Semantic adaptation network for unsupervised domain adaptation
Neurocomputing(2021)
摘要
Domain adaptation methods aim at learning transferable model for the unlabeled target domain. Recently, extensive domain adaptation models are proposed to align representations by minimizing distribution distance between different domains or adversarial training manner. However, most existing methods only adapt the different features in feature space and ignore the semantic features that generated by the classifier, which can lead to misclassification of target samples near the source decision boundaries. In this paper, we propose a Semantic Adaptation Network (SAN) for unsupervised domain adaptation. SAN aligns the domain representations at both feature-level and semantic-level. SAN utilizes adversarial training to align different domain representations in the feature space. In the semantic space, SAN obtains the pseudo labels of target samples by nearest source semantic representation centroid, then forces the pseudo labeled target semantic representations close to corresponding source semantic representation centroids. Experiments on ImageCLEF-DA, Office-Home and VisDA datasets validate the effectiveness and superiority of our model.
更多查看译文
关键词
Domain adaptation,Adversarial training,Deep networks,Image classification
AI 理解论文
溯源树
样例
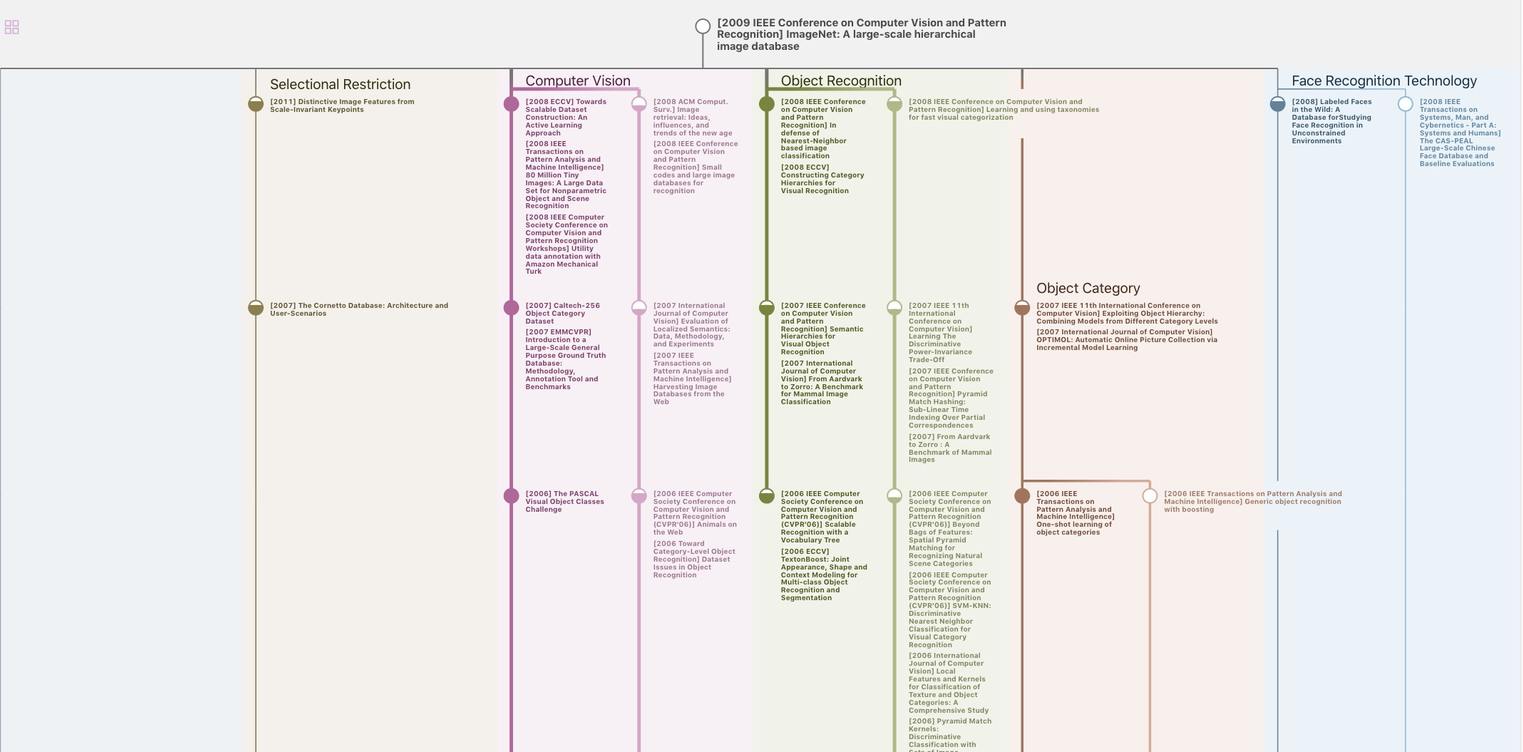
生成溯源树,研究论文发展脉络
Chat Paper
正在生成论文摘要