FeatTS: Feature-based Time Series Clustering
International Conference on Management of Data(2021)
摘要
ABSTRACTClustering time series is a recurrent problem in real-life applications involving data science and data analytics pipelines. Existing time series clustering algorithms are ineffective for feature-rich real-world time series since they only compare the time series based on raw data or use a fixed set of features for determining the similarity. In this paper, we showcase FeatTS, a feature-based semi-supervised clustering framework addressing the above issues for variable-length and heterogeneous time series. Specifically, FeatTS leverages a graph encoding of the time series that is obtained by considering a high number of significant extracted features. It then employs community detection and builds upon a Co-Occurrence matrix in order to unify all the best clustering results. We let the user explore the various steps of FeatTS by visualizing the initial data, its graph encoding and its division into communities along with the obtained clusters. We show how the user can interact with the process for the choice of the features and for varying the percentage of input labels and the various parameters. In view of its characteristics, FeatTS outperforms the state of the art clustering methods and is the first to be able to digest domain-specific time series such as healthcare time series, while still being robust and scalable.
更多查看译文
关键词
Semi-supervised Clustering, Community Detection, Features Selection, Clustering for Data Science
AI 理解论文
溯源树
样例
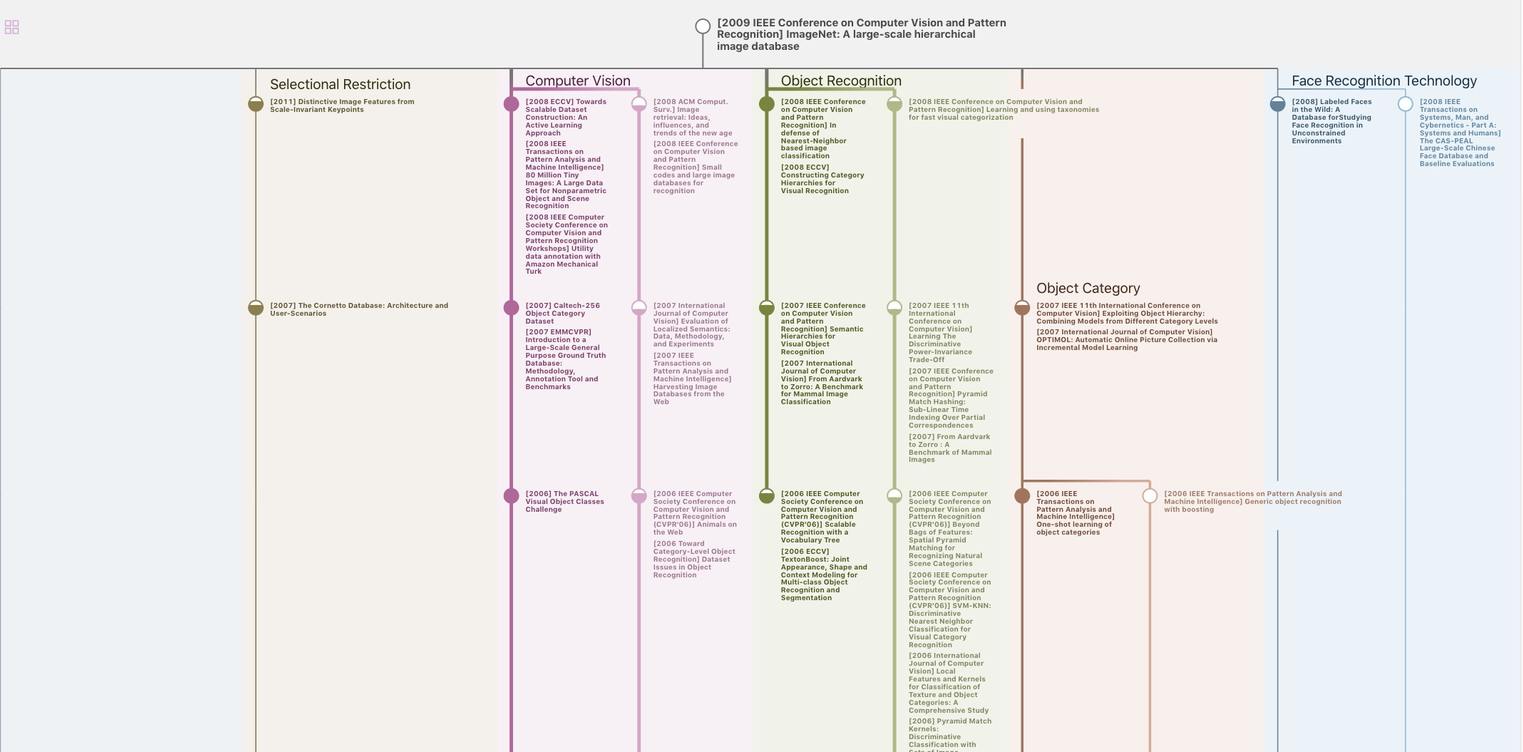
生成溯源树,研究论文发展脉络
Chat Paper
正在生成论文摘要