KAISA: an adaptive second-order optimizer framework for deep neural networks
The International Conference for High Performance Computing, Networking, Storage, and Analysis(2021)
摘要
ABSTRACTKronecker-factored Approximate Curvature (K-FAC) has recently been shown to converge faster in deep neural network (DNN) training than stochastic gradient descent (SGD); however, K-FAC's larger memory footprint hinders its applicability to large models. We present KAISA, a K-FAC-enabled, Adaptable, Improved, and ScAlable second-order optimizer framework that adapts the memory footprint, communication, and computation given specific models and hardware to improve performance and increase scalability. We quantify the tradeoffs between memory and communication cost and evaluate KAISA on large models, including ResNet-50, Mask R-CNN, U-Net, and BERT, on up to 128 NVIDIA A100 GPUs. Compared to the original optimizers, KAISA converges 18.1--36.3% faster across applications with the same global batch size. Under a fixed memory budget, KAISA converges 32.5% and 41.6% faster in ResNet-50 and BERT-Large, respectively. KAISA can balance memory and communication to achieve scaling efficiency equal to or better than the baseline optimizers.
更多查看译文
关键词
Machine Learning,Distributed Computing,Second-Order Optimization,K-FAC,Data-Parallel Algorithms
AI 理解论文
溯源树
样例
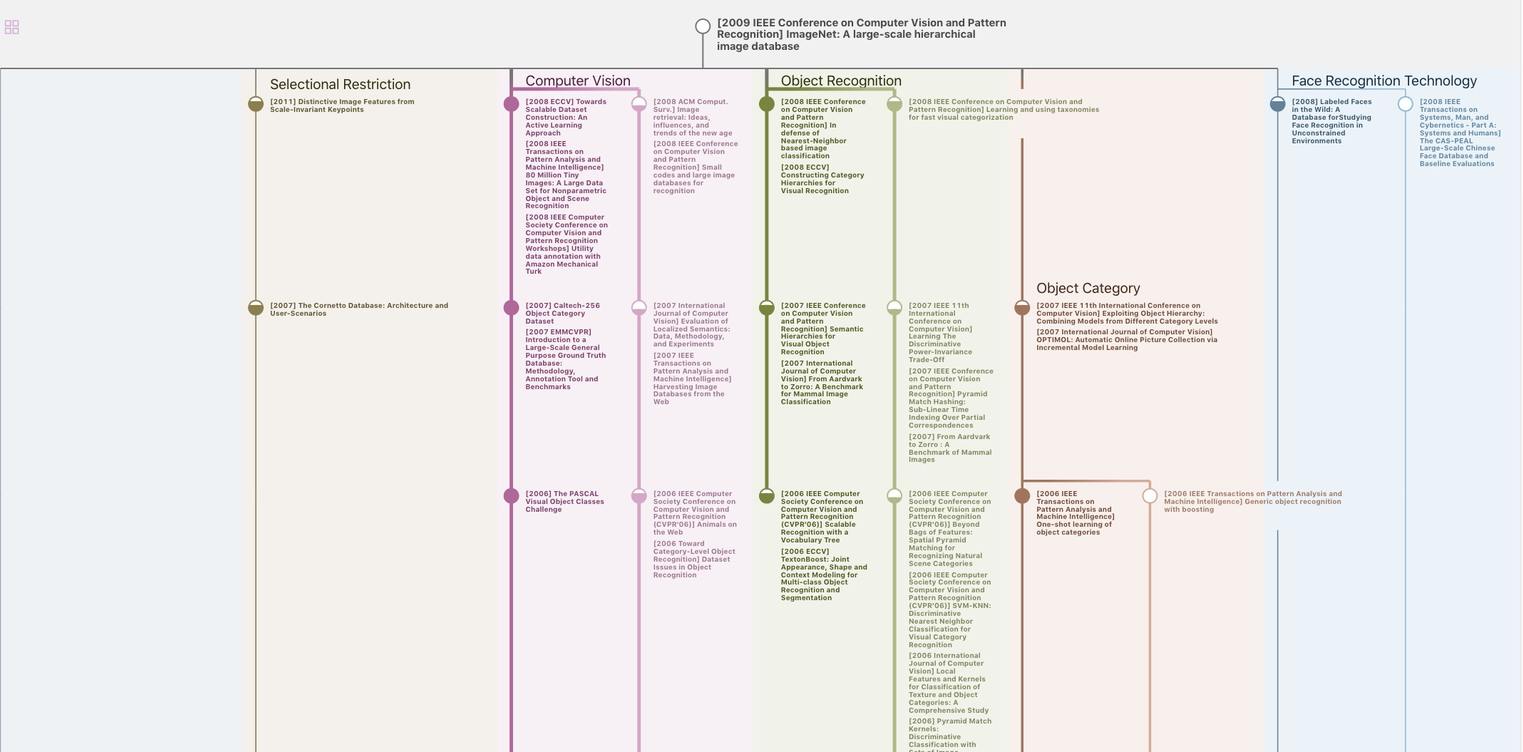
生成溯源树,研究论文发展脉络
Chat Paper
正在生成论文摘要