Conformal Prediction With Orange
JOURNAL OF STATISTICAL SOFTWARE(2021)
摘要
Conformal predictors estimate the reliability of outcomes made by supervised machine learning models. Instead of a point value, conformal prediction defines an outcome region that meets a user-specified reliability threshold. Provided that the data are independently and identically distributed, the user can control the level of the prediction errors and adjust it following the requirements of a given application. The quality of conformal predictions often depends on the choice of nonconformity estimate for a given machine learning method. To promote the selection of a successful approach, we have developed Orange3-Conformal, a Python library that provides a range of conformal prediction methods for classification and regression. The library also implements several nonconformity scores. It has a modular design and can be extended to add new conformal prediction methods and nonconformities.
更多查看译文
关键词
conformal prediction, nonconformity, machine learning, Python, Orange
AI 理解论文
溯源树
样例
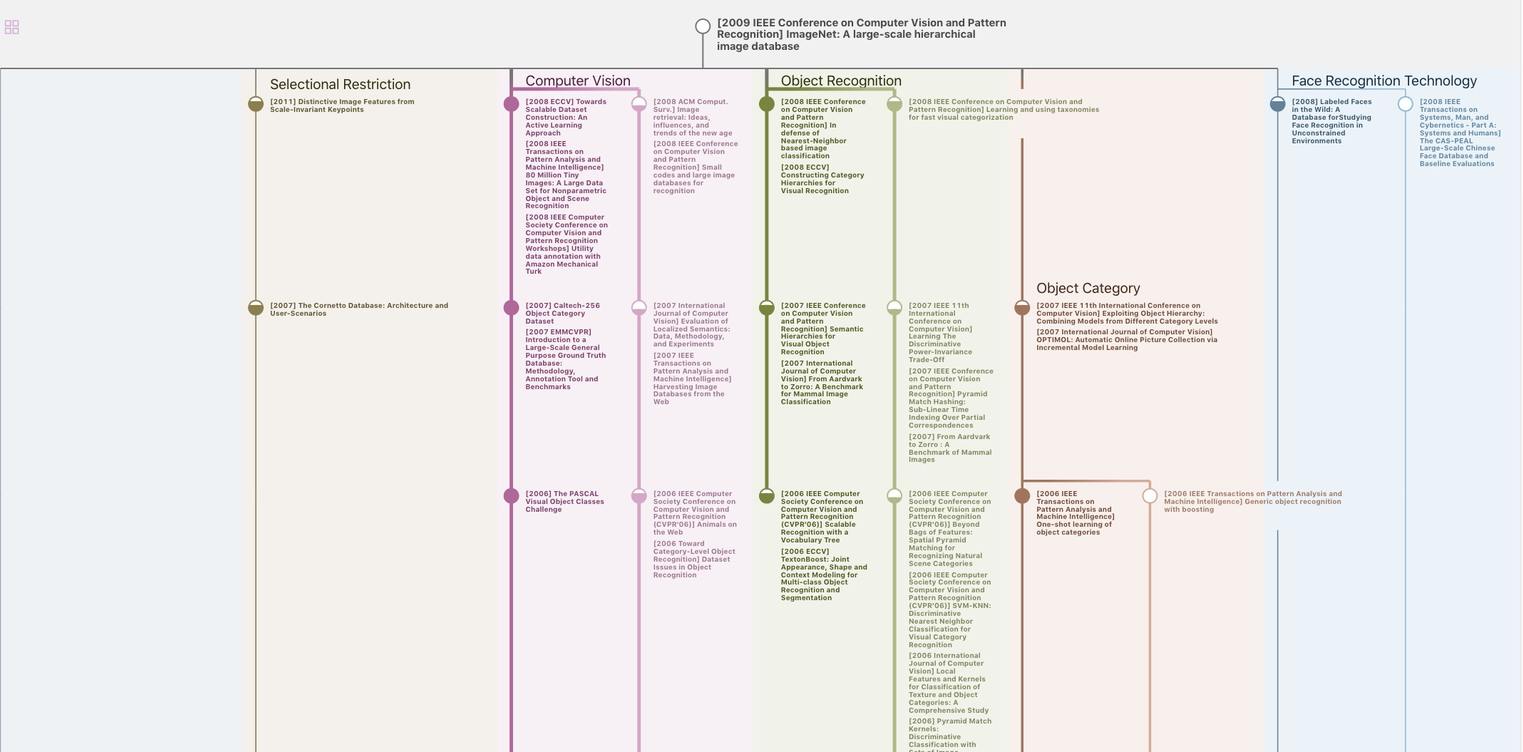
生成溯源树,研究论文发展脉络
Chat Paper
正在生成论文摘要