Modeling Focused-Ultrasound Response for Non-Invasive Treatment Using Machine Learning.
BIOENGINEERING-BASEL(2021)
摘要
The interactions between body tissues and a focused ultrasound beam can be evaluated using various numerical models. Among these, the Rayleigh-Sommerfeld and angular spectrum methods are considered to be the most effective in terms of accuracy. However, they are computationally expensive, which is one of the underlying issues of most computational models. Typically, evaluations using these models require a significant amount of time (hours to days) if realistic scenarios such as tissue inhomogeneity or non-linearity are considered. This study aims to address this issue by developing a rapid estimation model for ultrasound therapy using a machine learning algorithm. Several machine learning models were trained on a very-large dataset (19,227 simulations), and the performance of these models were evaluated with metrics such as Root Mean Squared Error (RMSE), R-squared (R2), Akaike Information Criterion (AIC), and Bayesian Information Criterion (BIC). The resulted random forest provides superior accuracy with an R2 value of 0.997, an RMSE of 0.0123, an AIC of -82.56, and a BIC of -81.65 on an external test dataset. The results indicate the efficacy of the random forest-based model for the focused ultrasound response, and practical adoption of this approach will improve the therapeutic planning process by minimizing simulation time.
更多查看译文
关键词
machine learning, numerical model, random forest, focused ultrasound, Rayleigh-Sommerfeld, angular spectrum
AI 理解论文
溯源树
样例
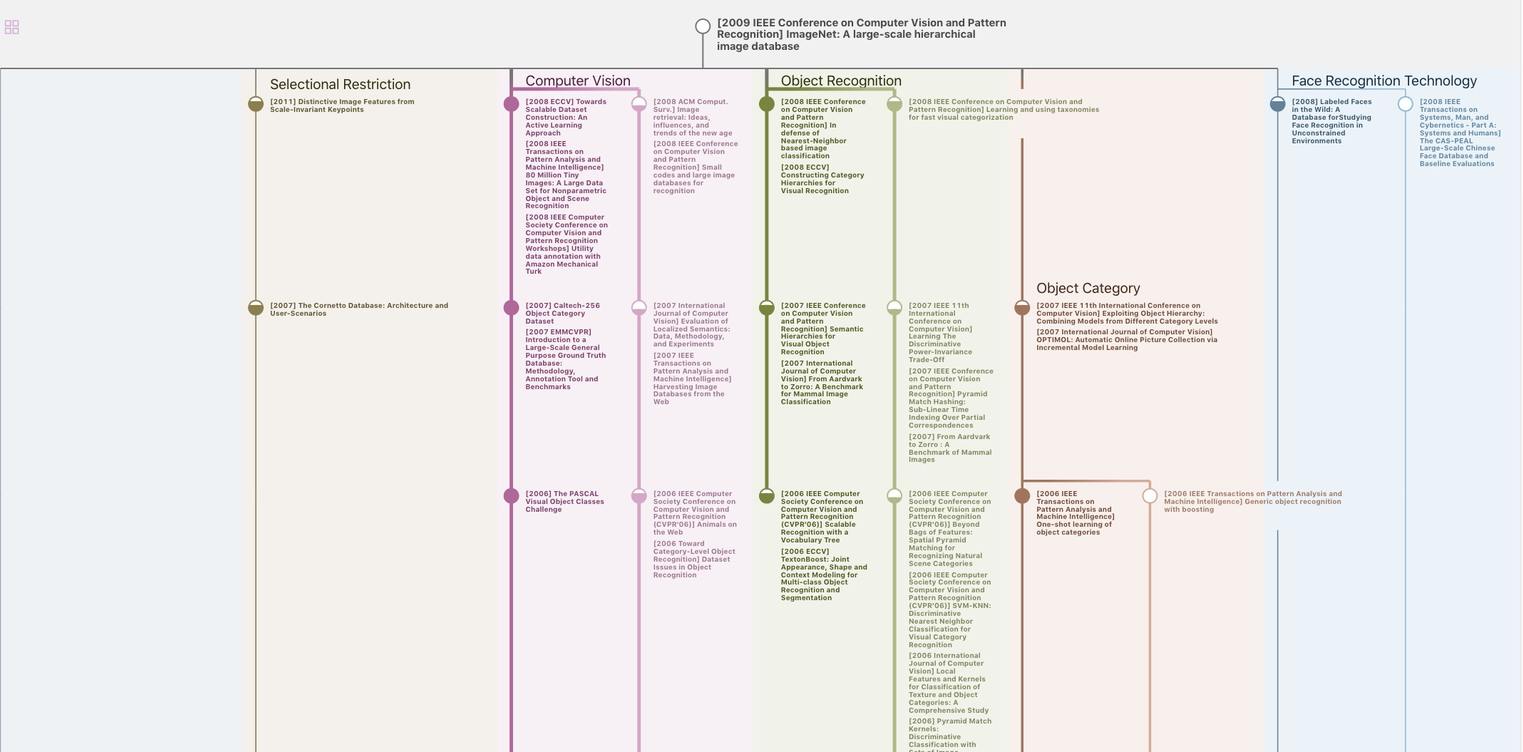
生成溯源树,研究论文发展脉络
Chat Paper
正在生成论文摘要