MULTI-SCALE CASCADE DISPARITY REFINEMENT STEREO NETWORK
2021 IEEE INTERNATIONAL CONFERENCE ON ACOUSTICS, SPEECH AND SIGNAL PROCESSING (ICASSP 2021)(2021)
摘要
Stereo matching has attracted much attention in recent years. Traditional methods can quickly generate a disparity result, but the accuracy is low. On the contrary, methods based on neural networks can achieve a high accuracy level, but they are difficult to reach the real-time level. Therefore, this paper presents MCDRNet, which combines traditional methods with neural networks to achieve real-time and accurate stereo matching results. Concretely, our network first generates a rough disparity map based on the traditional ADCensus algorithm. Then we design a novel Multi-Scale Cascade Network to refine the disparity map from coarse to fine. We evaluate our best-trained model on the KITTI official website. The results show that our network is much faster than most current top-performing methods(31xthan CSPN, 56xthan GANet, etc.). Meanwhile, it is more accurate than traditional stereo methods(SGM, SPS-St) and other fast 2D convolution networks(Fast DS-CS, DispNetC, etc.), demonstrating the rationalities and feasibilities of our method.
更多查看译文
关键词
Computer vision, Stereo matching, Depth estimation, Traditional method, Neural network
AI 理解论文
溯源树
样例
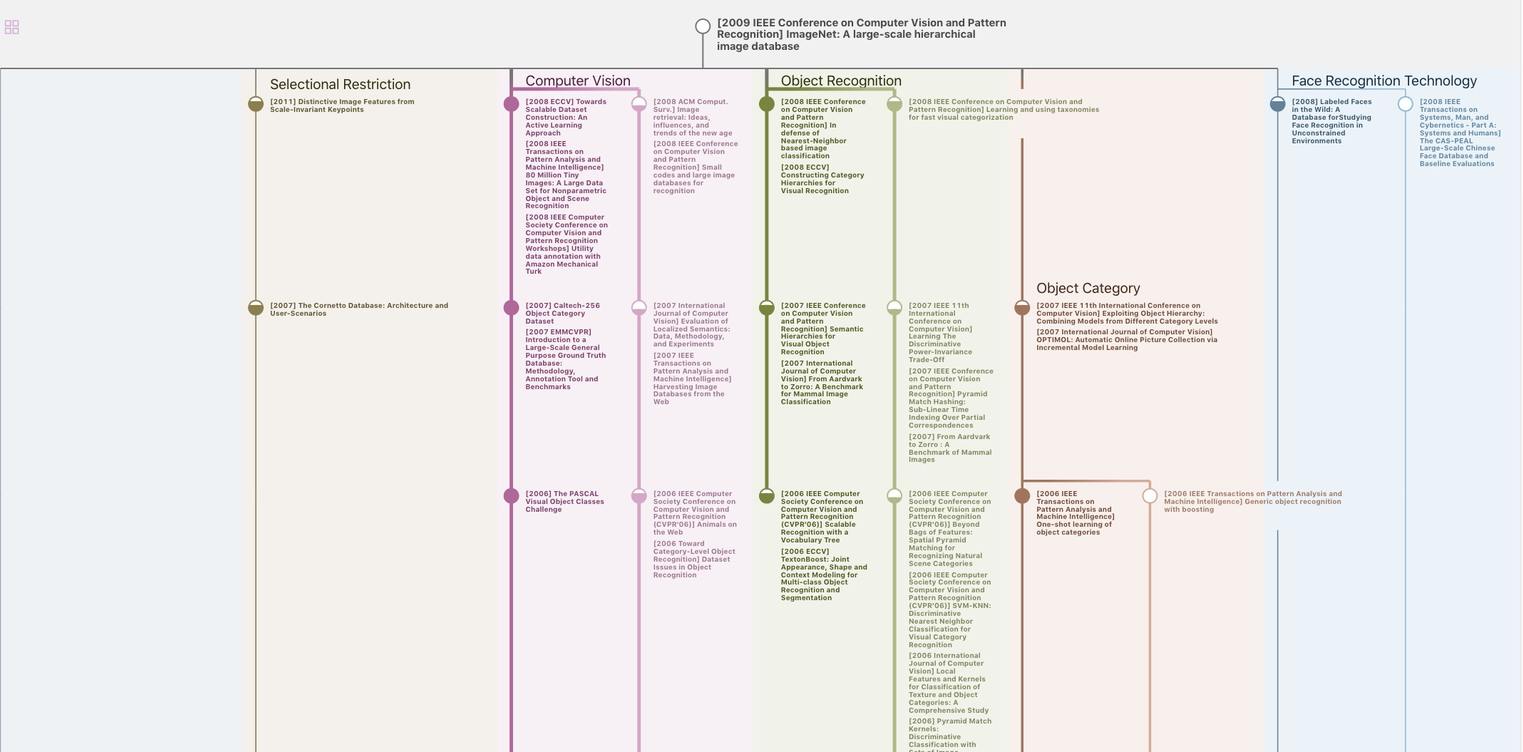
生成溯源树,研究论文发展脉络
Chat Paper
正在生成论文摘要