DP-SGD vs PATE: Which Has Less Disparate Impact on Model Accuracy?
arxiv(2021)
摘要
Recent advances in differentially private deep learning have demonstrated that application of differential privacy, specifically the DP-SGD algorithm, has a disparate impact on different sub-groups in the population, which leads to a significantly high drop-in model utility for sub-populations that are under-represented (minorities), compared to well-represented ones. In this work, we aim to compare PATE, another mechanism for training deep learning models using differential privacy, with DP-SGD in terms of fairness. We show that PATE does have a disparate impact too, however, it is much less severe than DP-SGD. We draw insights from this observation on what might be promising directions in achieving better fairness-privacy trade-offs.
更多查看译文
关键词
model accuracy,pate,dp-sgd
AI 理解论文
溯源树
样例
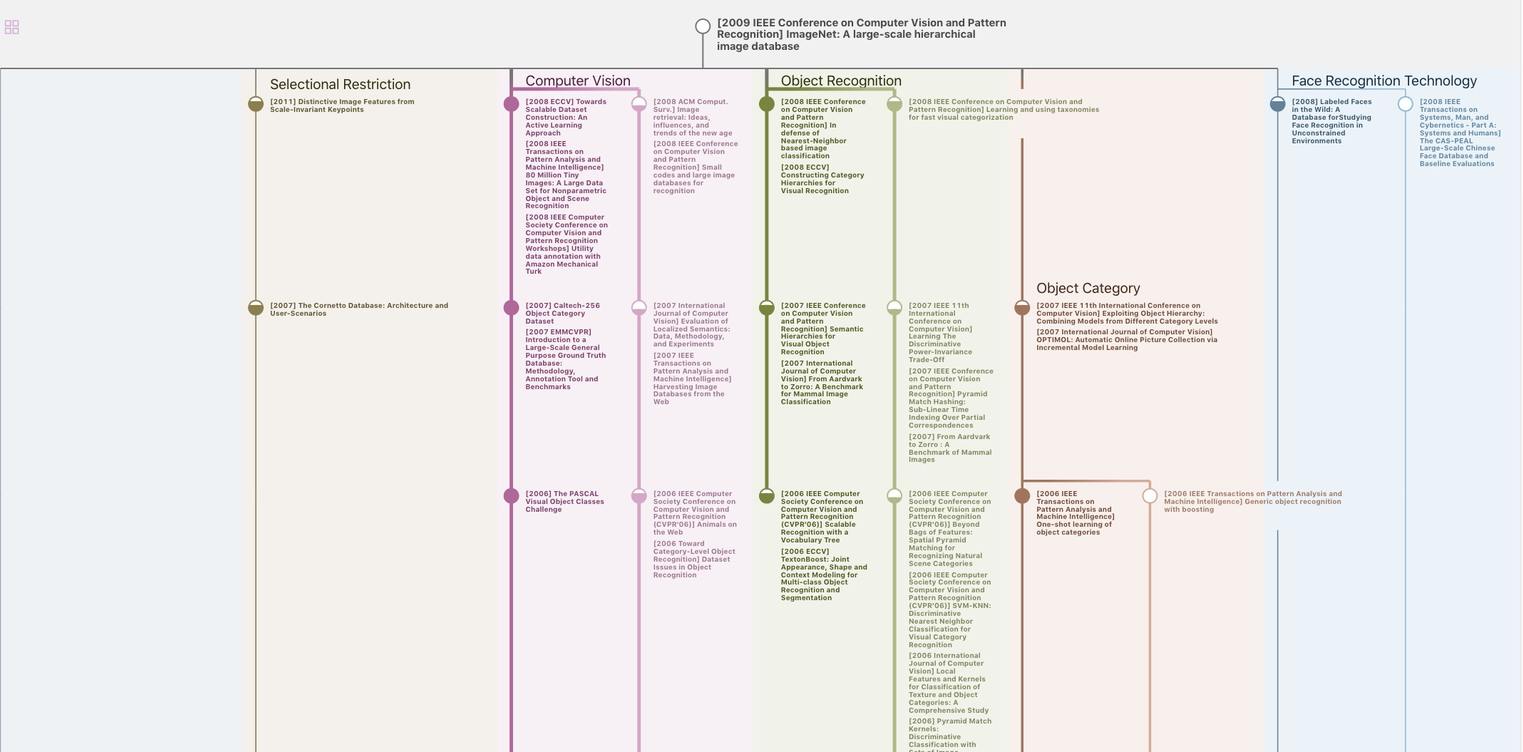
生成溯源树,研究论文发展脉络
Chat Paper
正在生成论文摘要