Prediction of harmful algal blooms in large water bodies using the combined EFDC and LSTM models
Journal of Environmental Management(2021)
摘要
Harmful algal blooms (HABs) is a worldwide water environmental problem. HABs usually happens in short time and is difficult to be controlled. Early warning of HABs using data-driven models is prospective in making time for taking precaution against HABs. High-frequency water quality monitoring data are necessary to improve the reliability of the model, but it is expensive. This research used environmental fluid dynamics code (EFDC) to extend one-point data obtained by only one instrument to the whole 249 ha water area instead of multi-instruments monitoring, followed by Long short-term memory (LSTM) to predict the HABs in the whole water body. Correlation analysis and principal component analysis were used to reduce the data dimension and improve model accuracy. Finally, the LSTM model was calibrated to predict chlorophyll-a (Chl-a) for the next 1 to 3 time steps. The Nash-Sutcliffe efficiency coefficient (NSE) and mean absolute percentage error (MAPE) of EFDC-LSTM were 0.797–0.991 and 2.74–13.16%, respectively, suggesting the promising utilization of this model in early warning systems for HABs. EFDC-LSTM achieves high-precision HABs forecasting in a cost-effective manner, providing a reliable way to detect HABs in advance.
更多查看译文
关键词
Bloom,Chlorophyll-a,Deep learning,Model coupling,Prediction
AI 理解论文
溯源树
样例
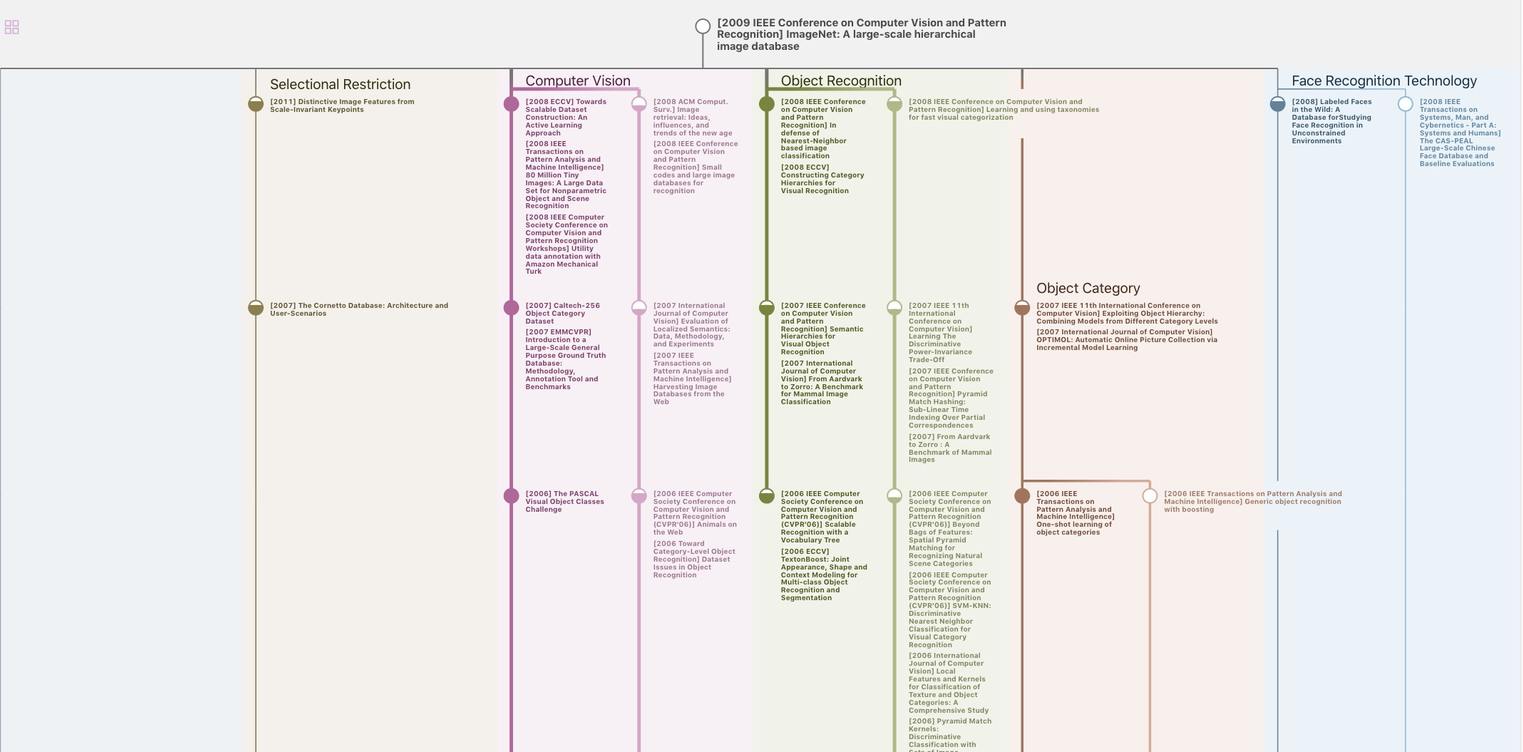
生成溯源树,研究论文发展脉络
Chat Paper
正在生成论文摘要