Prediction of student attrition risk using machine learning
JOURNAL OF APPLIED RESEARCH IN HIGHER EDUCATION(2022)
摘要
Purpose The prediction of student attrition is critical to facilitate retention mechanisms. This study aims to focus on implementing a method to predict student attrition in the upper years of a physiotherapy program. Design/methodology/approach Machine learning is a computer tool that can recognize patterns and generate predictive models. Using a quantitative research methodology, a database of 336 university students in their upper-year courses was accessed. The participant's data were collected from the Financial Academic Management and Administration System and a platform of Universidad Autonoma de Chile. Five quantitative and 11 qualitative variables were chosen, associated with university student attrition. With this database, 23 classifiers were tested based on supervised machine learning. Findings About 23.58% of males and 17.39% of females were among the attrition student group. The mean accuracy of the classifiers increased based on the number of variables used for the training. The best accuracy level was obtained using the "Subspace KNN" algorithm (86.3%). The classifier "RUSboosted trees" yielded the lowest number of false negatives and the higher sensitivity of the algorithms used (78%) as well as a specificity of 86%. Practical implications This predictive method identifies attrition students in the university program and could be used to improve student retention in higher grades. Originality/value The study has developed a novel predictive model of student attrition from upper-year courses, useful for unbalanced databases with a lower number of attrition students.
更多查看译文
关键词
Student attrition, Supervised machine learning, Data classification, University student
AI 理解论文
溯源树
样例
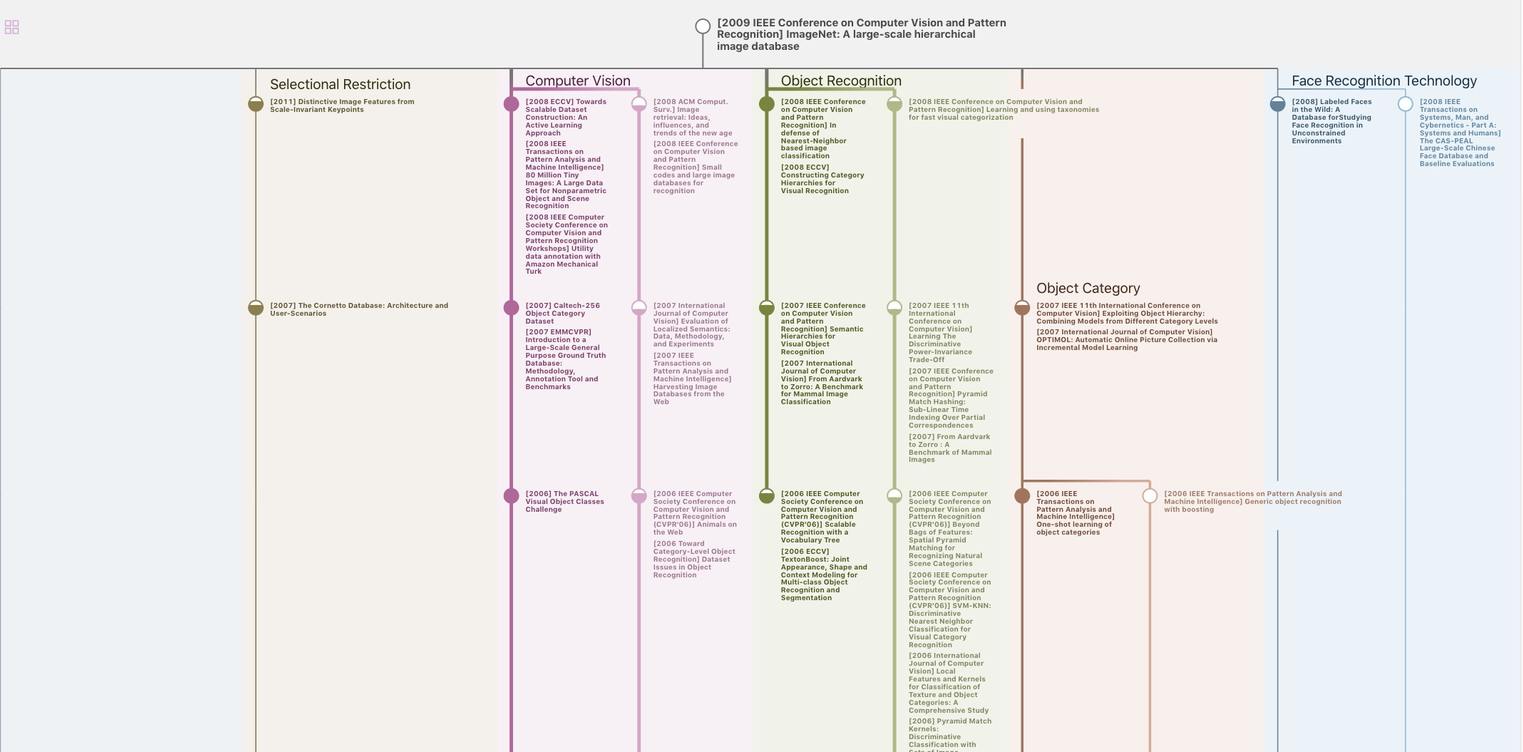
生成溯源树,研究论文发展脉络
Chat Paper
正在生成论文摘要