DAE‐GAN : An autoencoder based adversarial network for Gaussian denoising
Expert Systems(2021)
摘要
Image denoising is one of the most classic problems in computer vision for restoring corrupted images. It has been approached by using various traditional state of the art architectures in convolutional neural network (CNN), which has demonstrated considerably better results than the prior methods. There has been recent advancements in approaching the problem using generative adversarial networks (GAN), which has shown considerable promise. In this paper, we propose a novel denoising adversarial architecture to generate denoised image samples from a noisy distribution. A denoising autoencoder has been employed as the Generator to learn image distributions and generate denoised images while the discriminator penalizes the generated output. We employ an additive loss comprising of root mean square and mean absolute error for the Generator function. The model is trained adversarially followed by extensive experiments. We achieved PSNR and SSIM values comparable to the state-of-the-art for a range of blind and non-blind Gaussian noise.
更多查看译文
关键词
computer vision, convolutional neural networks, deep learning, generating adversarial networks
AI 理解论文
溯源树
样例
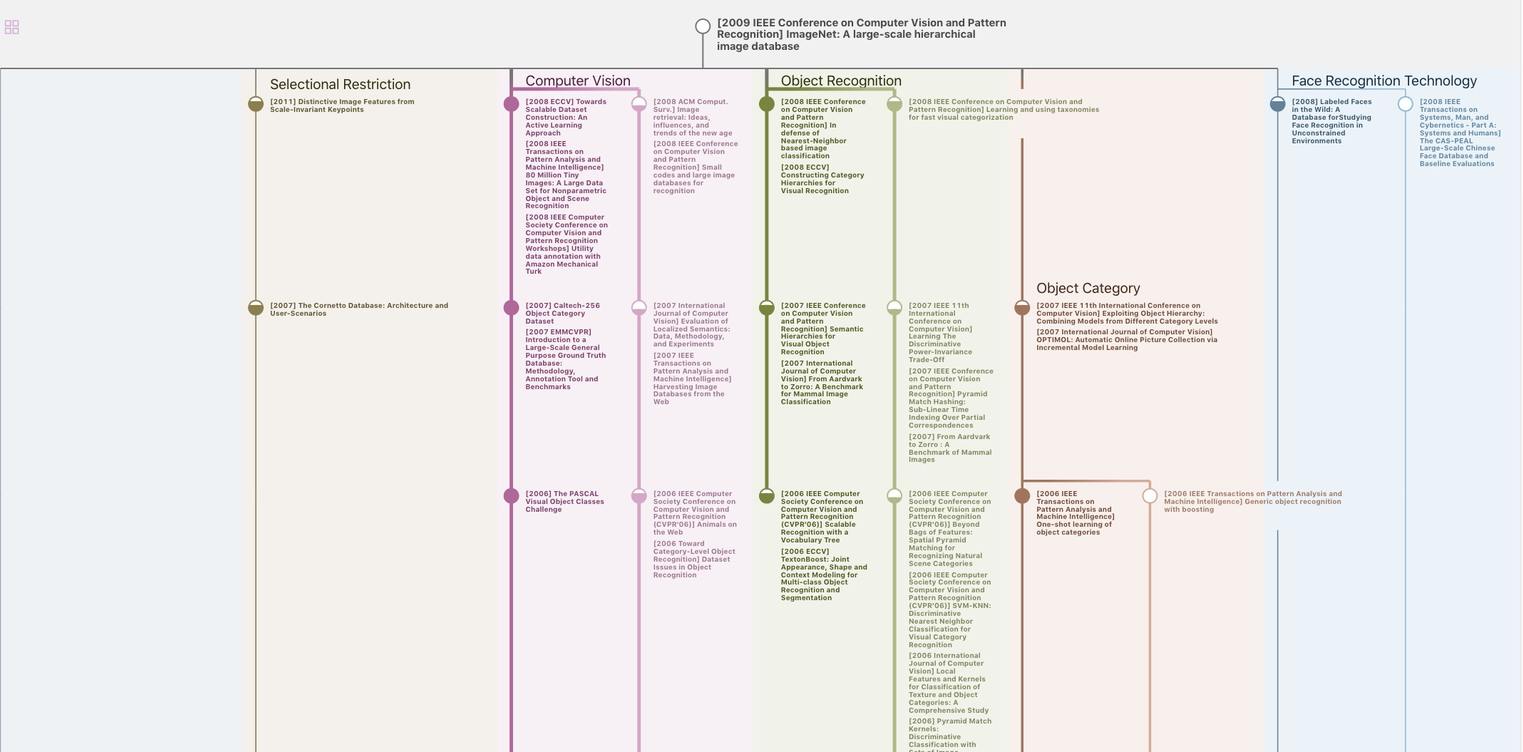
生成溯源树,研究论文发展脉络
Chat Paper
正在生成论文摘要