Dynamic Bayesian Network Learning to Infer Sparse Models From Time Series Gene Expression Data
IEEE/ACM Transactions on Computational Biology and Bioinformatics(2022)
摘要
One of the key challenges in systems biology is to derive gene regulatory networks (GRNs) from complex high-dimensional sparse data. Bayesian networks (BNs) and dynamic Bayesian networks (DBNs) have been widely applied to infer GRNs from gene expression data. GRNs are typically sparse but traditional approaches of BN structure learning to elucidate GRNs often produce many spurious (false positive) edges. We present two new BN scoring functions, which are extensions to the Bayesian Information Criterion (BIC) score, with additional penalty terms and use them in conjunction with DBN structure search methods to find a graph structure that maximises the proposed scores. Our BN scoring functions offer better solutions for inferring networks with fewer spurious edges compared to the BIC score. The proposed methods are evaluated extensively on auto regressive and DREAM4 benchmarks. We found that they significantly improve the precision of the learned graphs, relative to the BIC score. The proposed methods are also evaluated on three real time series gene expression datasets. The results demonstrate that our algorithms are able to learn sparse graphs from high-dimensional time series data. The implementation of these algorithms is open source and is available in form of an R package on GitHub at
https://github.com/HamdaBinteAjmal/DBN4GRN
, along with the documentation and tutorials.
更多查看译文
关键词
Computational biology,bioinformatics,Bayesian networks,gene regulatory networks,gene expression
AI 理解论文
溯源树
样例
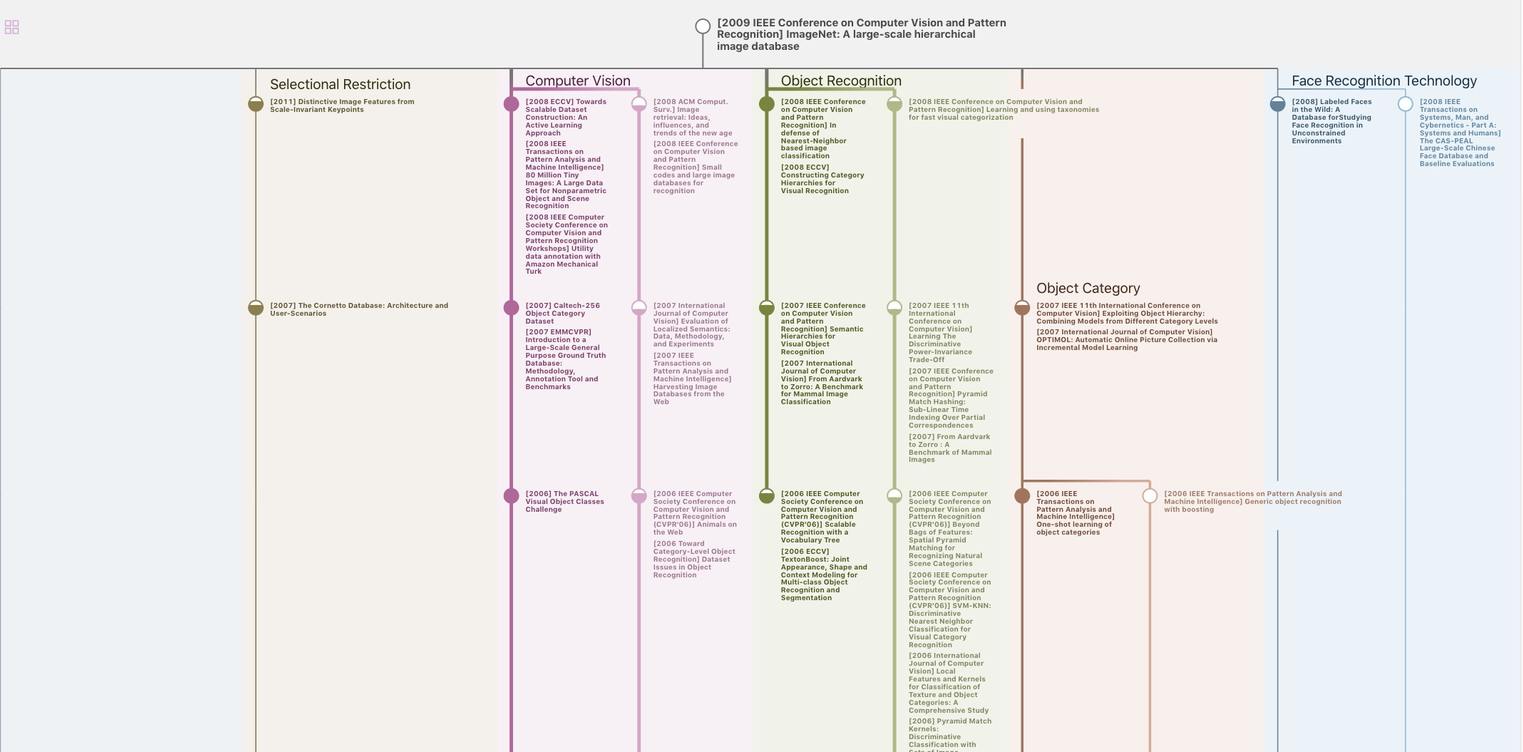
生成溯源树,研究论文发展脉络
Chat Paper
正在生成论文摘要