Neural Networks as Geometric Chaotic Maps
IEEE Transactions on Neural Networks and Learning Systems(2023)
摘要
The use of artificial neural networks (NNs) as models of chaotic dynamics has been rapidly expanding. Still, a theoretical understanding of how NNs learn chaos is lacking. Here, we employ a geometric perspective to show that NNs can efficiently model chaotic dynamics by becoming structurally chaotic themselves. We first confirm NN’s efficiency in emulating chaos by showing that a parsimonious NN trained only on few data points can reconstruct strange attractors, extrapolate outside training data boundaries, and accurately predict local divergence rates. We then posit that the trained network’s map comprises sequential geometric stretching, rotation, and compression operations. These geometric operations indicate topological mixing and chaos, explaining why NNs are naturally suitable to emulate chaotic dynamics.
更多查看译文
关键词
Chaos,neural networks (NNs),nonlinear dynamical systems,topological mixing
AI 理解论文
溯源树
样例
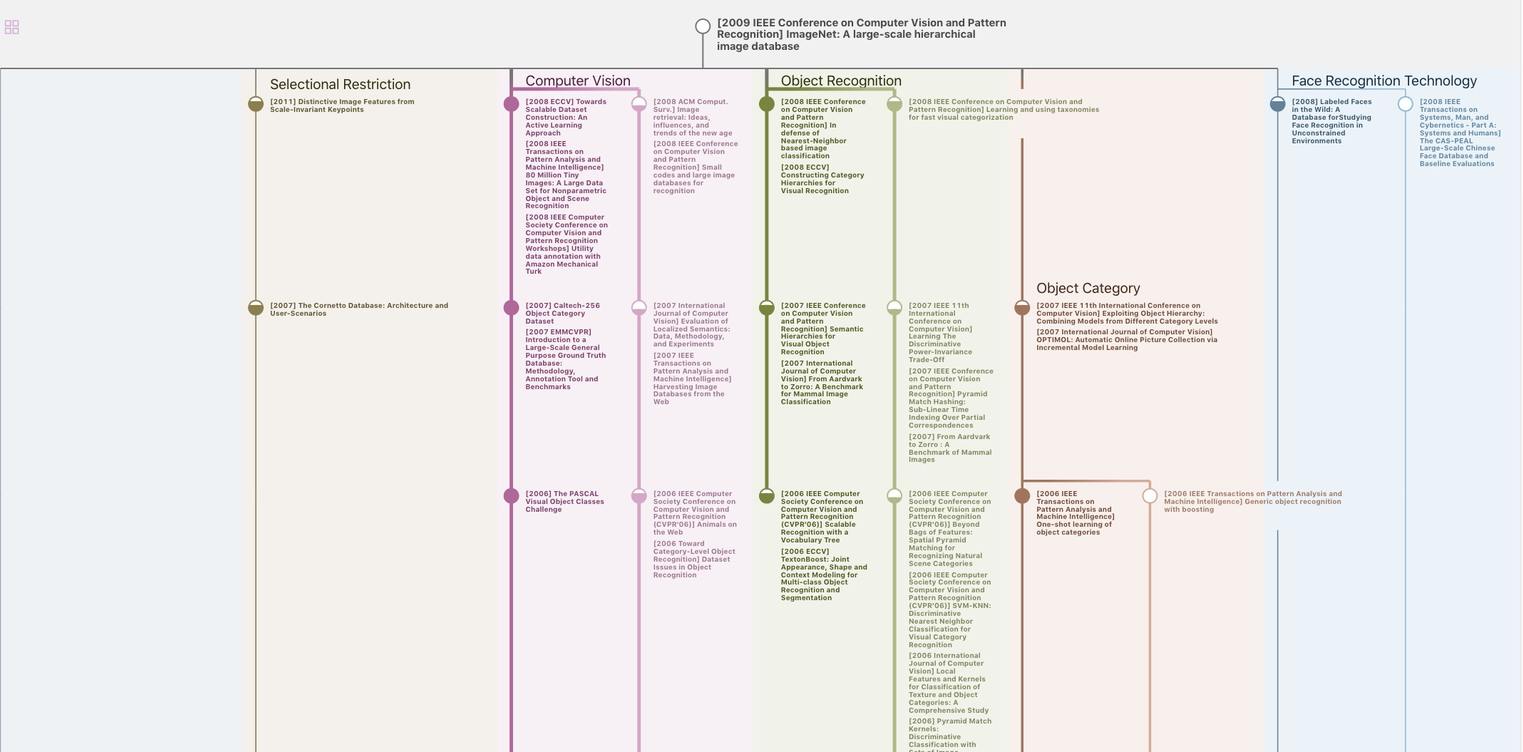
生成溯源树,研究论文发展脉络
Chat Paper
正在生成论文摘要