Cut to the Trace! Process-Aware Partitioning of Long-Running Cases in Customer Journey Logs
ADVANCED INFORMATION SYSTEMS ENGINEERING (CAISE 2021)(2021)
摘要
Customer journeys are recordings of customer interactions with organizational information systems. These interactions are often recorded into so-called customer journey logs. Customer journeys often correspond to long-running and flexible traces that may temper the use of process analytics techniques such as process mining. A common method to make long-running traces suitable for process mining algorithms is to partition them at the largest temporal differences between consecutive events. However, these techniques ignores process context that journeys are often influenced by. In this work, we propose a probabilistic framework that generalizes previous techniques and introduces two novel process-aware partitioning approaches. The first method is inspired by the directly-follows relation, a predominant abstraction in process discovery. The second approach leverages LSTMs, a type of Neural Networks that learn long-term dependencies in sequences. We show that both approaches outperform existing time partitioning methods on both synthetic and real-world customer journey data.
更多查看译文
关键词
Customer journey analysis, Process mining, Trace partitioning
AI 理解论文
溯源树
样例
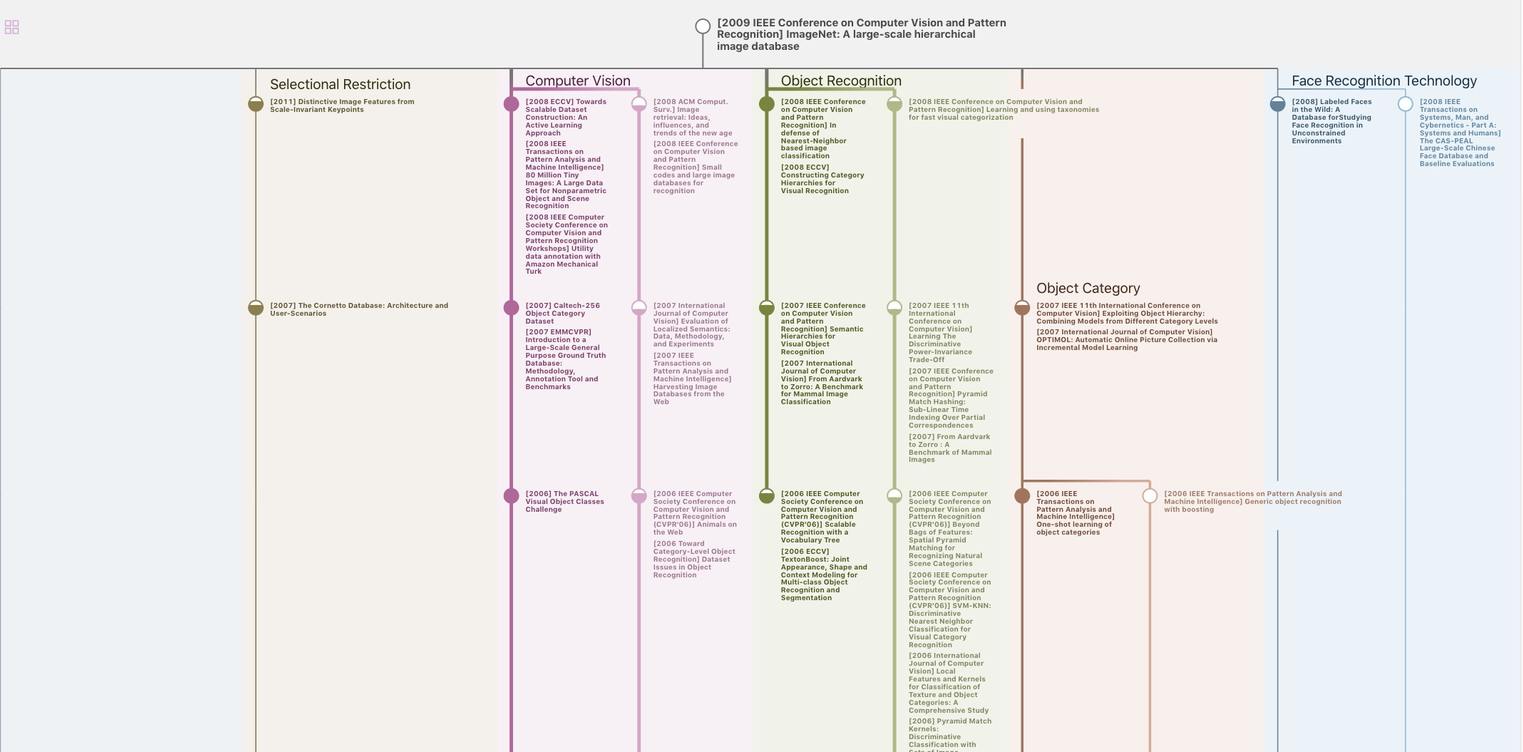
生成溯源树,研究论文发展脉络
Chat Paper
正在生成论文摘要