An Efficient Deep Distribution Network for Bid Shading in First-Price Auctions
Knowledge Discovery and Data Mining(2021)
摘要
ABSTRACTSince 2019, most ad exchanges and sell-side platforms (SSPs), in the online advertising industry, shifted from second to first price auctions. Due to the fundamental difference between these auctions, demand-side platforms (DSPs) have had to update their bidding strategies to avoid bidding unnecessarily high and hence overpaying. Bid shading was proposed to adjust the bid price intended for second-price auctions, in order to balance cost and winning probability in a first-price auction setup. In this study, we introduce a novel deep distribution network for optimal bidding in both open (non-censored) and closed (censored) online first-price auctions. Offline and online A/B testing results show that our algorithm outperforms previous state-of-art algorithms in terms of both surplus and effective cost per action (eCPX) metrics. Furthermore, the algorithm is optimized in run-time and has been deployed into VerizonMedia DSP as production algorithm, serving hundreds of billions of bid requests per day. Online A/B test shows that advertiser's ROI are improved by +2.4%, +2.4%, and +8.6% for impression based (CPM), click based (CPC), and conversion based (CPA) campaigns respectively.
更多查看译文
关键词
Online Auction, Real-Time Bidding, Display Advertising, Bid Shading, Distribution Learning
AI 理解论文
溯源树
样例
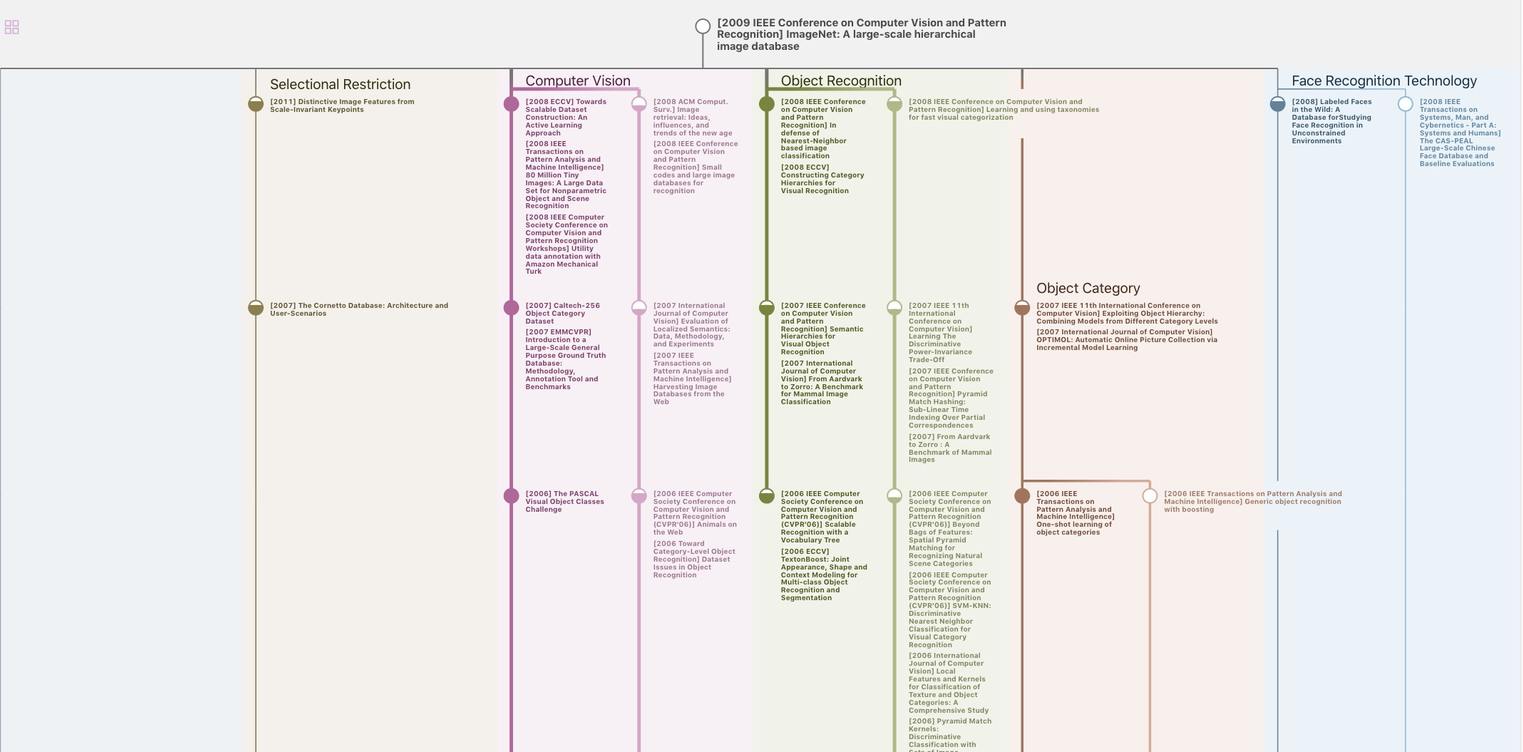
生成溯源树,研究论文发展脉络
Chat Paper
正在生成论文摘要