Shared Data and Algorithms for Deep Learning in Fundamental Physics
Computing and Software for Big Science(2022)
摘要
We introduce a Python package that provides simple and unified access to a collection of datasets from fundamental physics research—including particle physics, astroparticle physics, and hadron- and nuclear physics—for supervised machine learning studies. The datasets contain hadronic top quarks, cosmic-ray-induced air showers, phase transitions in hadronic matter, and generator-level histories. While public datasets from multiple fundamental physics disciplines already exist, the common interface and provided reference models simplify future work on cross-disciplinary machine learning and transfer learning in fundamental physics. We discuss the design and structure and line out how additional datasets can be submitted for inclusion. As showcase application, we present a simple yet flexible graph-based neural network architecture that can easily be applied to a wide range of supervised learning tasks. We show that our approach reaches performance close to dedicated methods on all datasets. To simplify adaptation for various problems, we provide easy-to-follow instructions on how graph-based representations of data structures, relevant for fundamental physics, can be constructed and provide code implementations for several of them. Implementations are also provided for our proposed method and all reference algorithms.
更多查看译文
关键词
Deep learning,Fundamental physics,Datasets
AI 理解论文
溯源树
样例
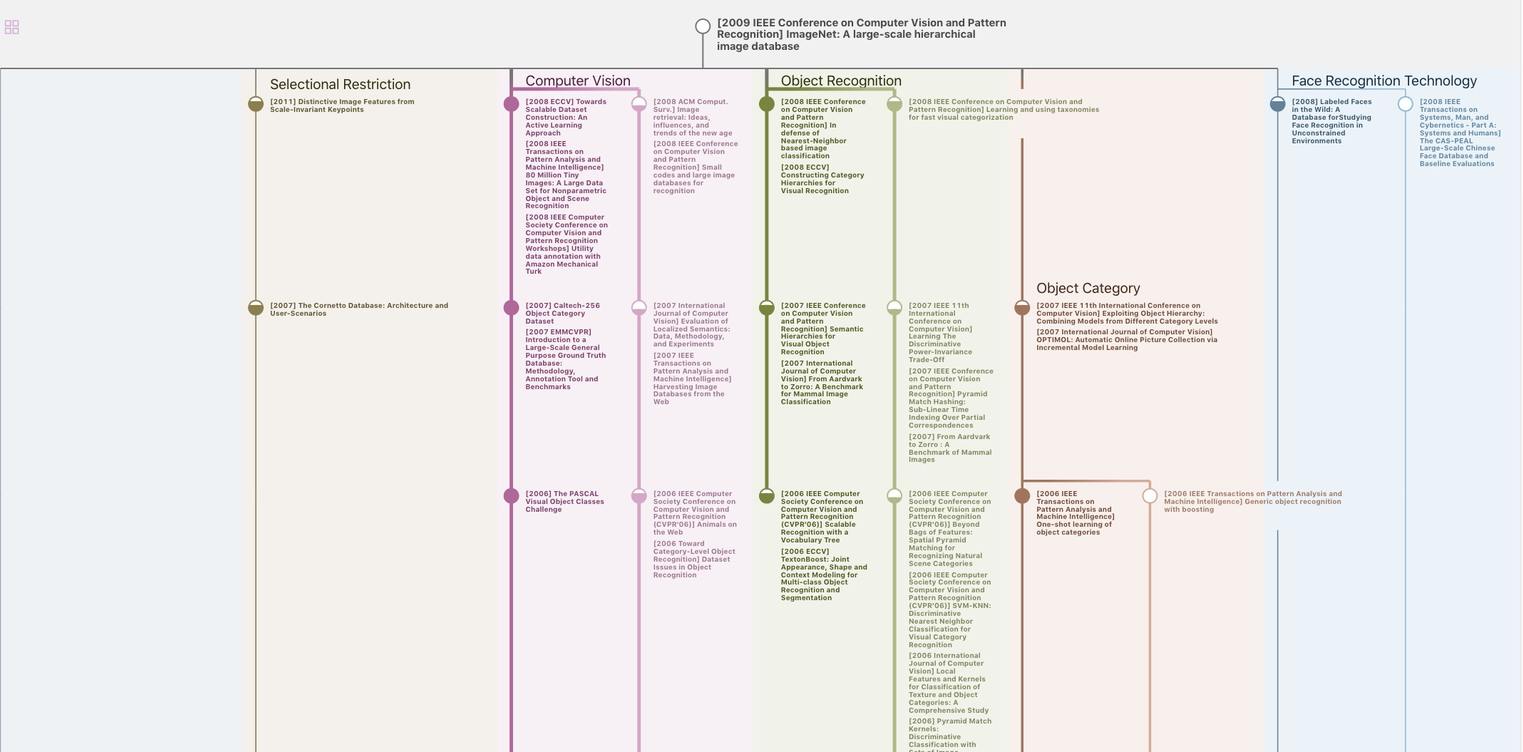
生成溯源树,研究论文发展脉络
Chat Paper
正在生成论文摘要