A Survey on Stream-Based Recommender Systems
ACM Computing Surveys(2021)
摘要
AbstractRecommender Systems (RS) have proven to be effective tools to help users overcome information overload, and significant advances have been made in the field over the past two decades. Although addressing the recommendation problem required first a formulation that could be easily studied and evaluated, there currently exists a gap between research contributions and industrial applications where RS are actually deployed. In particular, most RS are meant to function in batch: they rely on a large static dataset and build a recommendation model that is only periodically updated. This functioning introduces several limitations in various settings, leading to considering more realistic settings where RS learn from continuous streams of interactions. Such RS are framed as Stream-Based Recommender Systems (SBRS).In this article, we review SBRS, underline their relation with time-aware RS and online adaptive learning, and present and categorize existing work that tackle the corresponding problem and its multiple facets. We discuss the methodologies used to evaluate SBRS and the adapted datasets that can be used, and finally we outline open challenges in the area.
更多查看译文
关键词
Personalization, recommendation, data streams, concept drifts
AI 理解论文
溯源树
样例
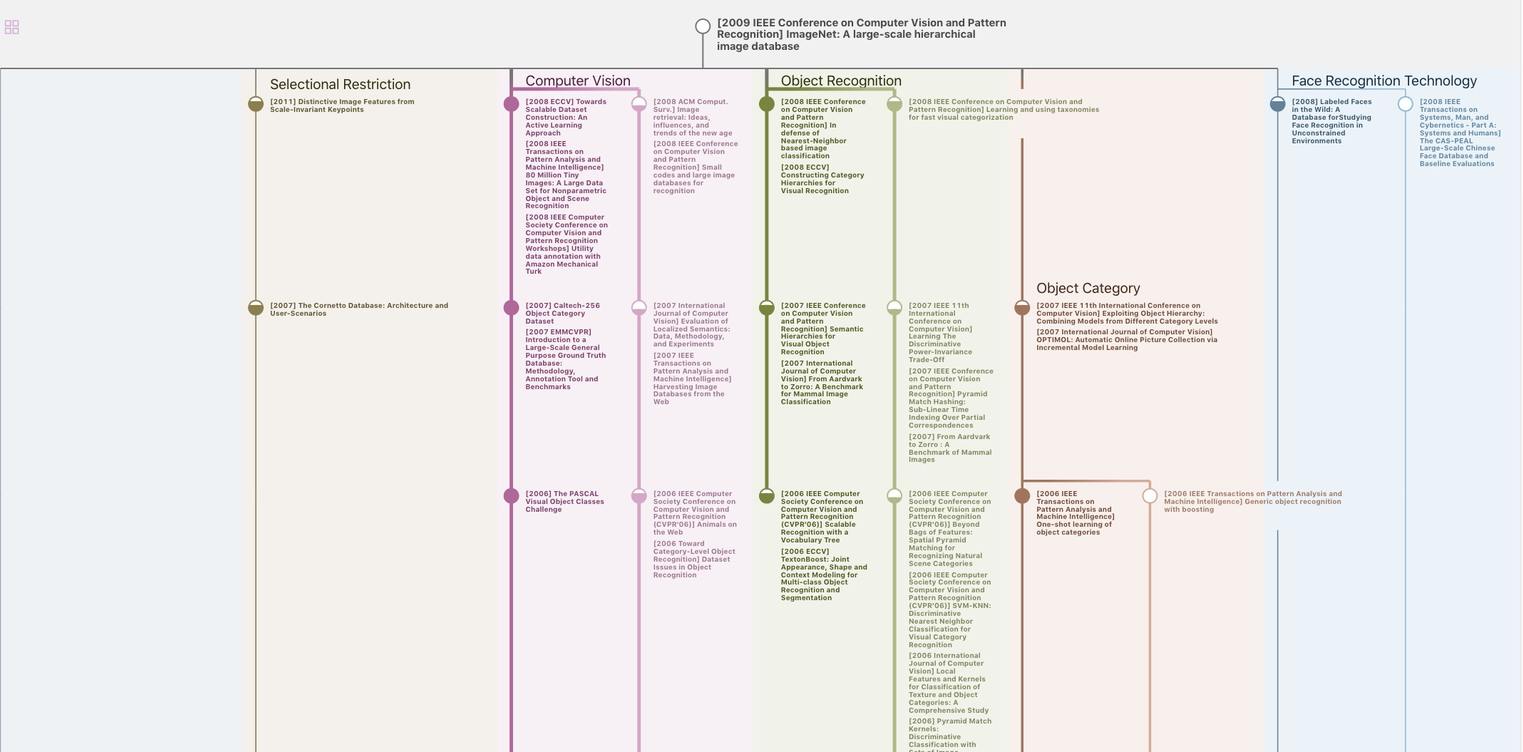
生成溯源树,研究论文发展脉络
Chat Paper
正在生成论文摘要