SPEEDING UP OF KERNEL-BASED LEARNING FOR HIGH-ORDER TENSORS
2021 IEEE INTERNATIONAL CONFERENCE ON ACOUSTICS, SPEECH AND SIGNAL PROCESSING (ICASSP 2021)(2021)
摘要
Supervised learning is a major task to classify datasets. In our context, we are interested into classification from high-order tensors datasets. The "curse of dimensionality" states that the complexities in terms of storage and computation grow exponentially with the order. As a consequence, the method from the state-of-art based on the Higher-Order SVD (HOSVD) works well but suffers from severe limitation in terms of complexities. In this work, we propose a fast Grassmannian kernel-based method for high-order tensor learning based on the equivalence between the Tucker and the tensor-train decompositions. Our solution is linked to the tensor network, where the aim is to break the initial high-order tensor into a collection of low-order tensors (at most 3-order). We show on several real datasets that the proposed method reaches a similar accuracy classification rate as the Grassmannian kernel-based method based on the HOSVD but for a much lower complexity.
更多查看译文
关键词
Tensor classification, HOSVD, subspaces, Grassman manifold, Tensor Train
AI 理解论文
溯源树
样例
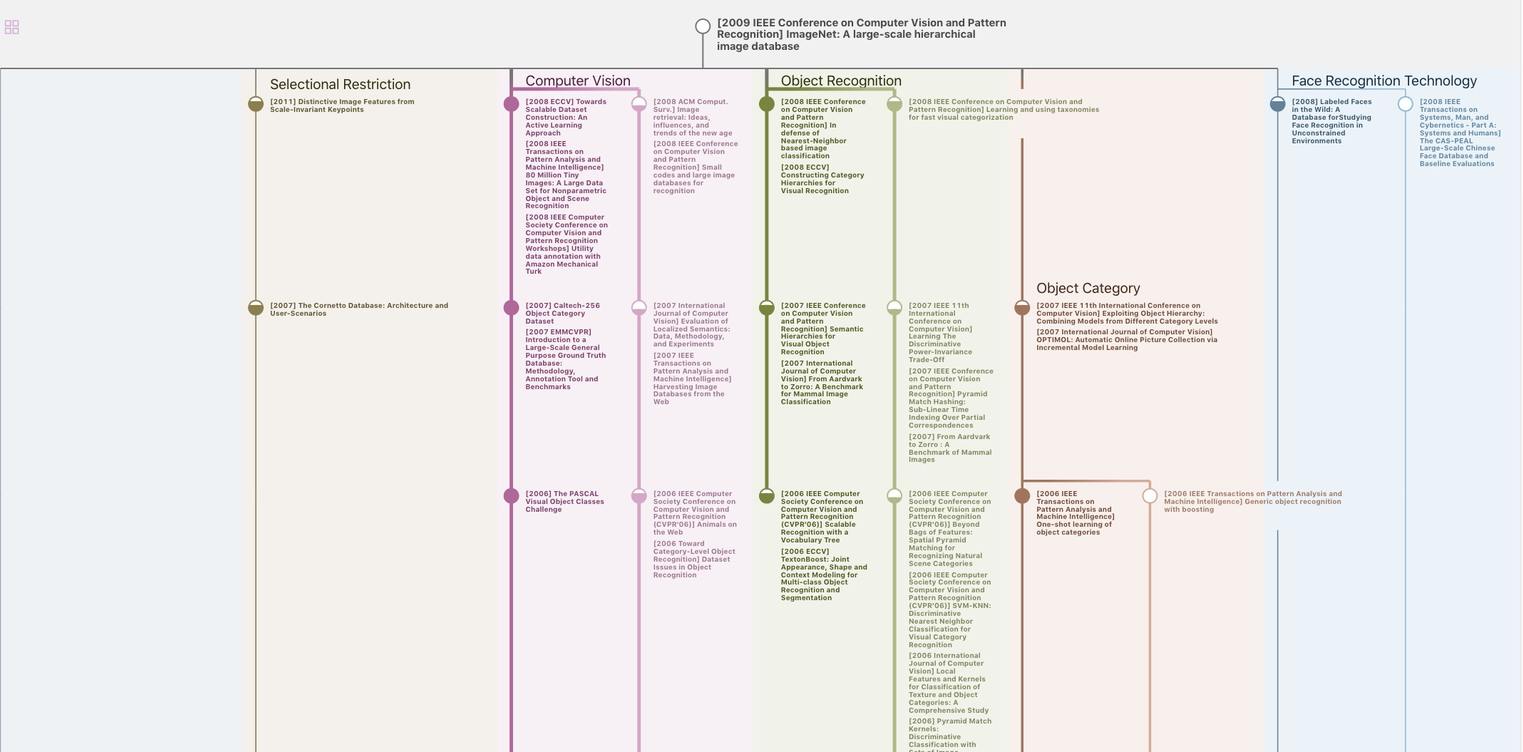
生成溯源树,研究论文发展脉络
Chat Paper
正在生成论文摘要