Collaborative Reinforcement Learning Framework to Model Evolution of Cooperation in Sequential Social Dilemmas
ADVANCES IN KNOWLEDGE DISCOVERY AND DATA MINING, PAKDD 2021, PT I(2021)
摘要
Multi-agent reinforcement learning (MARL) has very high sample complexity leading to slow learning. For repeated social dilemma games e.g. Public Goods Game(PGG), Fruit Gathering Game(FGG), MARL exhibits low sustainability of cooperation due to non-stationarity of the agents and the environment, and the large sample complexity. Motivated by the fact that humans learn not only through their own actions (organic learning) but also by following the actions of other humans (social learning) who also continuously learn about the environment, we address this challenge by augmenting RL based models with a notion of collaboration among agents. In particular, we propose Collaborative-Reinforcement-Learning (CRL), where agents collaborate by observing and following other agent's actions/decisions. The CRL model significantly influences the speed of individual learning, which effects the collective behavior as compared to RL only models and thereby effectively explaining the sustainability of cooperation in repeated PGG settings. We also extend the CRL model for PGGs over different generations where agents die, and new agents are born following a birth-death process. Also, extending the proposed CRL model, we propose Collaborative Deep RL Network(CDQN) for a team based game (FGG) and the experimental results confirm that agents following CDQN learns faster and collects more fruits.
更多查看译文
关键词
MARL, PGG, Collaborative
AI 理解论文
溯源树
样例
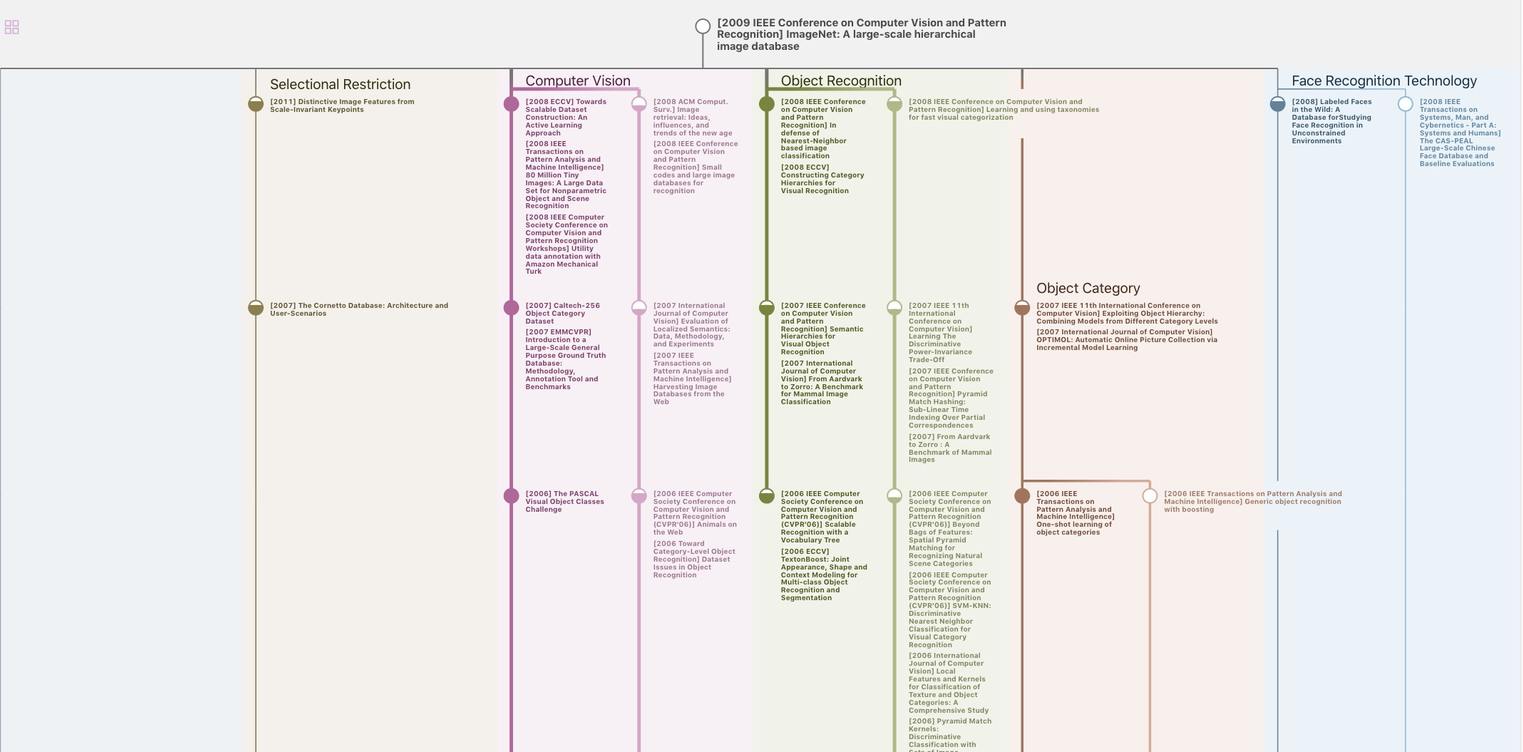
生成溯源树,研究论文发展脉络
Chat Paper
正在生成论文摘要