Grouped Learning: Group-By Model Selection Workloads
International Conference on Management of Data(2021)
摘要
ABSTRACTMachine Learning (ML) is gaining popularity in many applications. Increasingly, companies prefer more targeted models for different subgroups of the population like locations, which helps improve accuracy. This practice is comparable to Group-By aggregation in SQL; we call it learning over groups. A smaller group means the data distribution is more straightforward than the whole population. So, a group-level model may offer more accuracy in many cases. Non-technical business needs, such as privacy and regulatory compliance, may also necessitate group-level models. For instance, online advertising platforms would need to build disaggregated partner-specific ML models, where all partner groups' training data are aggregated together in one data pipeline.
更多查看译文
AI 理解论文
溯源树
样例
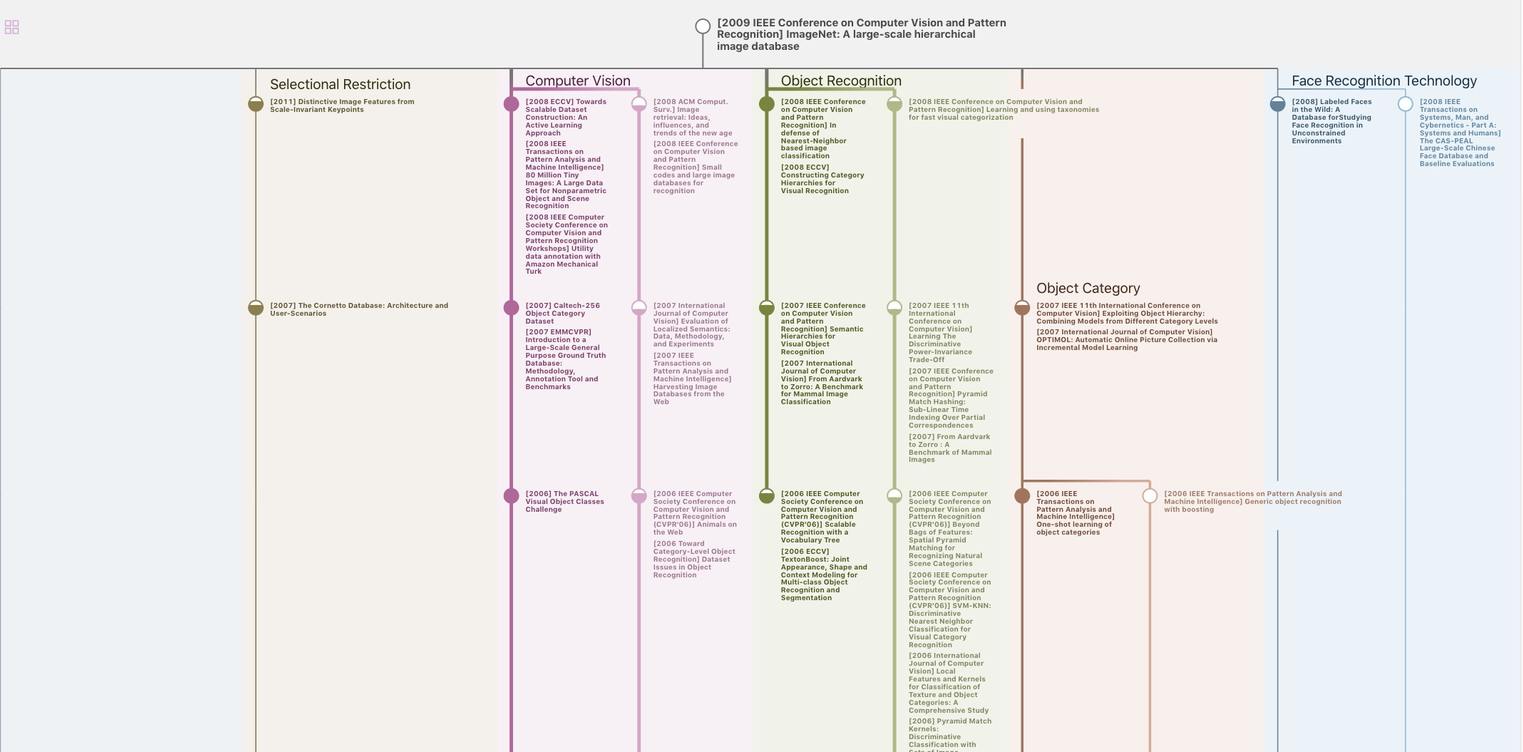
生成溯源树,研究论文发展脉络
Chat Paper
正在生成论文摘要