Orienting (Hyper)graphs Under Explorable Stochastic Uncertainty.
ESA(2021)
摘要
Given a hypergraph with uncertain node weights following known probability distributions, we study the problem of querying as few nodes as possible until the identity of a node with minimum weight can be determined for each hyperedge. Querying a node has a cost and reveals the precise weight of the node, drawn from the given probability distribution. Using competitive analysis, we compare the expected query cost of an algorithm with the expected cost of an optimal query set for the given instance. For the general case, we give a polynomial-time $f(\alpha)$-competitive algorithm, where $f(\alpha)\in [1.618+\epsilon,2]$ depends on the approximation ratio $\alpha$ for an underlying vertex cover problem. We also show that no algorithm using a similar approach can be better than $1.5$-competitive. Furthermore, we give polynomial-time $4/3$-competitive algorithms for bipartite graphs with arbitrary query costs and for hypergraphs with a single hyperedge and uniform query costs, with matching lower bounds.
更多查看译文
关键词
hypergraphs,uncertainty
AI 理解论文
溯源树
样例
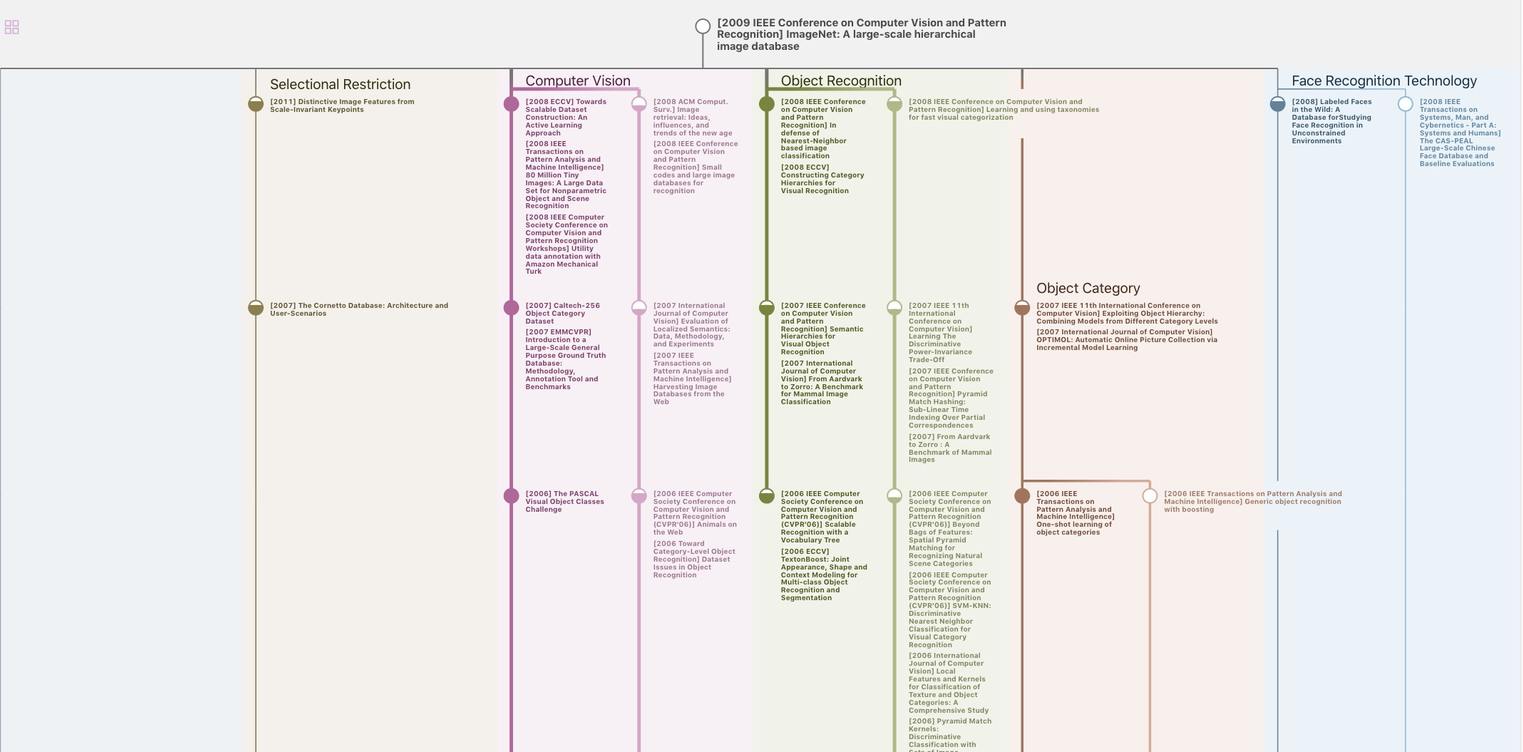
生成溯源树,研究论文发展脉络
Chat Paper
正在生成论文摘要