Open-Set Representation Learning Through Combinatorial Embedding
CVPR 2023(2023)
摘要
Visual recognition tasks are often limited to dealing with a small subset of classes simply because the labels for the remaining classes are unavailable. We are interested in identifying novel concepts in a dataset through representation learning based on both labeled and unlabeled examples, and extending the horizon of recognition to both known and novel classes. To address this challenging task, we propose a combinatorial learning approach, which naturally clusters the examples in unseen classes using the compositional knowledge given by multiple supervised meta-classifiers on heterogeneous label spaces. The representations given by the combinatorial embedding are made more robust by unsupervised pairwise relation learning. The proposed algorithm discovers novel concepts via a joint optimization for enhancing the discrimitiveness of unseen classes as well as learning the representations of known classes generalizable to novel ones. Our extensive experiments demonstrate remarkable performance gains by the proposed approach on public datasets for image retrieval and image categorization with novel class discovery.
更多查看译文
关键词
combinatorial embedding,representation,learning,open-set
AI 理解论文
溯源树
样例
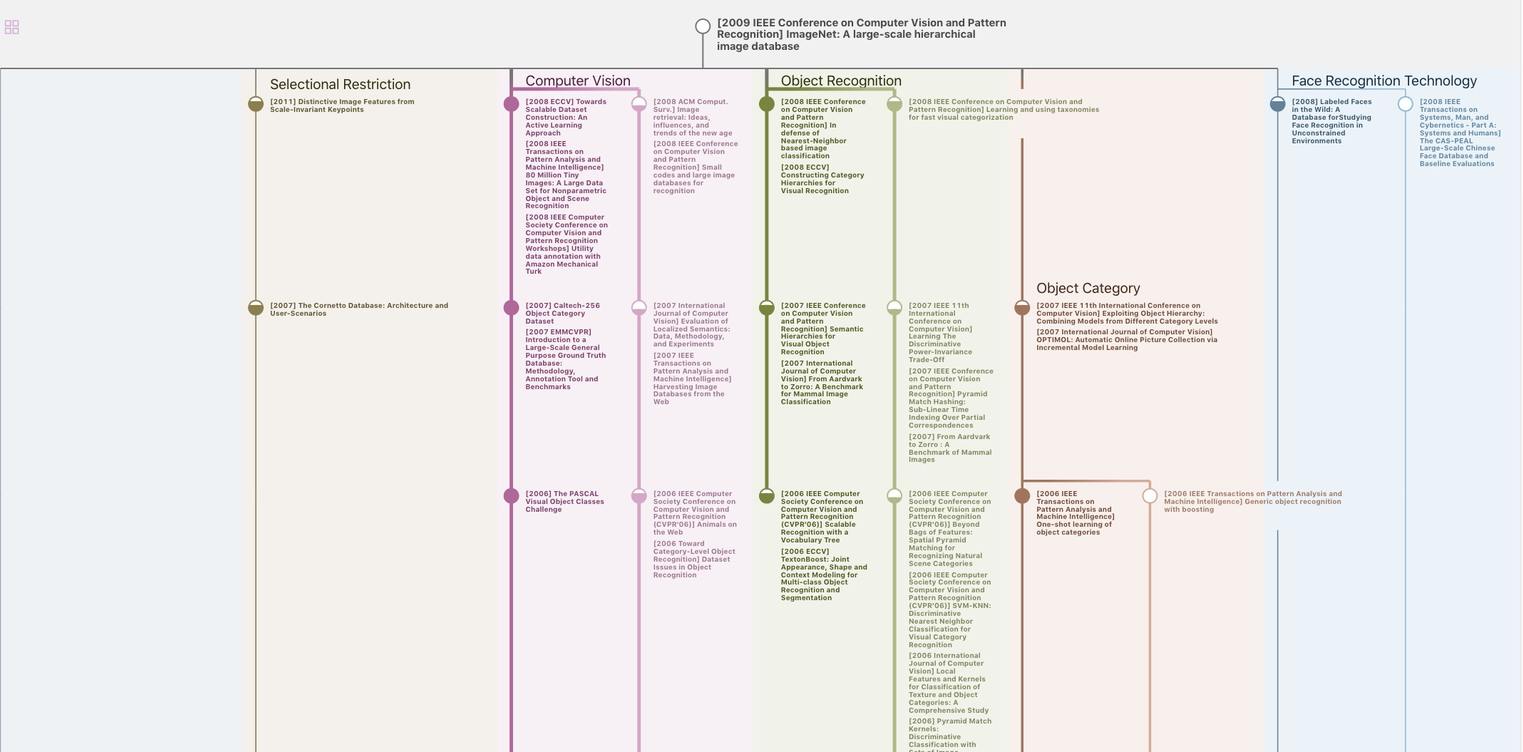
生成溯源树,研究论文发展脉络
Chat Paper
正在生成论文摘要