A visual analytics approach to anomaly detection in hydrocarbon reservoir time series data
Journal of Petroleum Science and Engineering(2021)
摘要
Detecting anomalies in time series data of hydrocarbon reservoir production is crucially important. Anomalies can result for different reasons: gross errors, system availability, human intervention, or abrupt changes in the series. They must be identified due to their potential to alter the series correlation, influence data-driven forecast, and affect classification results. We have developed a visual analytics approach based on an interactive visualization of time series data involving machine learning approaches for anomaly identification. Our methods rely upon a z-score normalization technique along with isolation forests. The methods leverage the prior probability of anomalies from a time-window, do not require labeled training data with normal and abnormal conditions, and incorporate specialist knowledge in the exploration process. We apply, evaluate, and discuss the methods’ capability using a benchmark data set (UNISIM–II–M-CO) and real field data in three visual exploration setups. The ground-truth annotations were done by human specialists and considered different interventions in the reservoir. Our methods detect approximately 95% of the human intervention anomalies, and about 82%–89% detection rate for other anomalies identified during data exploration.
更多查看译文
关键词
Hydrocarbon reservoir,Anomaly detection,Time series,Visual analytics
AI 理解论文
溯源树
样例
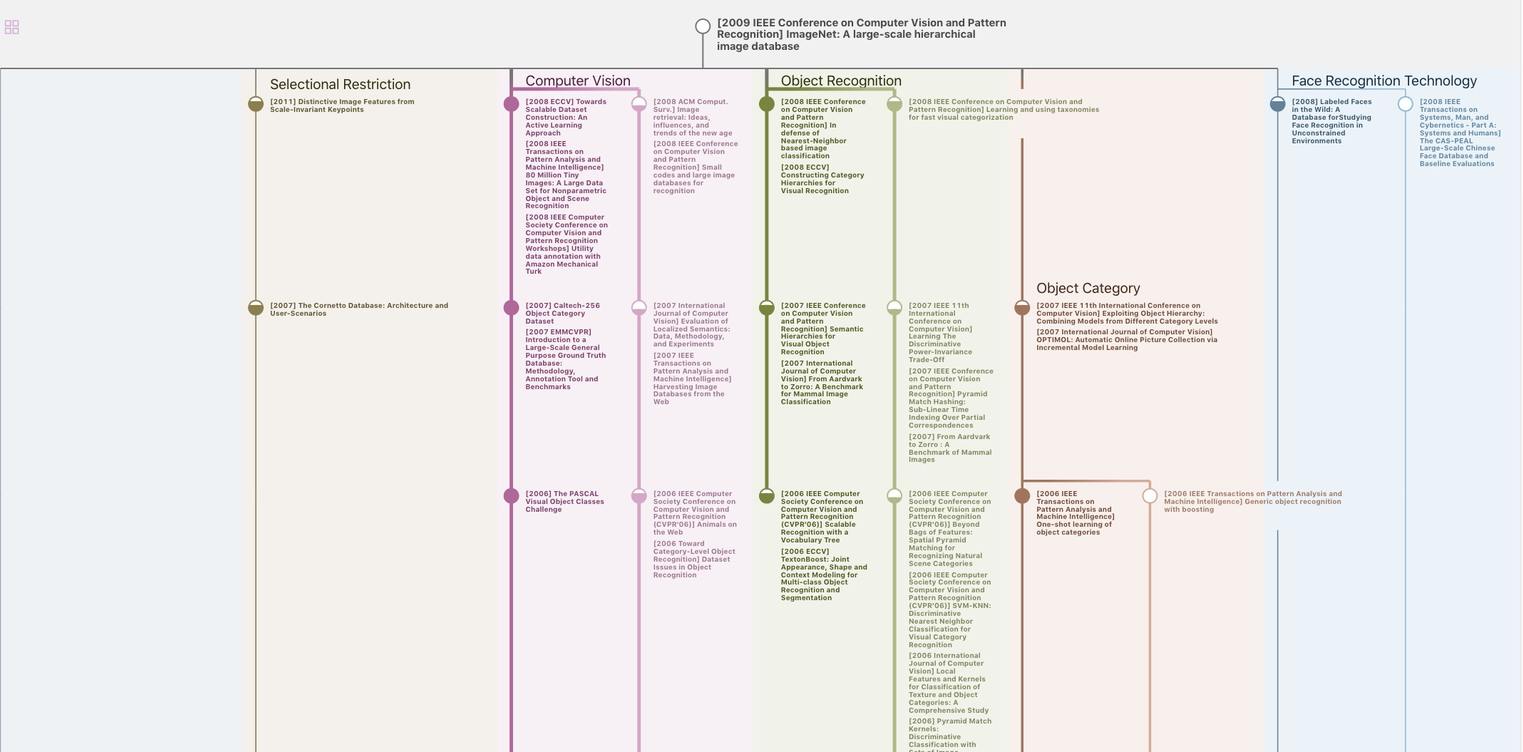
生成溯源树,研究论文发展脉络
Chat Paper
正在生成论文摘要