Combinatorial optimization with physics-inspired graph neural networks.
PHYSICAL REVIEW RESEARCH(2022)
摘要
We show how graph neural networks can be used to solve the canonical graph coloring problem. We frame graph coloring as a multiclass node classification problem and utilize an unsupervised training strategy based on the statistical physics Potts model. Generalizations to other multiclass problems such as community detection, data clustering, and the minimum clique cover problem are straightforward. We provide numerical benchmark results and illustrate our approach with an end-to-end application for a real-world scheduling use case within a comprehensive encode-process-decode framework. Our optimization approach performs on par or outperforms existing solvers, with the ability to scale to problems with millions of variables.
更多查看译文
关键词
Computer science,Operational research,Quantum information,Statistical physics,Engineering,general
AI 理解论文
溯源树
样例
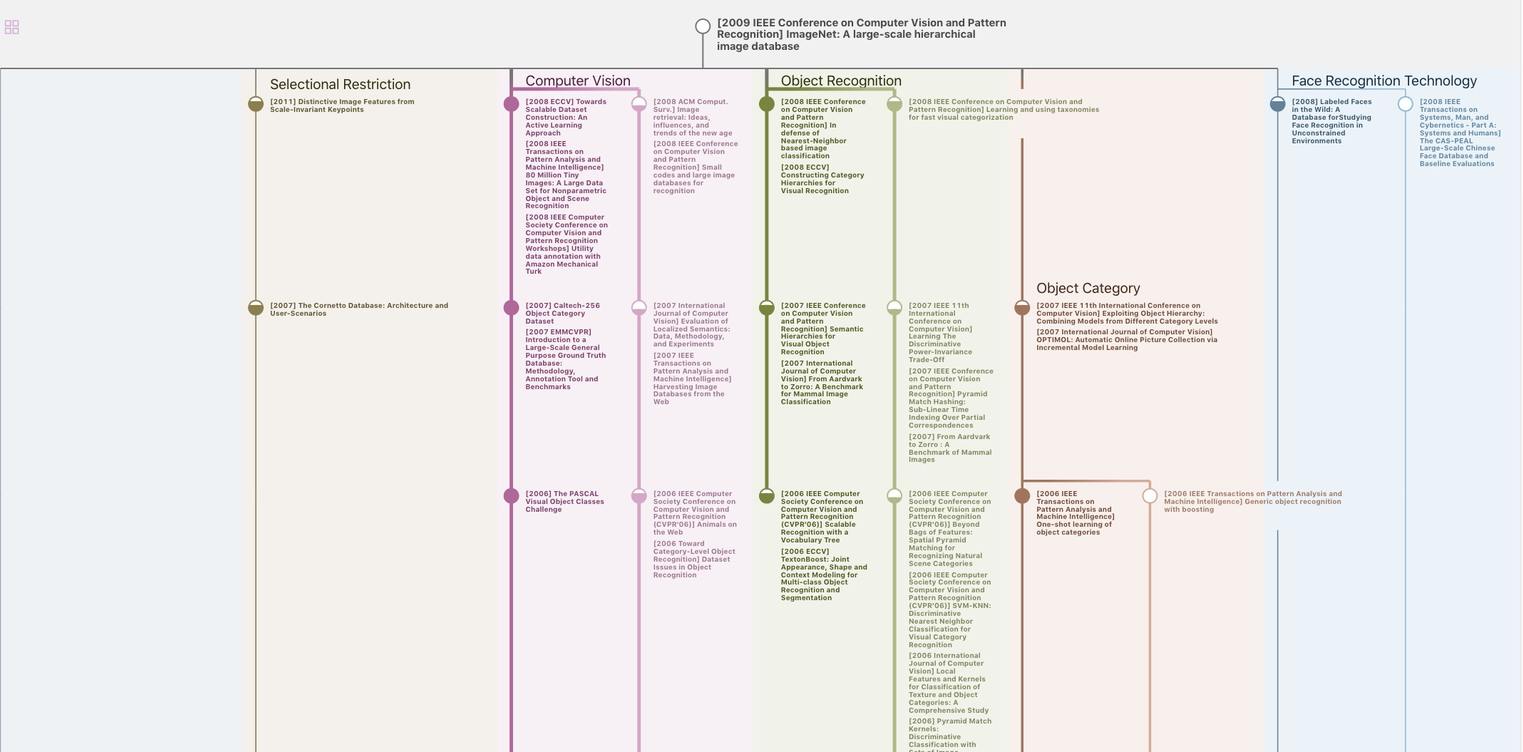
生成溯源树,研究论文发展脉络
Chat Paper
正在生成论文摘要