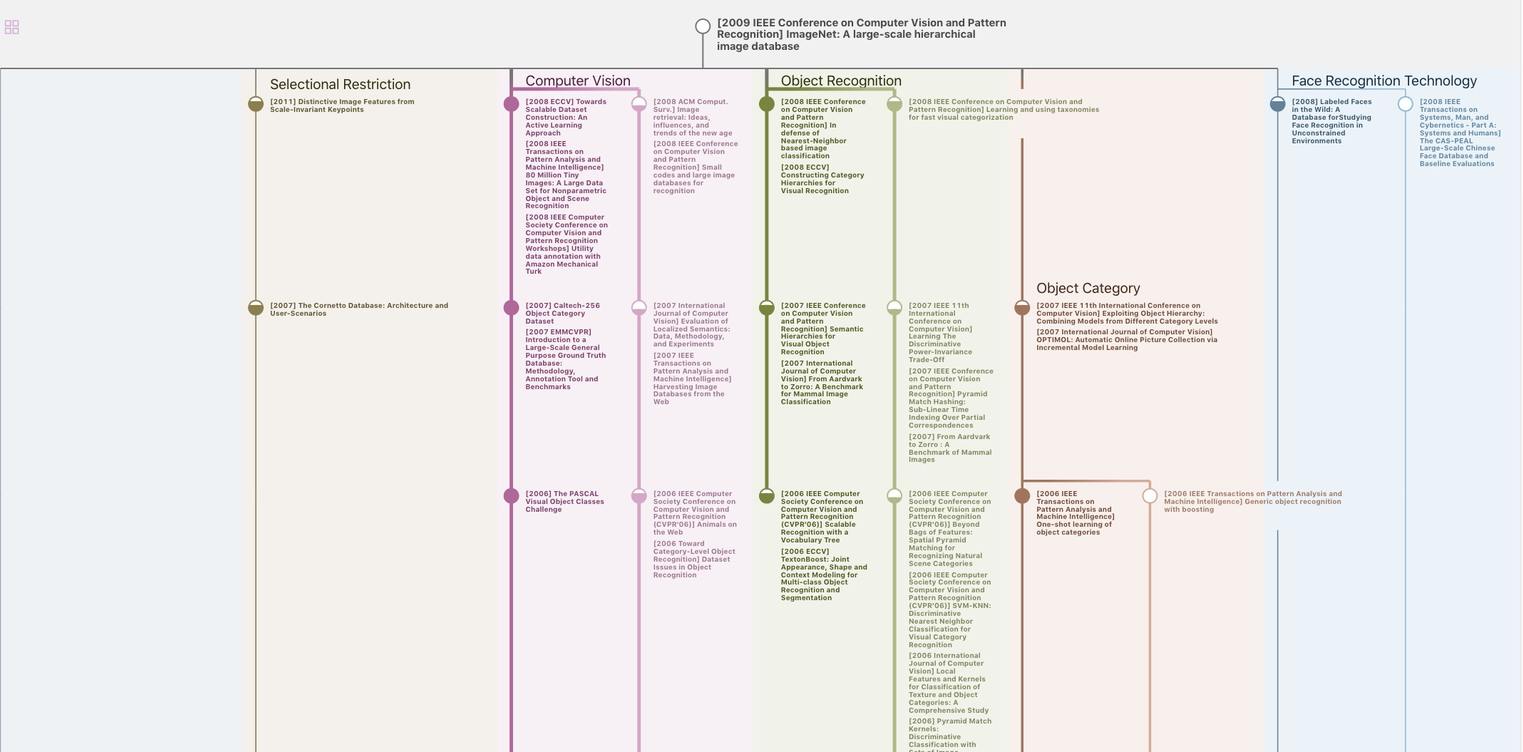
生成溯源树,研究论文发展脉络
Chat Paper
正在生成论文摘要