End-to-end privacy preserving deep learning on multi-institutional medical imaging
NATURE MACHINE INTELLIGENCE(2021)
摘要
Using large, multi-national datasets for high-performance medical imaging AI systems requires innovation in privacy-preserving machine learning so models can train on sensitive data without requiring data transfer. Here we present PriMIA (Privacy-preserving Medical Image Analysis), a free, open-source software framework for differentially private, securely aggregated federated learning and encrypted inference on medical imaging data. We test PriMIA using a real-life case study in which an expert-level deep convolutional neural network classifies paediatric chest X-rays; the resulting model’s classification performance is on par with locally, non-securely trained models. We theoretically and empirically evaluate our framework’s performance and privacy guarantees, and demonstrate that the protections provided prevent the reconstruction of usable data by a gradient-based model inversion attack. Finally, we successfully employ the trained model in an end-to-end encrypted remote inference scenario using secure multi-party computation to prevent the disclosure of the data and the model.
更多查看译文
关键词
Computer science,Radiography,Engineering,general
AI 理解论文
溯源树
样例
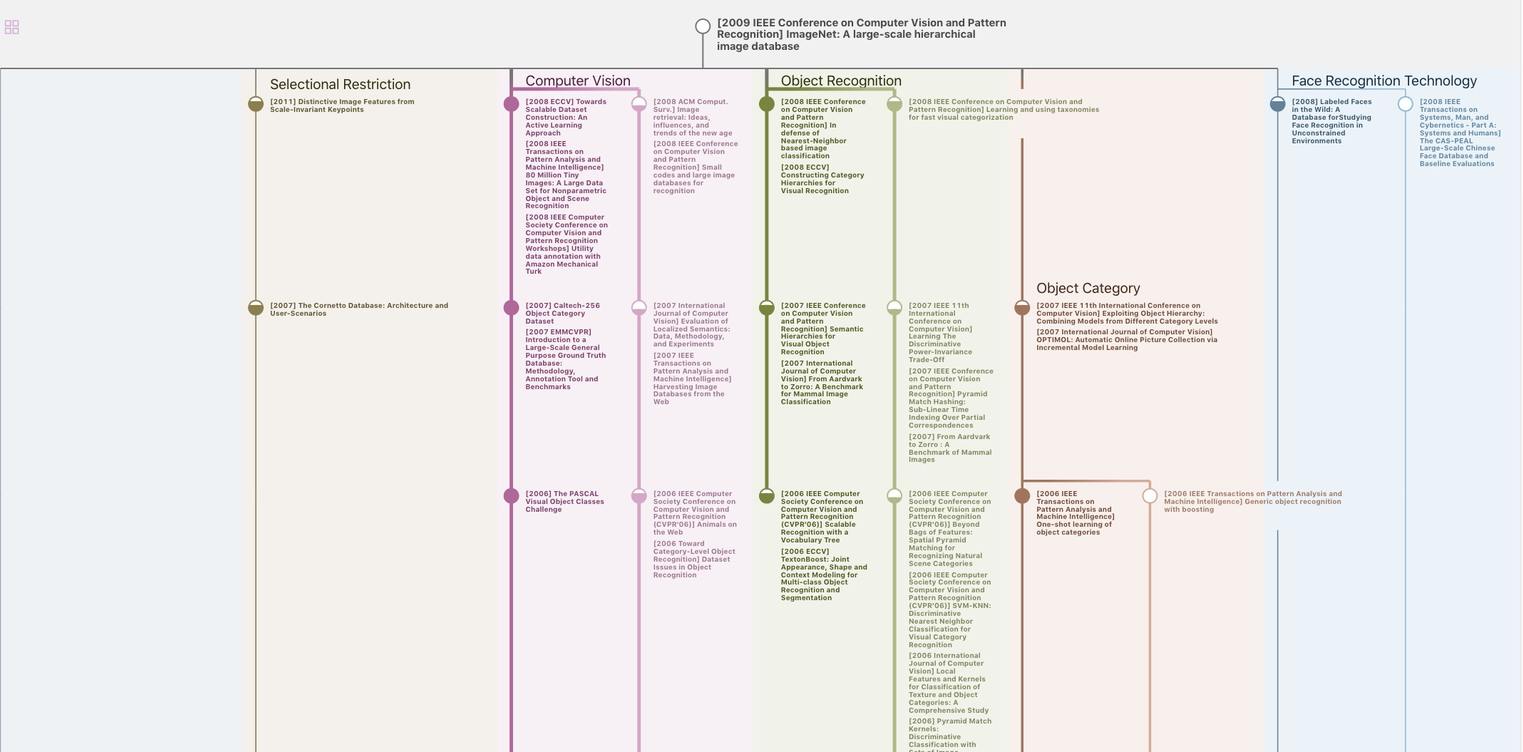
生成溯源树,研究论文发展脉络
Chat Paper
正在生成论文摘要