A computed tomography signs quantization analysis method for pulmonary nodules malignancy grading
INTERNATIONAL JOURNAL OF IMAGING SYSTEMS AND TECHNOLOGY(2021)
摘要
In order to improve the accuracy of pulmonary nodules malignancy grading, we propose a method to implement quantitative analysis for lung nodules using computed tomography (CT) signs. Firstly, we construct feature sets of CT signs by combing the radiomics features with the higher-order features extracted from a convolutional neural network. Secondly, on the basis of the mixed feature set, an evolutionary ensemble learning mechanism is used to generate a classifier to get the quantitative scores for seven lung nodule CT signs. Finally, the scores of seven CT signs are input into a multiclassifier optimized by the differential evolution algorithm to acquire the grade of malignancy. In the experimental study, 2000 lung nodule samples from the LIDC-IDRI dataset were used to train and test the evolutionary ensemble learner and malignancy classifier. The results show that the recognition accuracy of seven CT signs can reach more than 0.964. Comparison with many typical algorithms, the proposed method not only gets higher accuracy in pulmonary nodules malignancy grading but also can make the result more interpretable.
更多查看译文
关键词
CT signs, evolutionary ensemble learning, grade of malignancy, quantization analysis
AI 理解论文
溯源树
样例
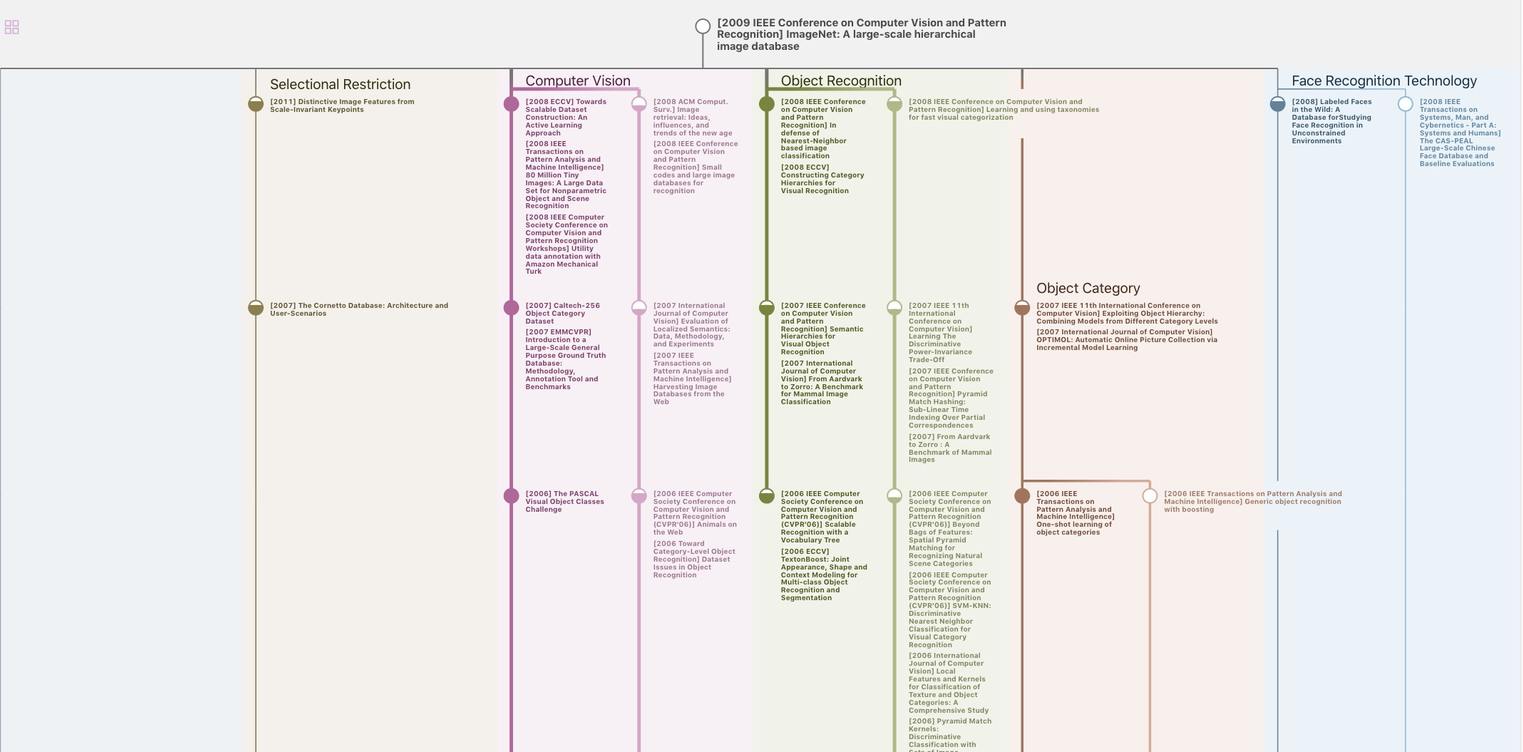
生成溯源树,研究论文发展脉络
Chat Paper
正在生成论文摘要